Reconstructing credit networks
Using ideas from statistical physics to reconstruct the average properties of financial networks from partial sets of information.
When piecing together the fractures and weaknesses that might have led to a system’s failure, the first obstacle is in obtaining all of the data. In the case of financial networks, a full set of data is rarely available due to privacy and non-disclosure issues. This means that statistical methods must be used in order to reconstruct the networks from partial data.
This project seeks new and innovative solutions to this problem, by focusing on the topological structure of financial networks. Whilst it may not be possible to fully reconstruct a financial network and all of its connections, reconstructing the topological features that describe how distress propagates through the network provides the key information in the most efficient way. It becomes possible to understand the higher order behaviour of the network without mapping out each node and link one by one.
Novel approaches lead to surprising results, and indeed structures that were thought to strengthen financial networks are found to be some of the most vulnerable to failure. Identifying these characteristics will help to assess the structure of current financial networks and mitigate the likelihood of the cascading failures that caused recent financial disasters.
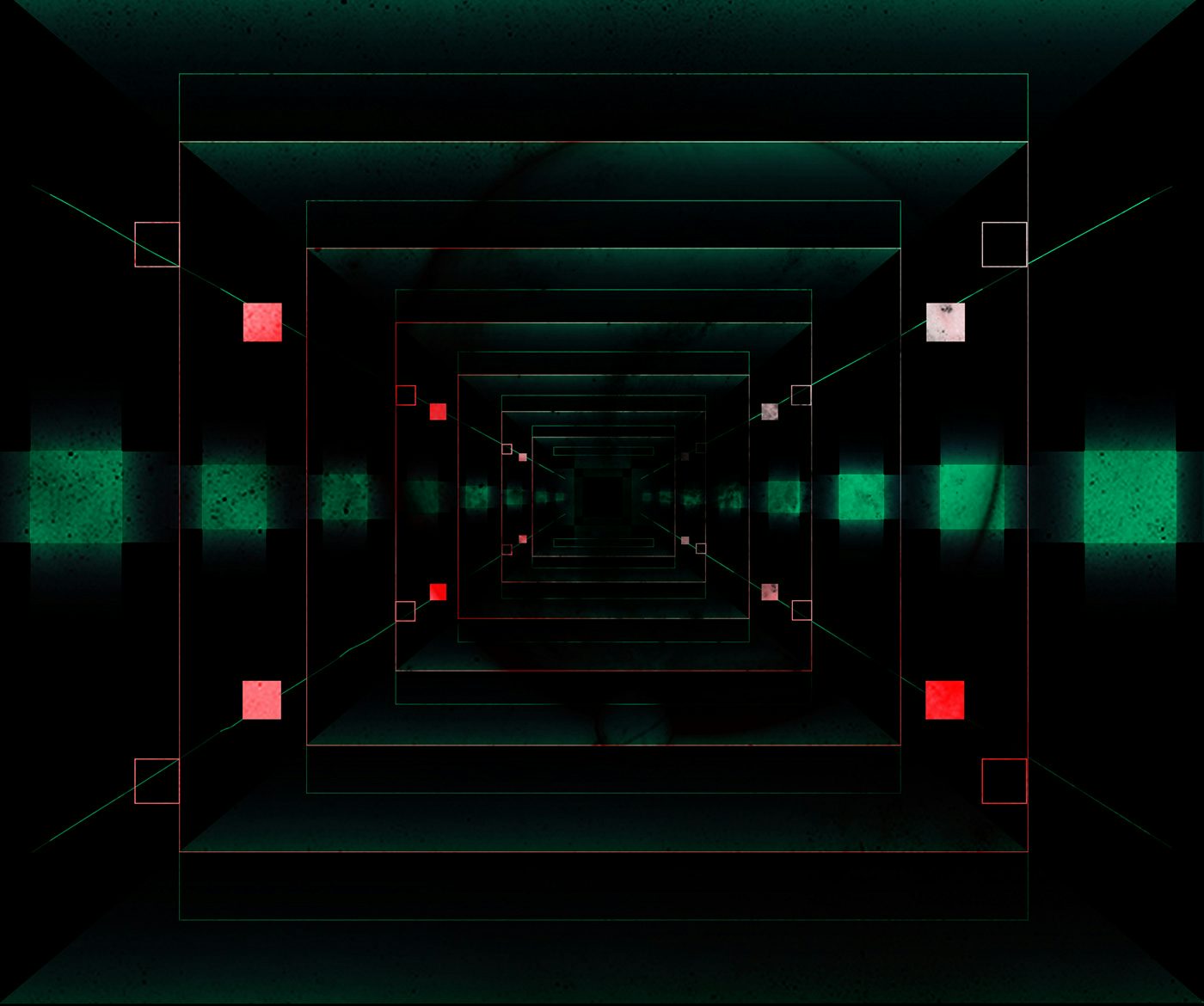
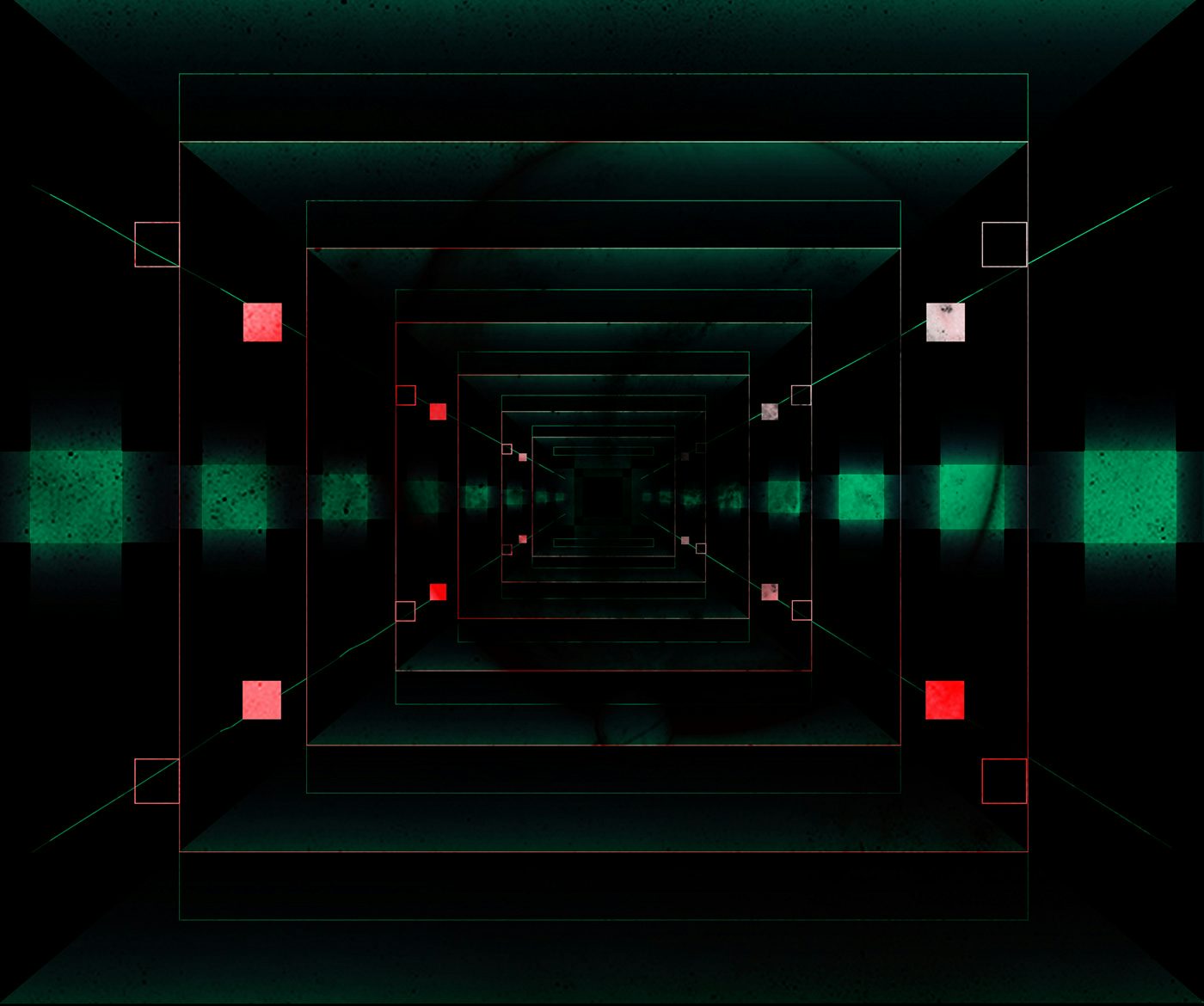
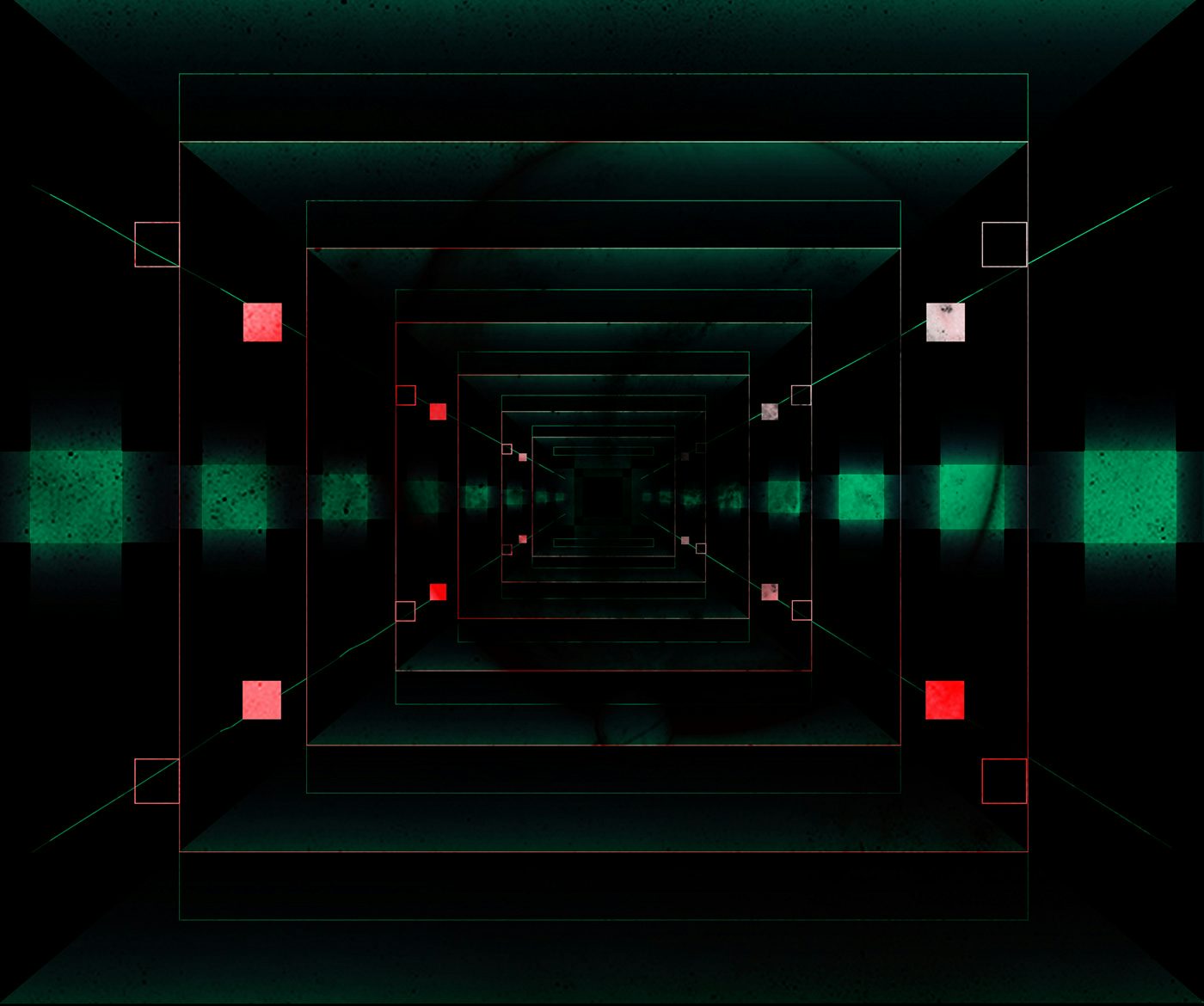
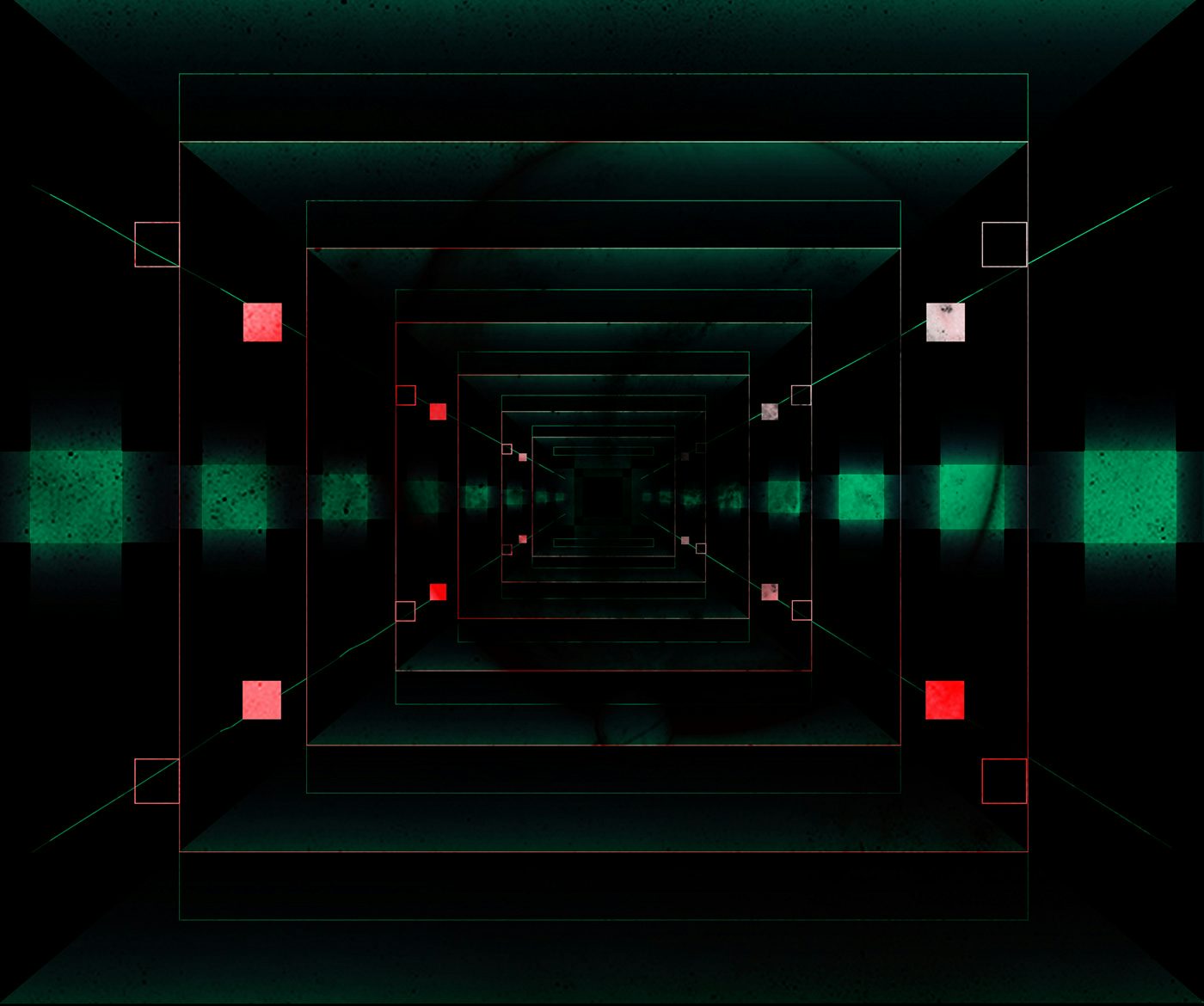
LCP
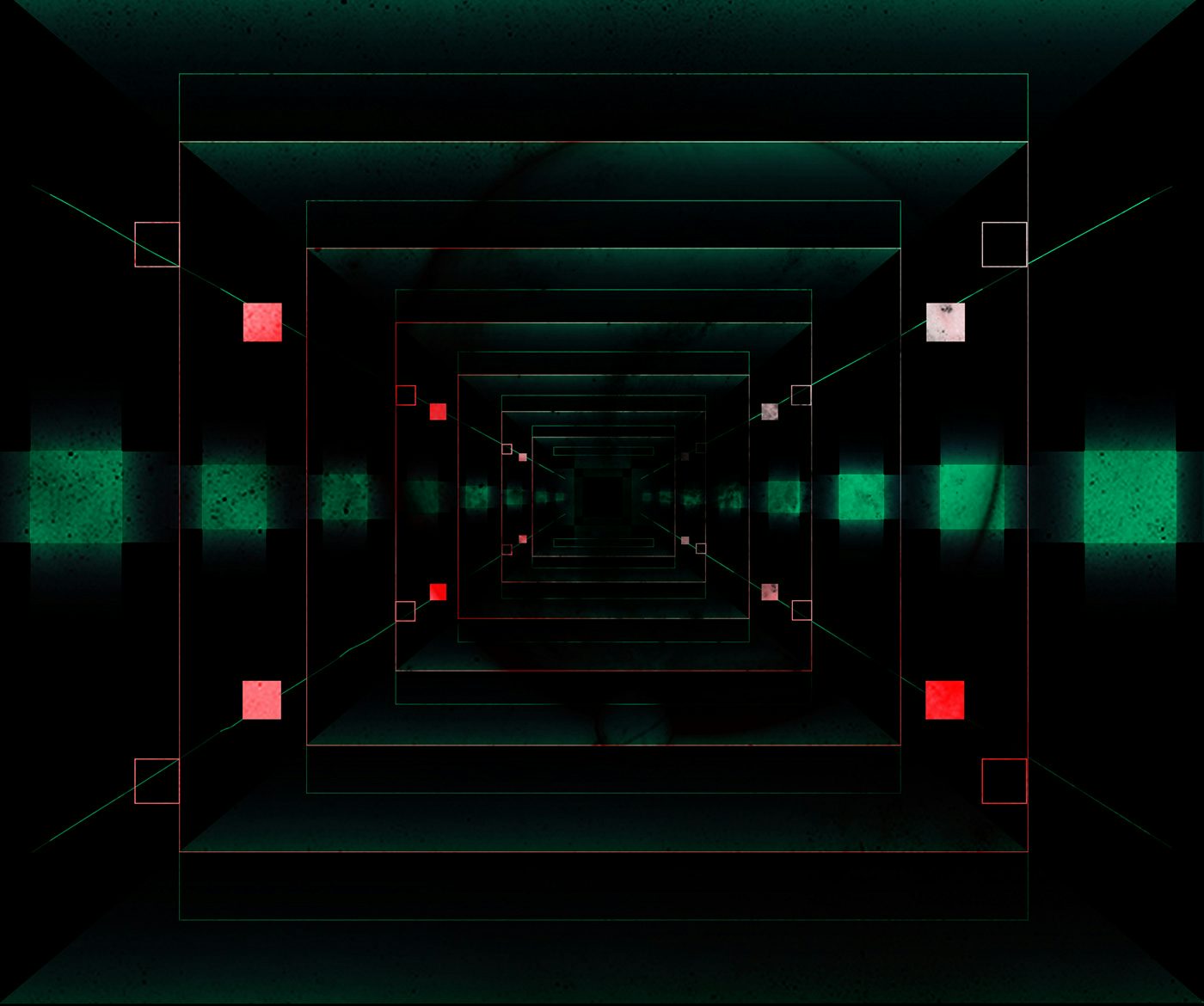
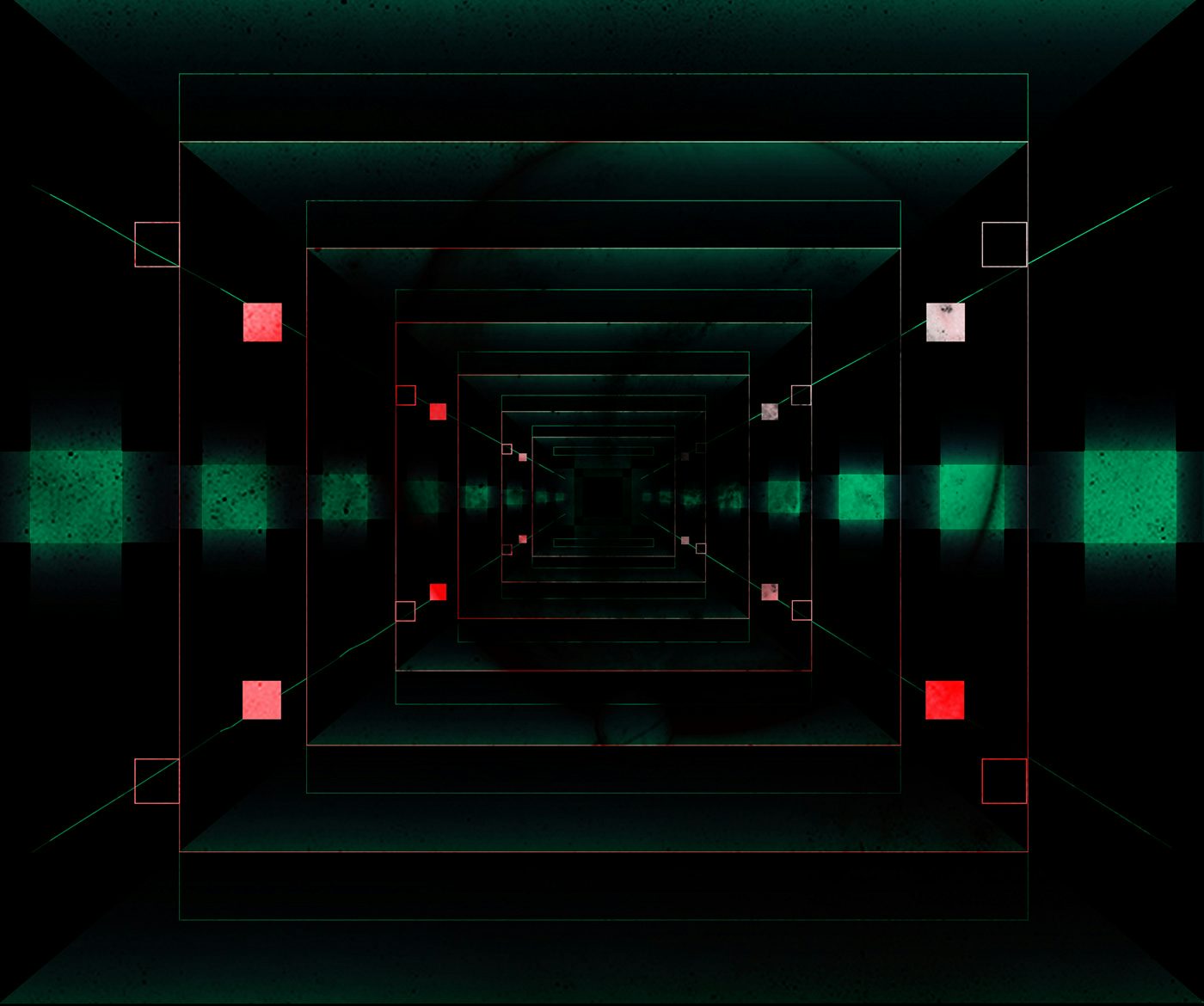
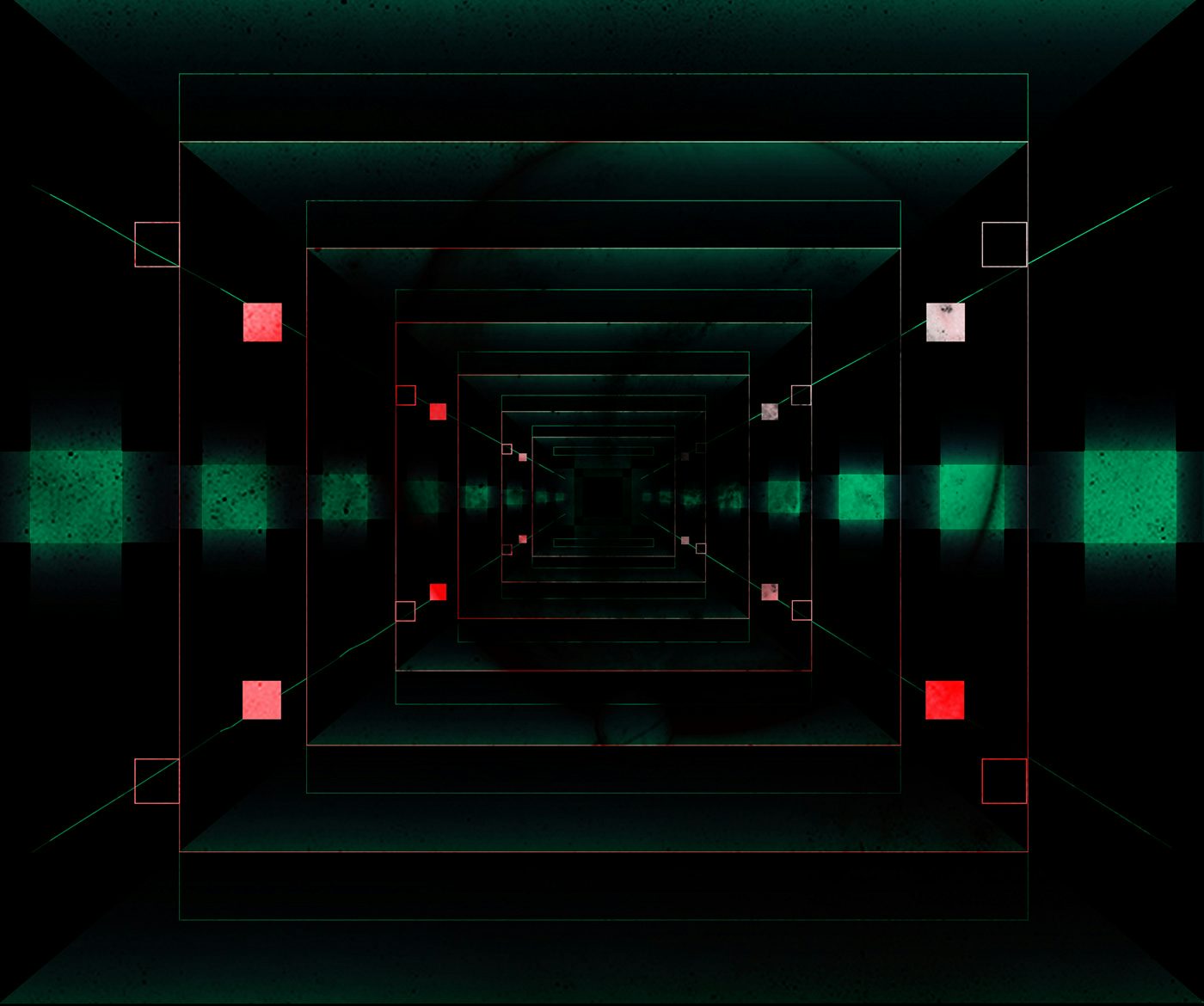
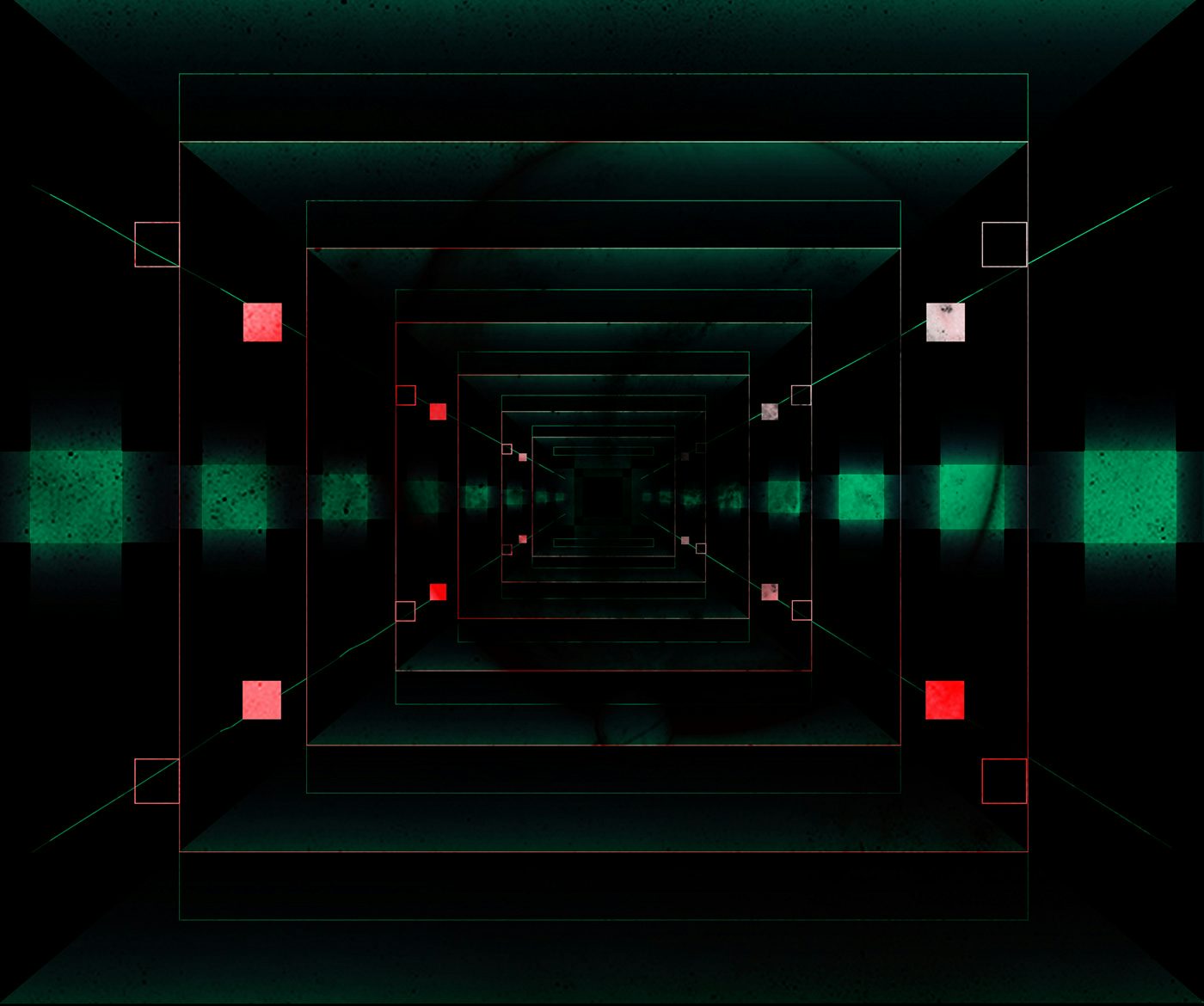
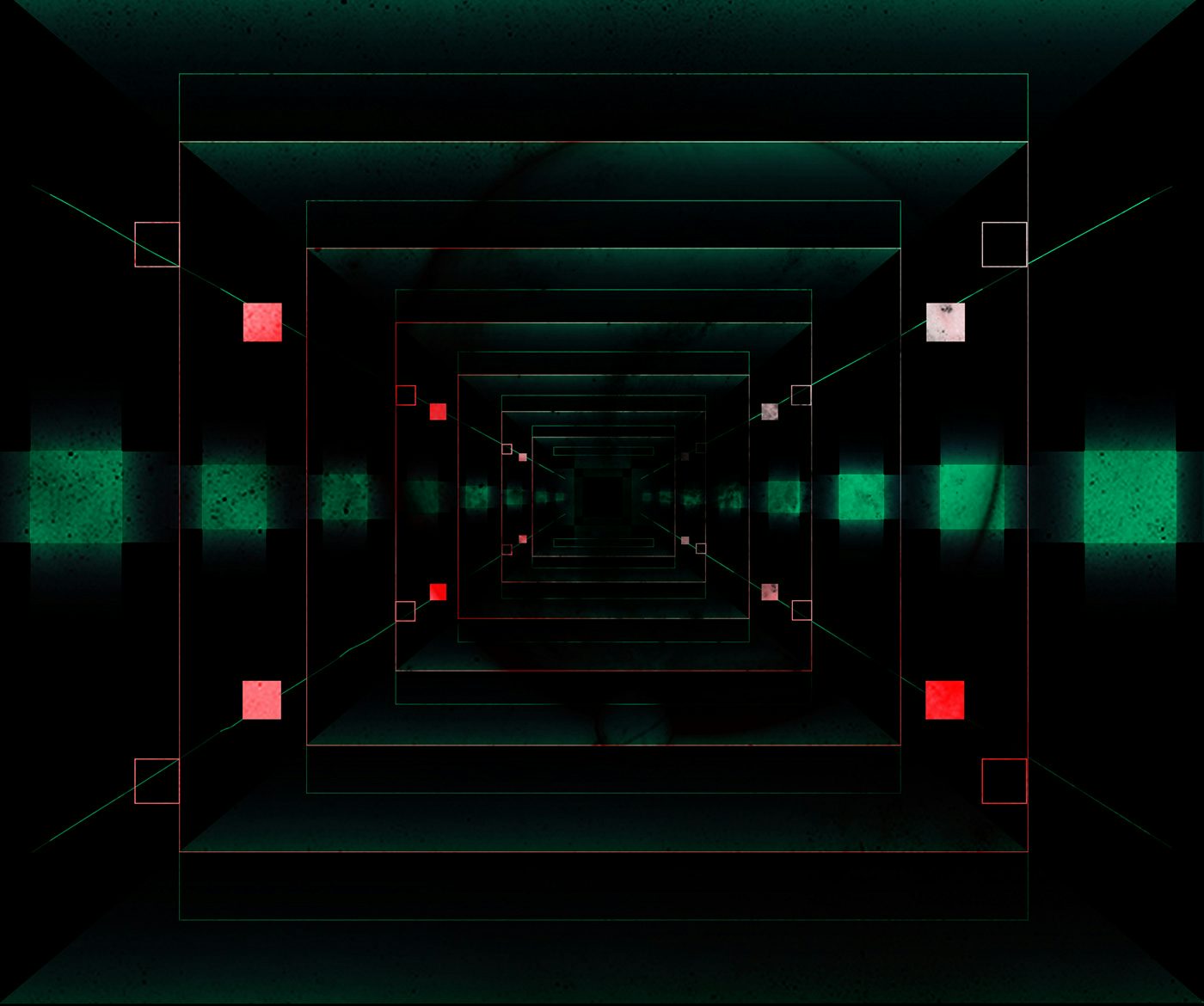
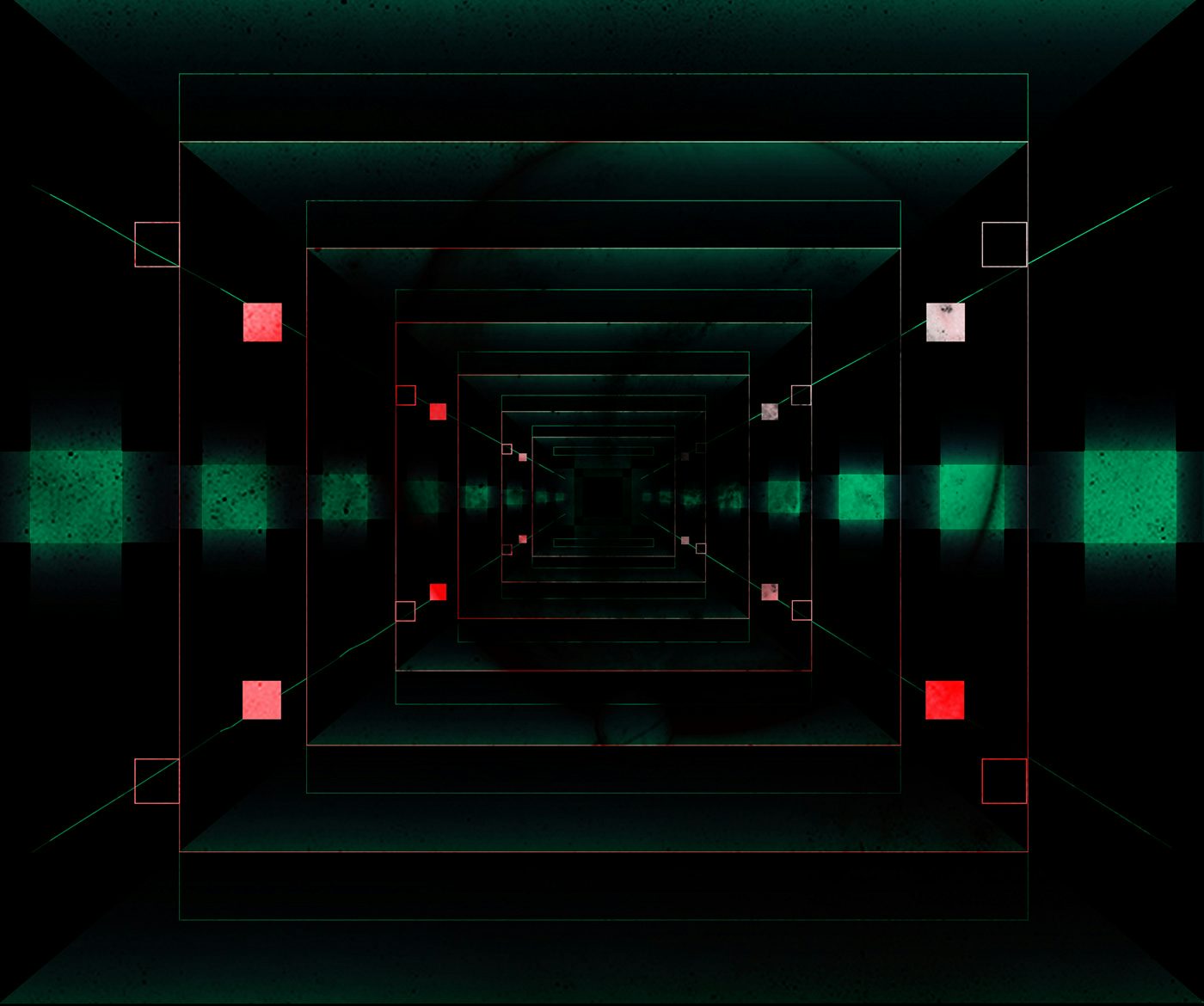
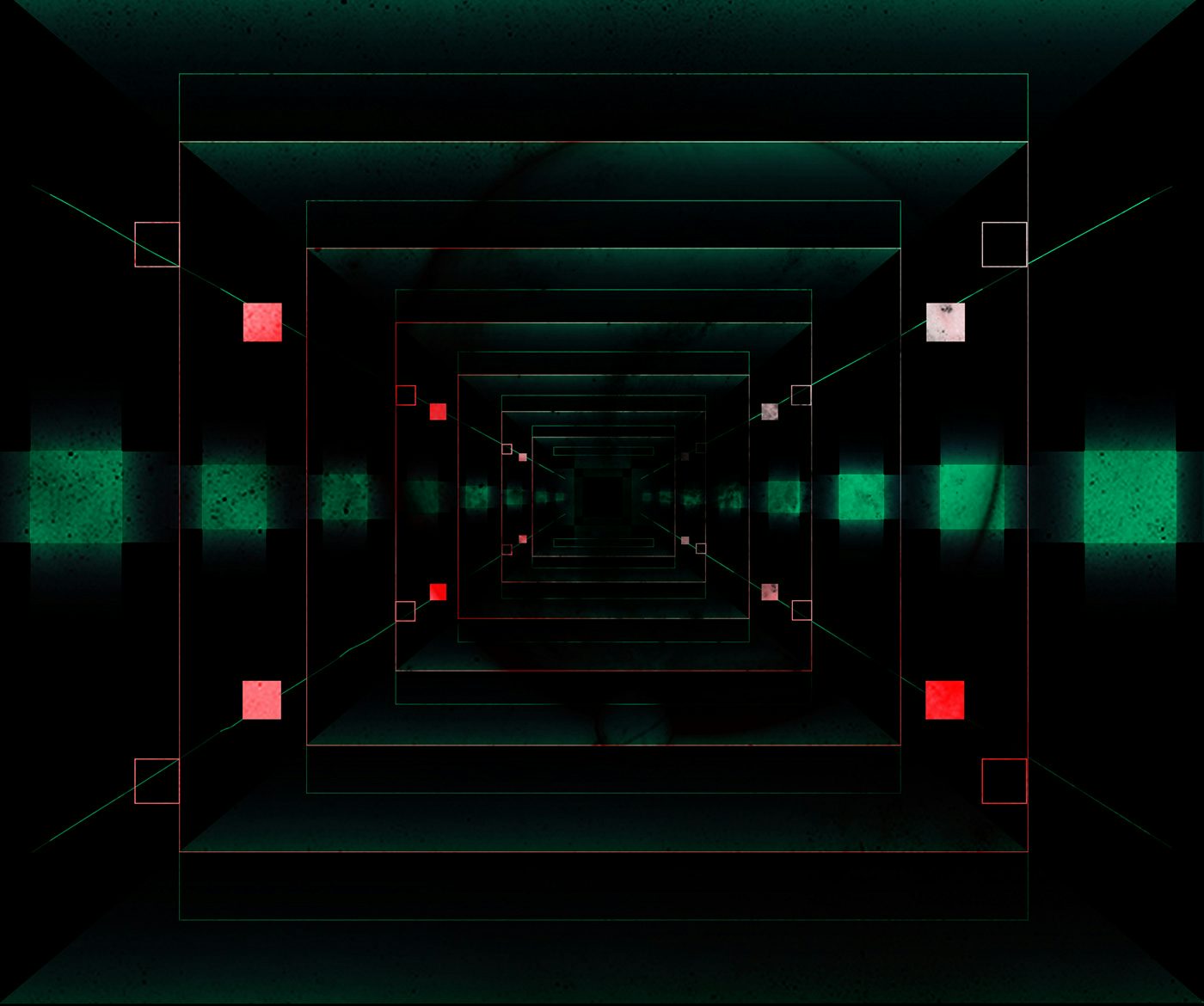
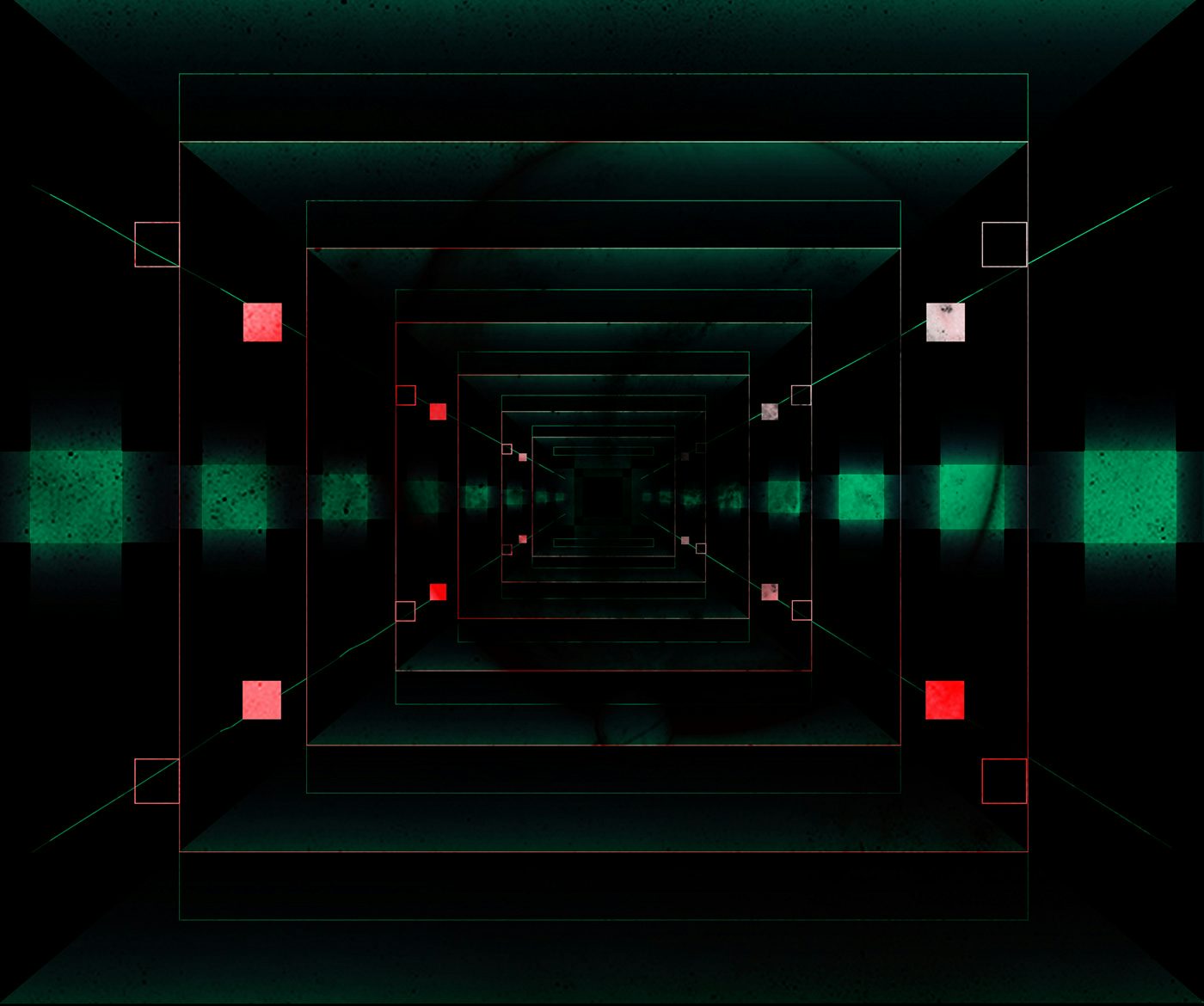
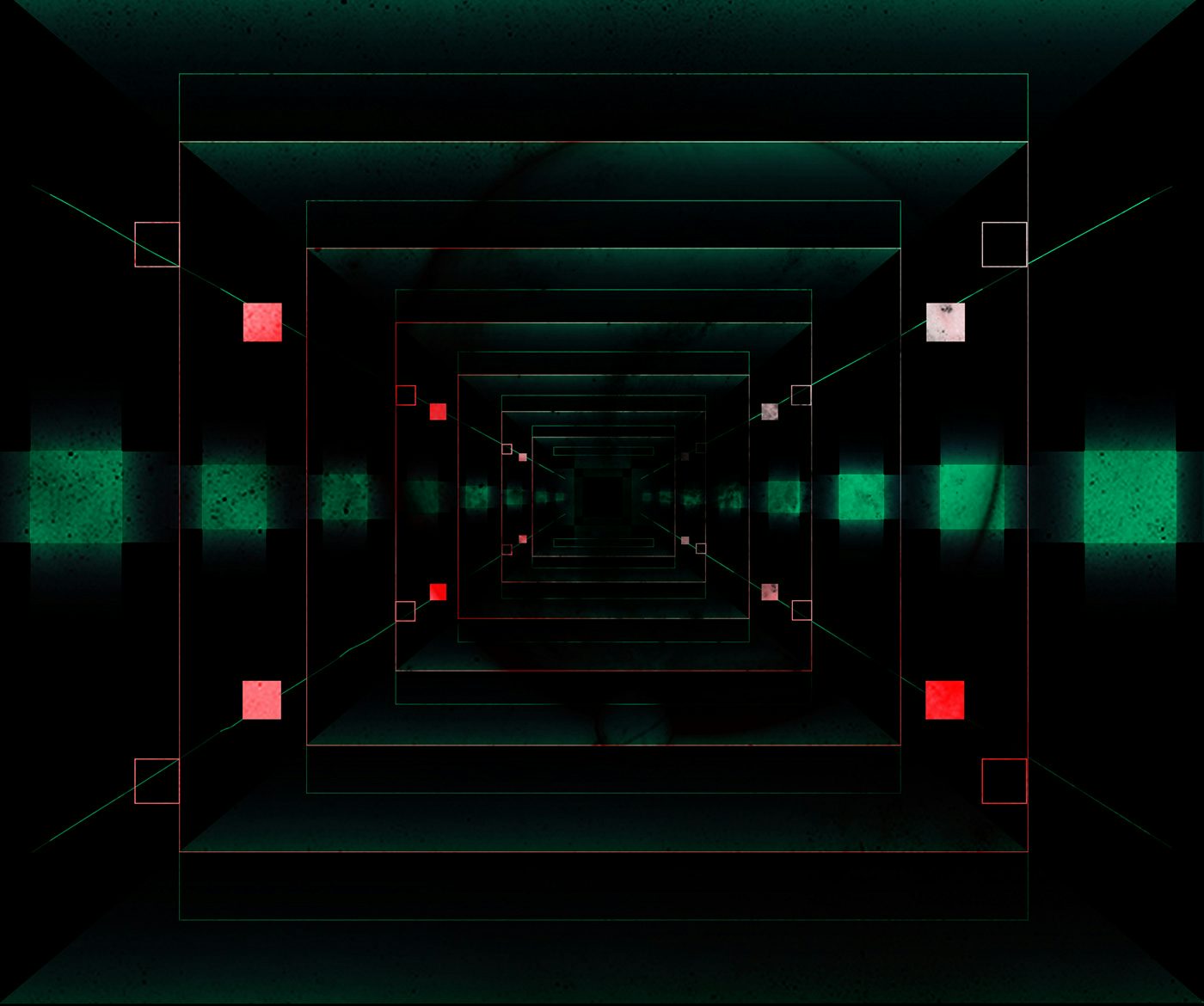
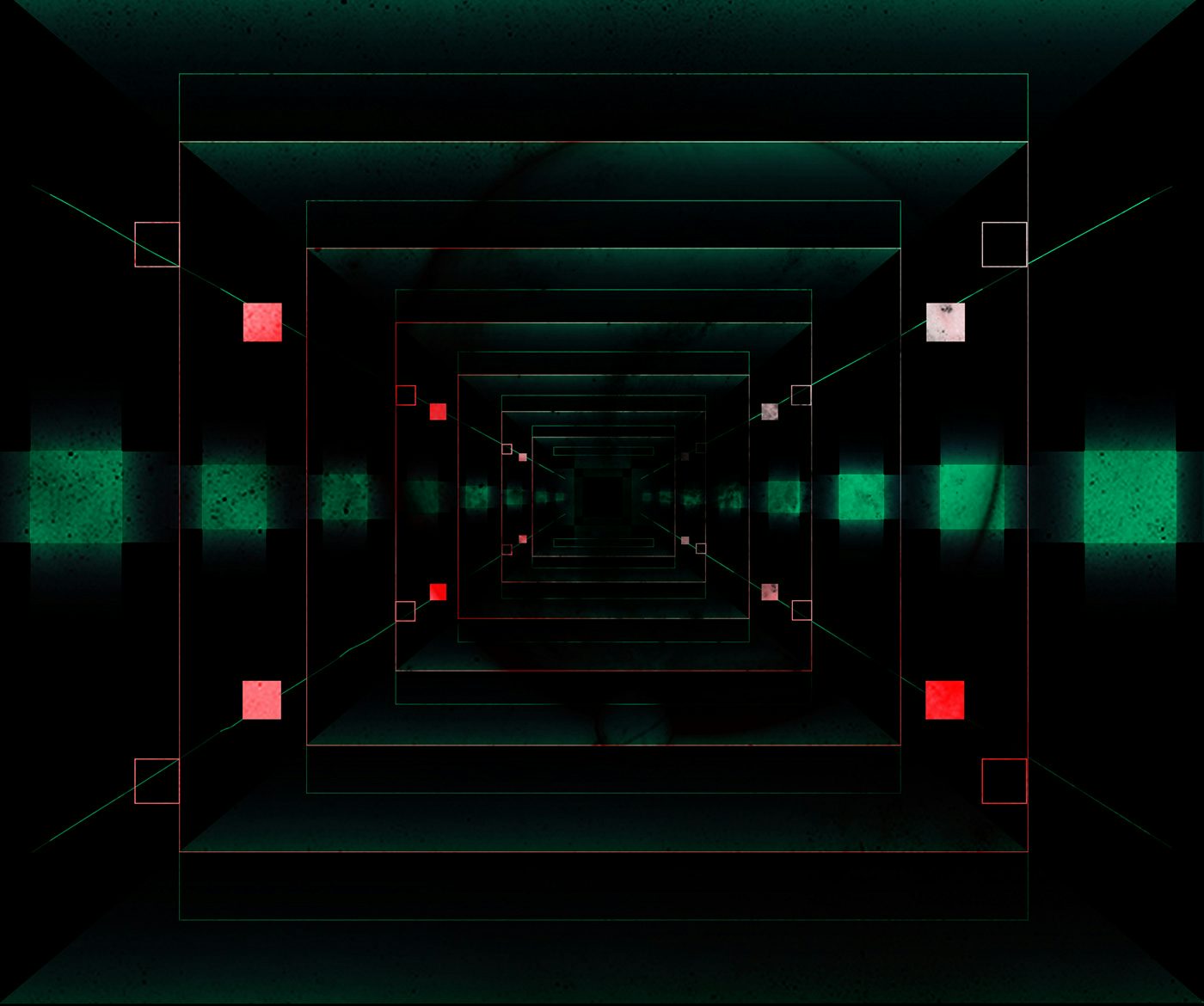
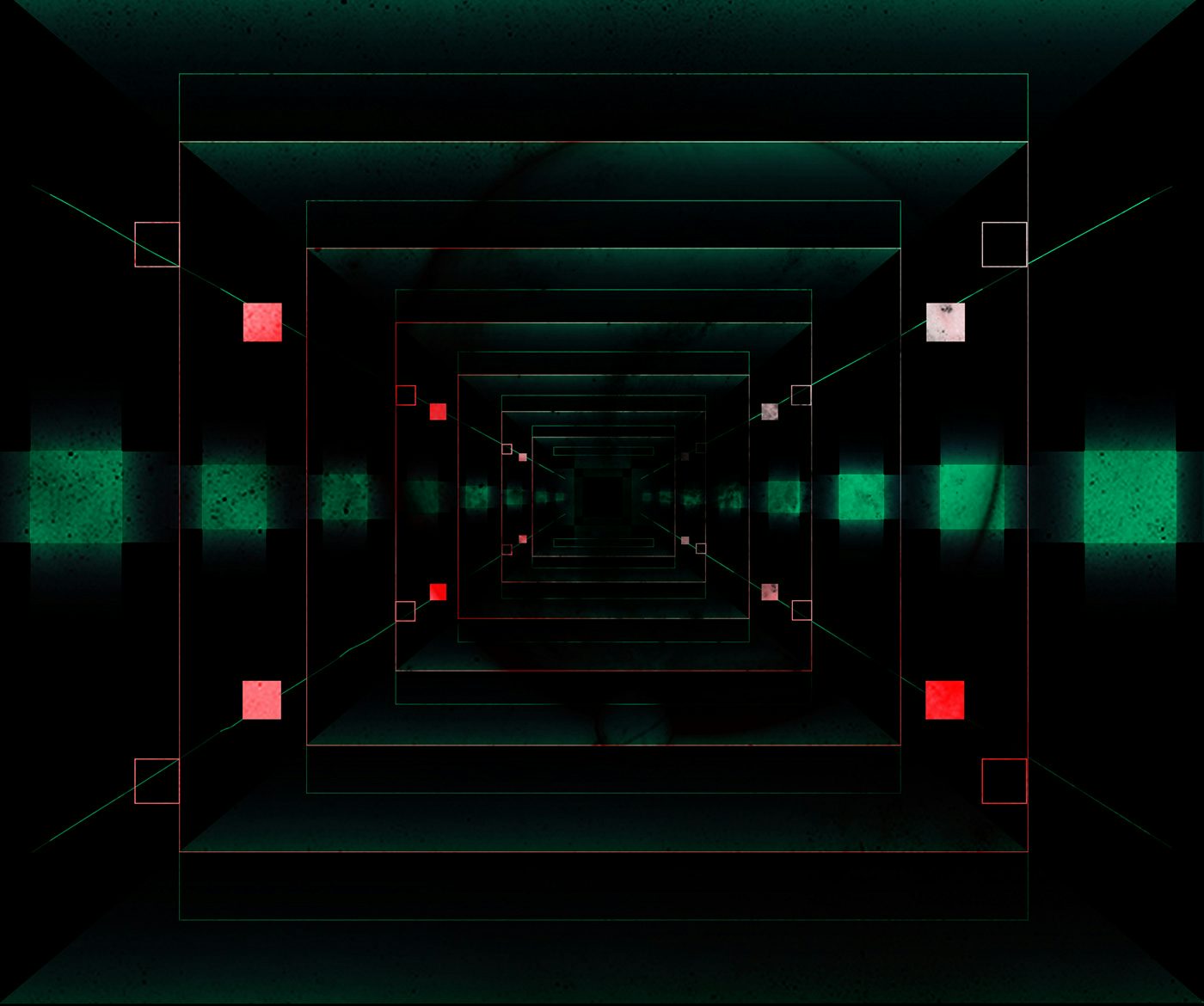
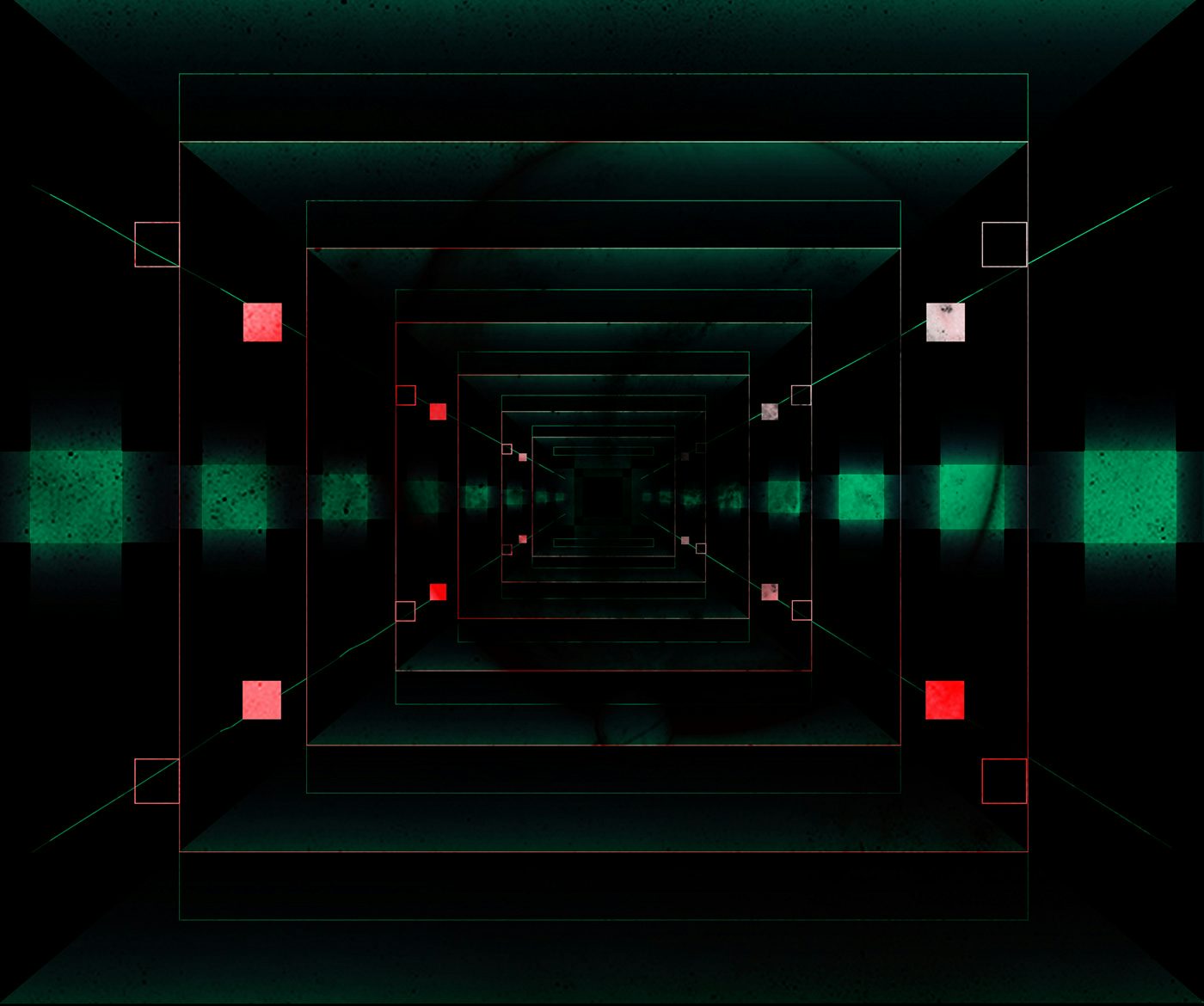
Papers in this project
Physics of financial networks
Statistical physics contributes to new models and metrics for the study of financial network structure, dynamics, stability and instability.
Network valuation in finance
Consistent valuation of interbank claims within an interconnected financial system can be found with a recursive update of banks' equities.
Financial network reconstruction
Statistical mechanics concepts reconstruct connections between financial institutions and the stock market, despite limited data disclosure.
Pathways towards instability
Processes believed to stabilize financial markets can drive them towards instability by creating cyclical structures that amplify distress.
Networks of credit default swaps
Time series data from networks of credit default swaps display no early warnings of financial crises without additional macroeconomic indicators.
Memory effects in stock dynamics
The likelihood of stock prices bouncing on specific values increases due to memory effects in the time series data of the price dynamics.
Reconstructing credit
New mathematical tools can help infer financial networks from partial data to understand the propagation of distress through the network.
Complex derivatives
Network-based metrics to assess systemic risk and the importance of financial institutions can help tame the financial derivatives market.