Spectral partitioning in equitable graphs
The spectral density of graph ensembles provides an exact solution to the graph partitioning problem and helps detect community structure.
Physical Review E 95, 62310 (2017)
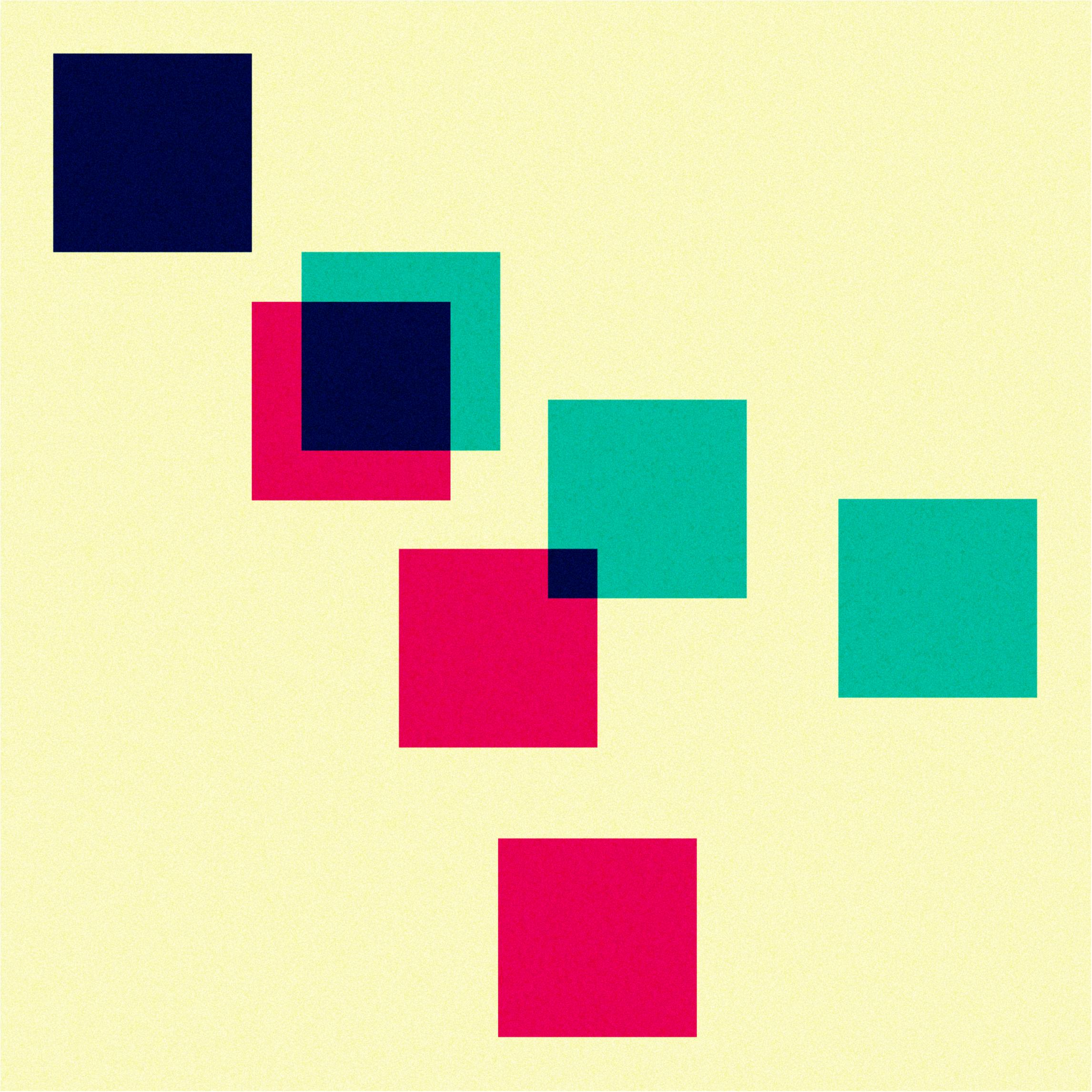
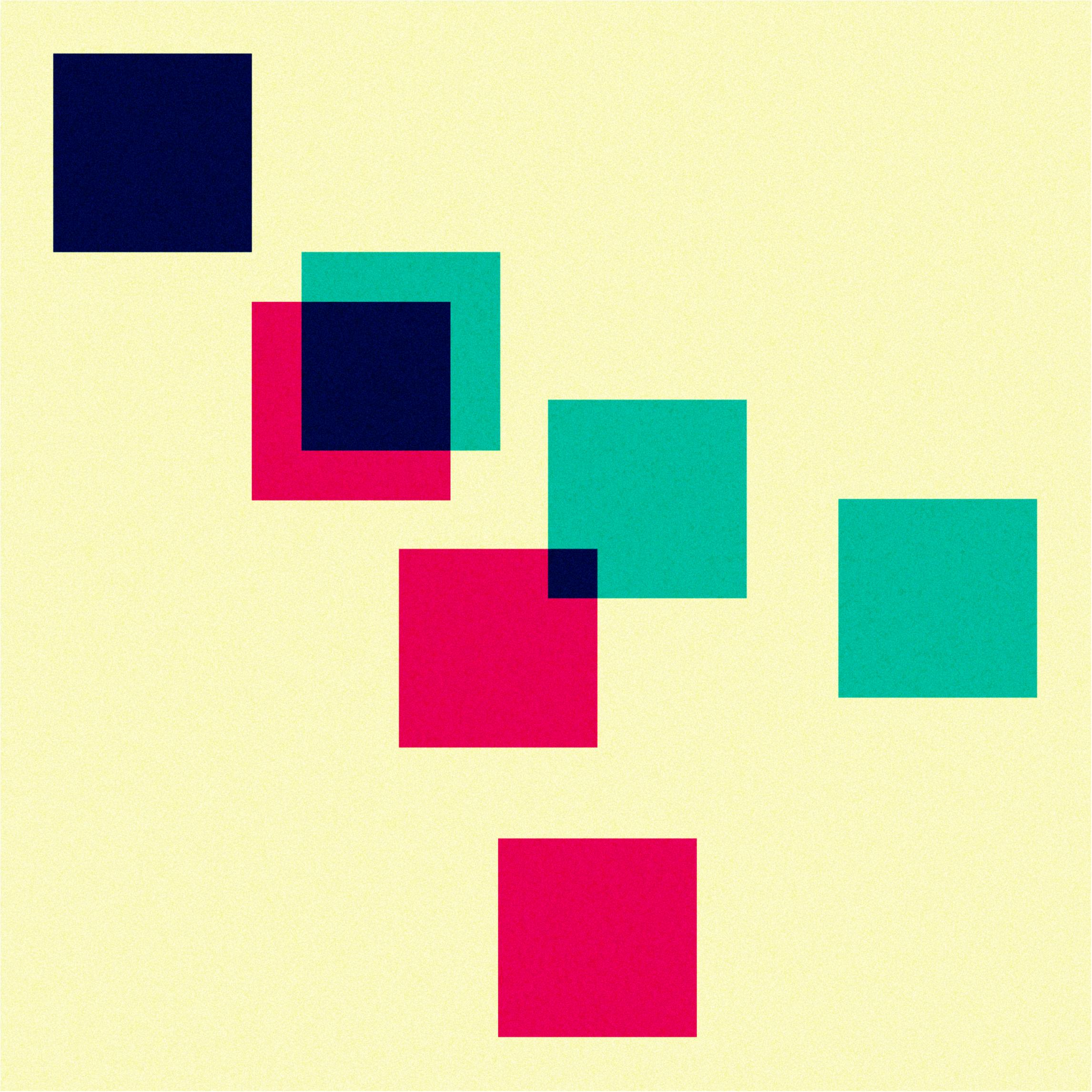
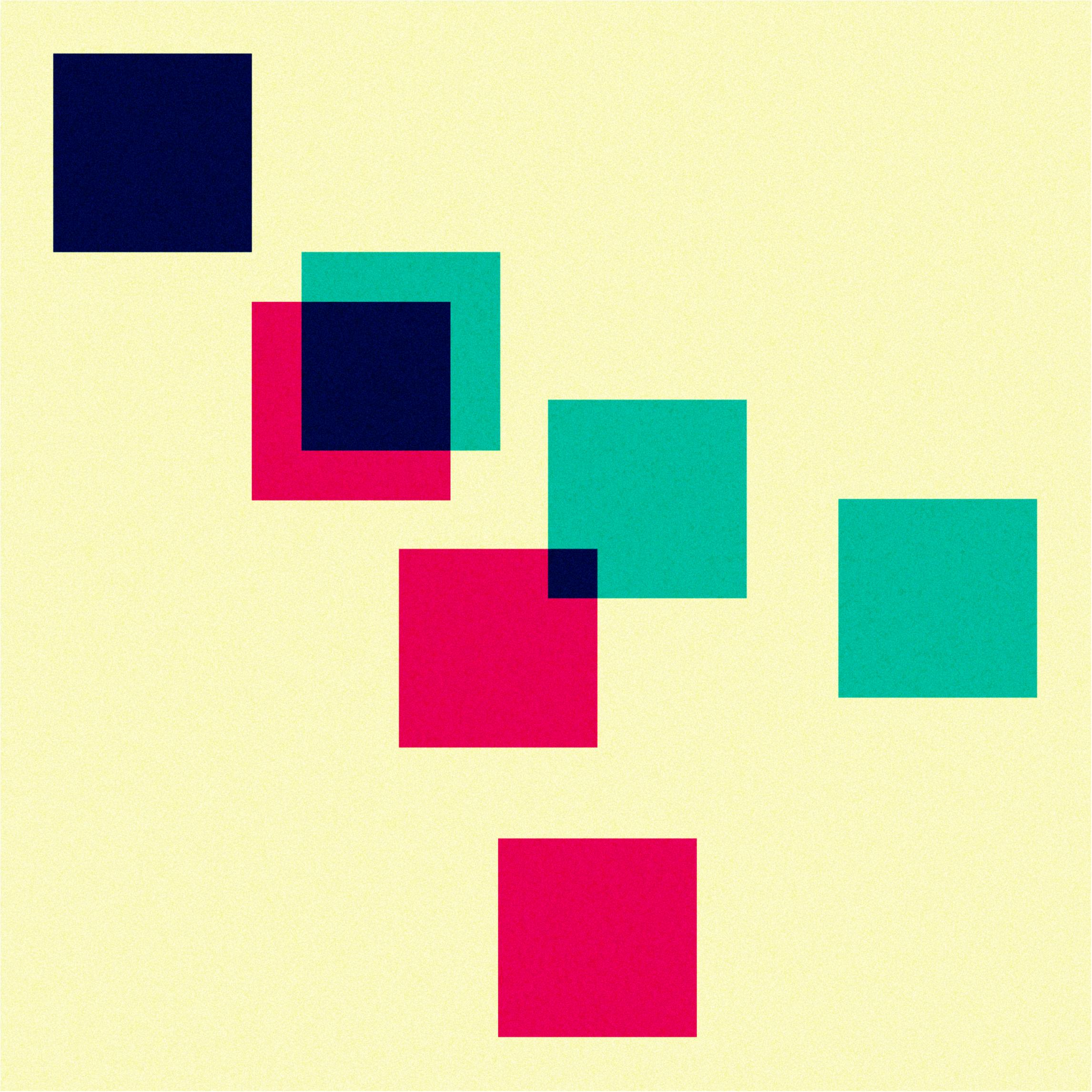
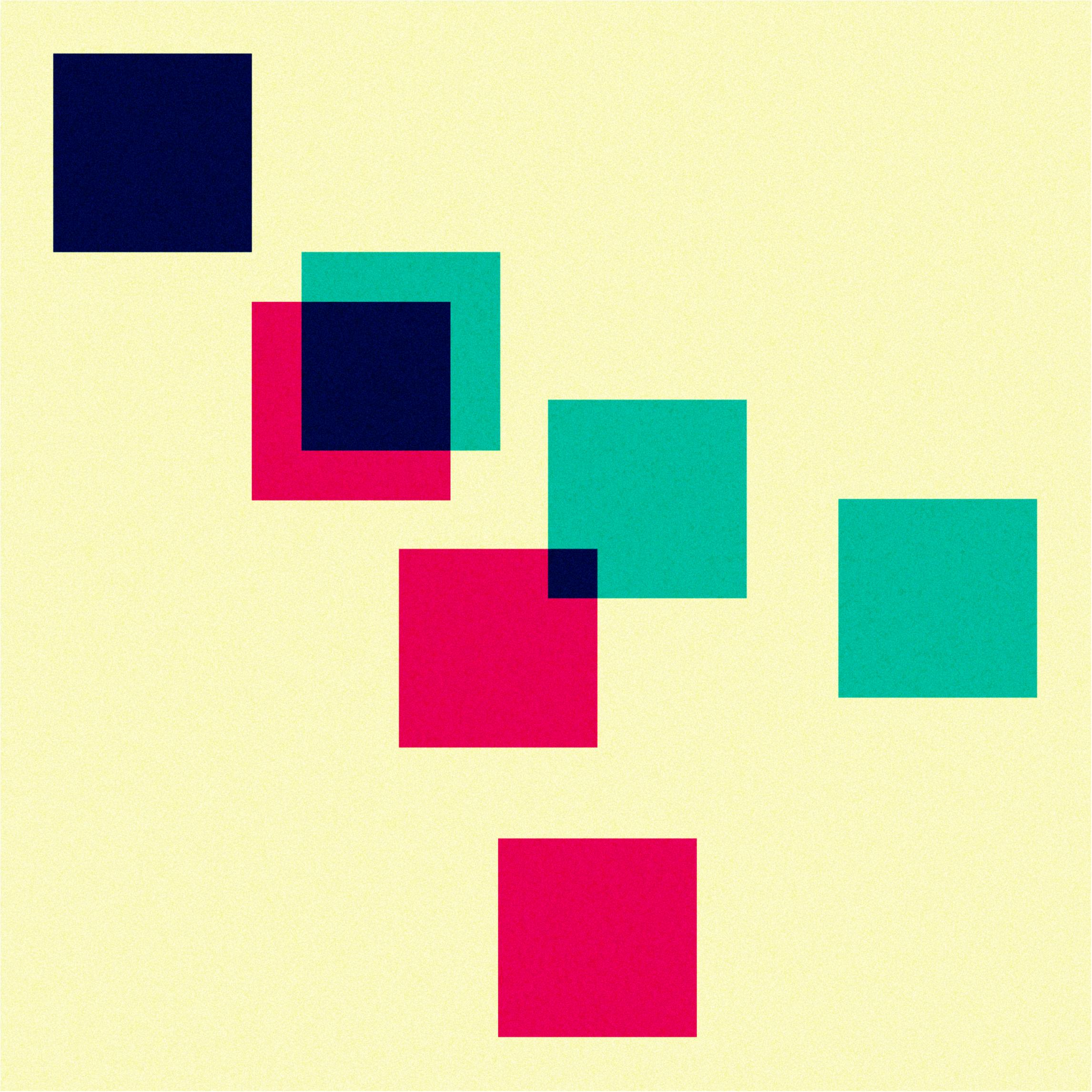
LCP
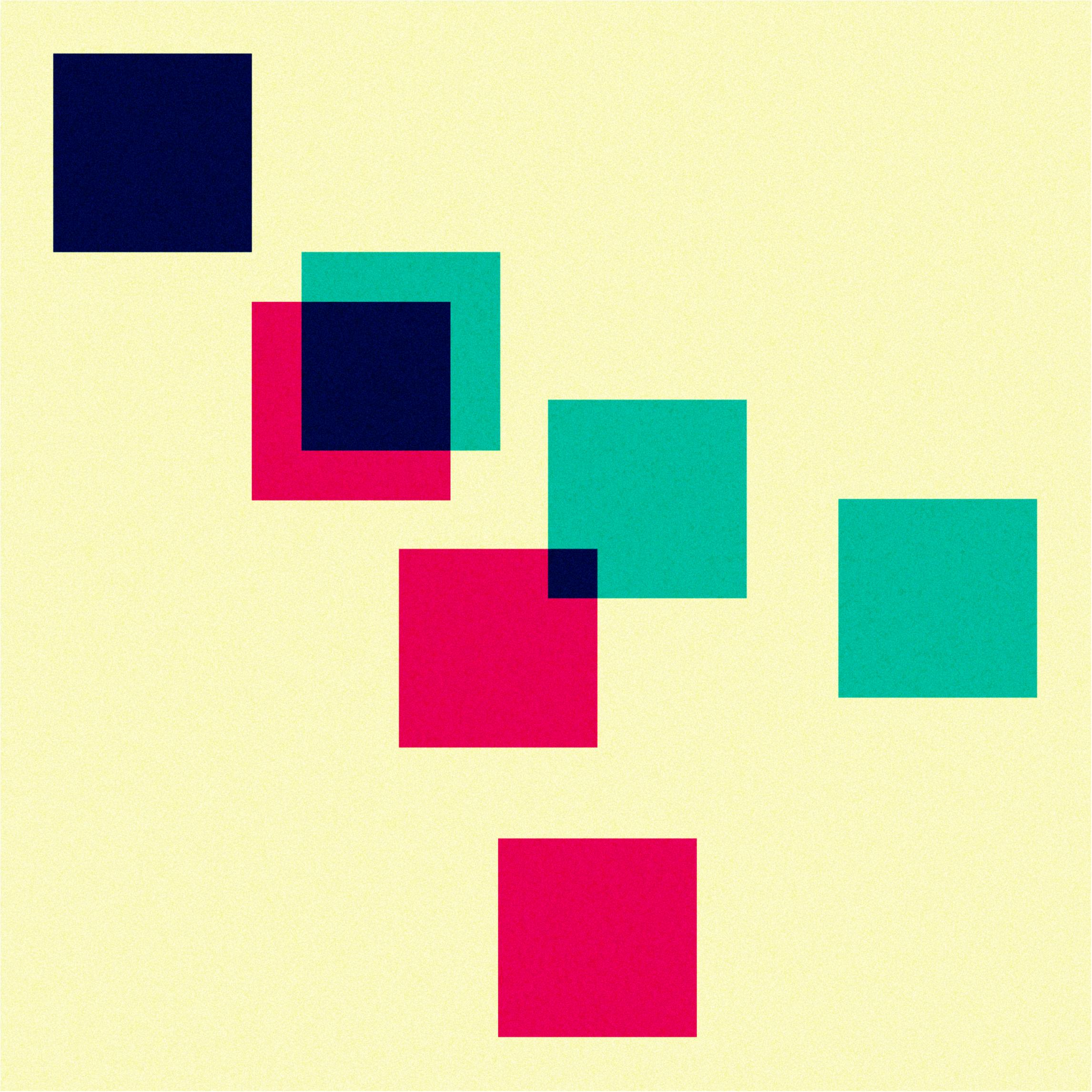
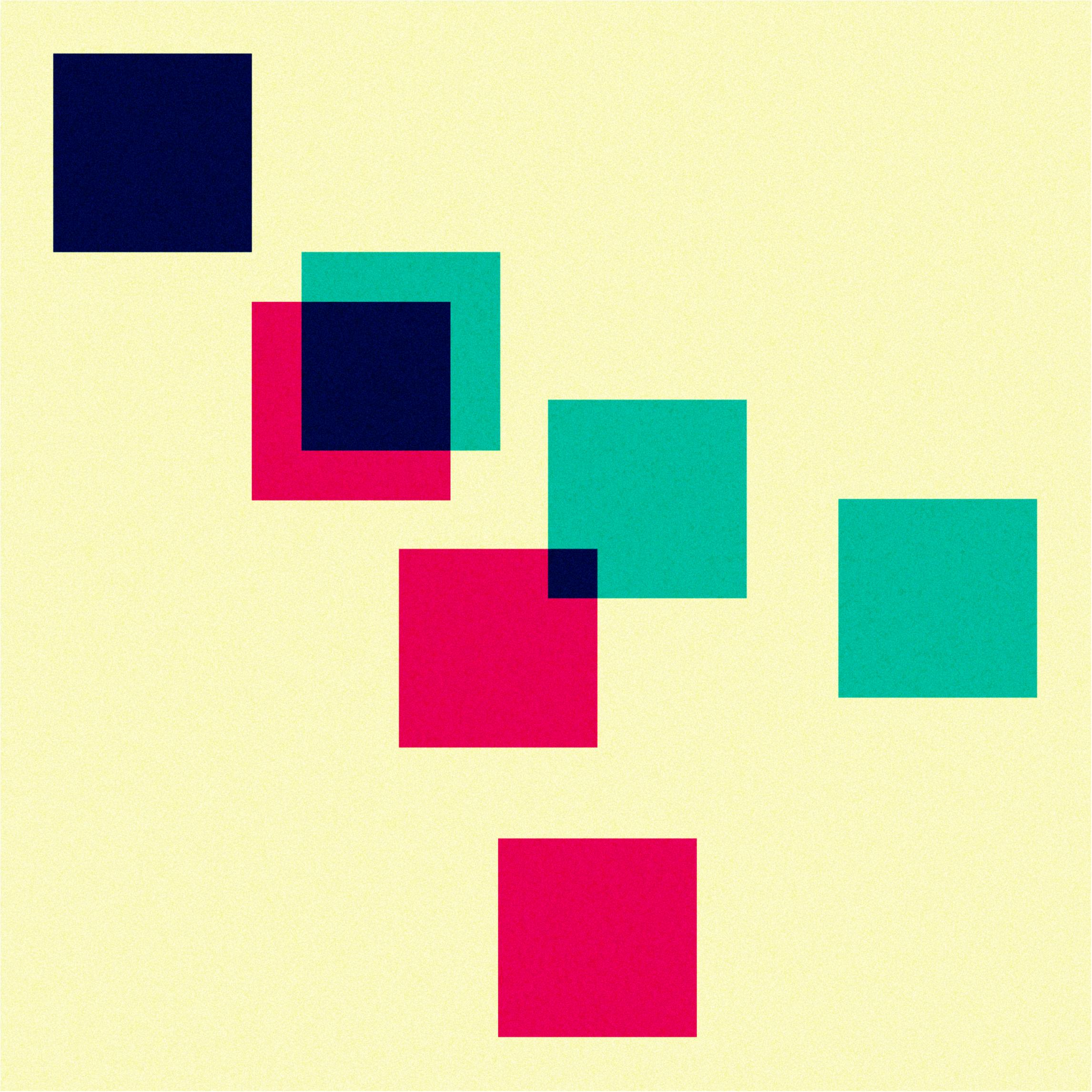
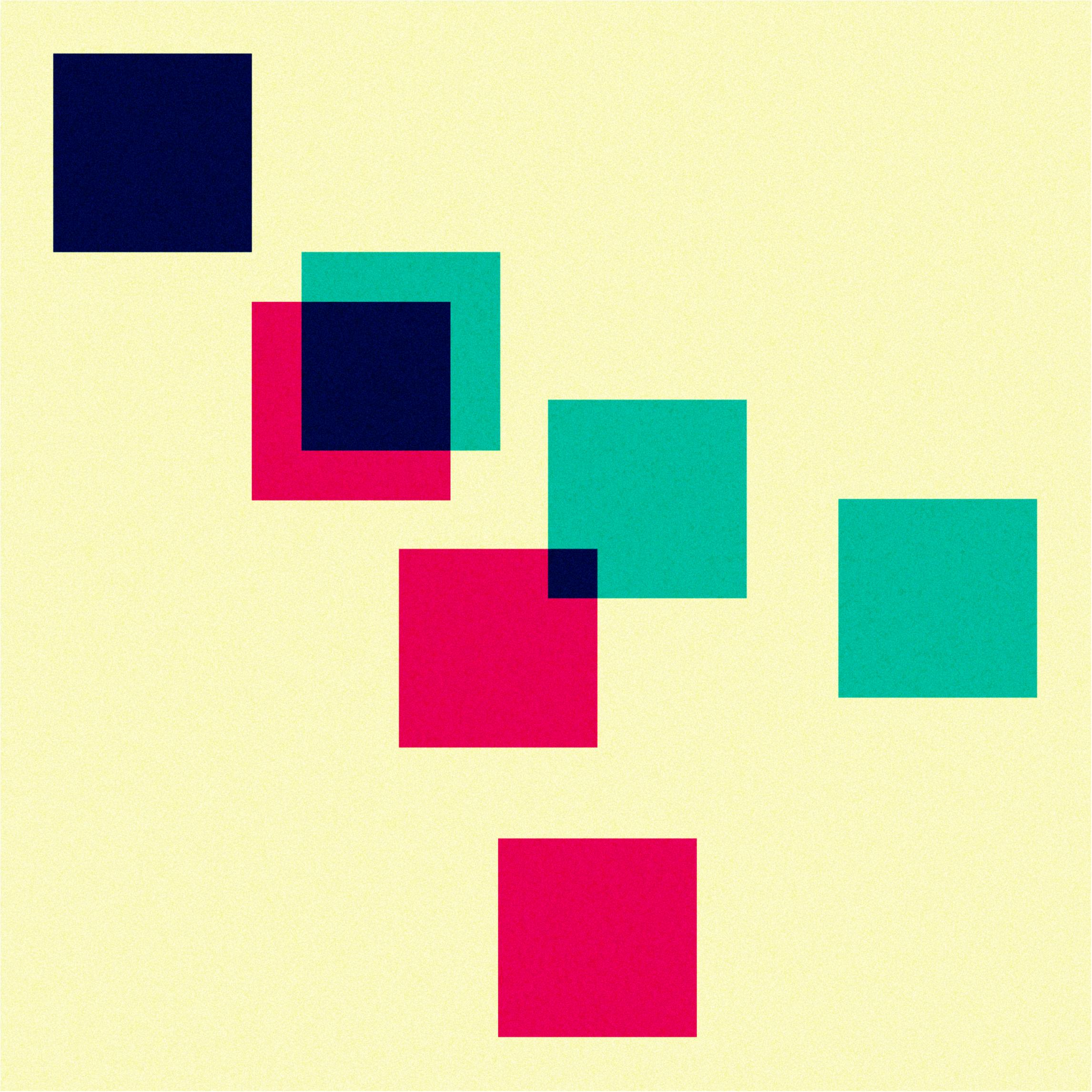
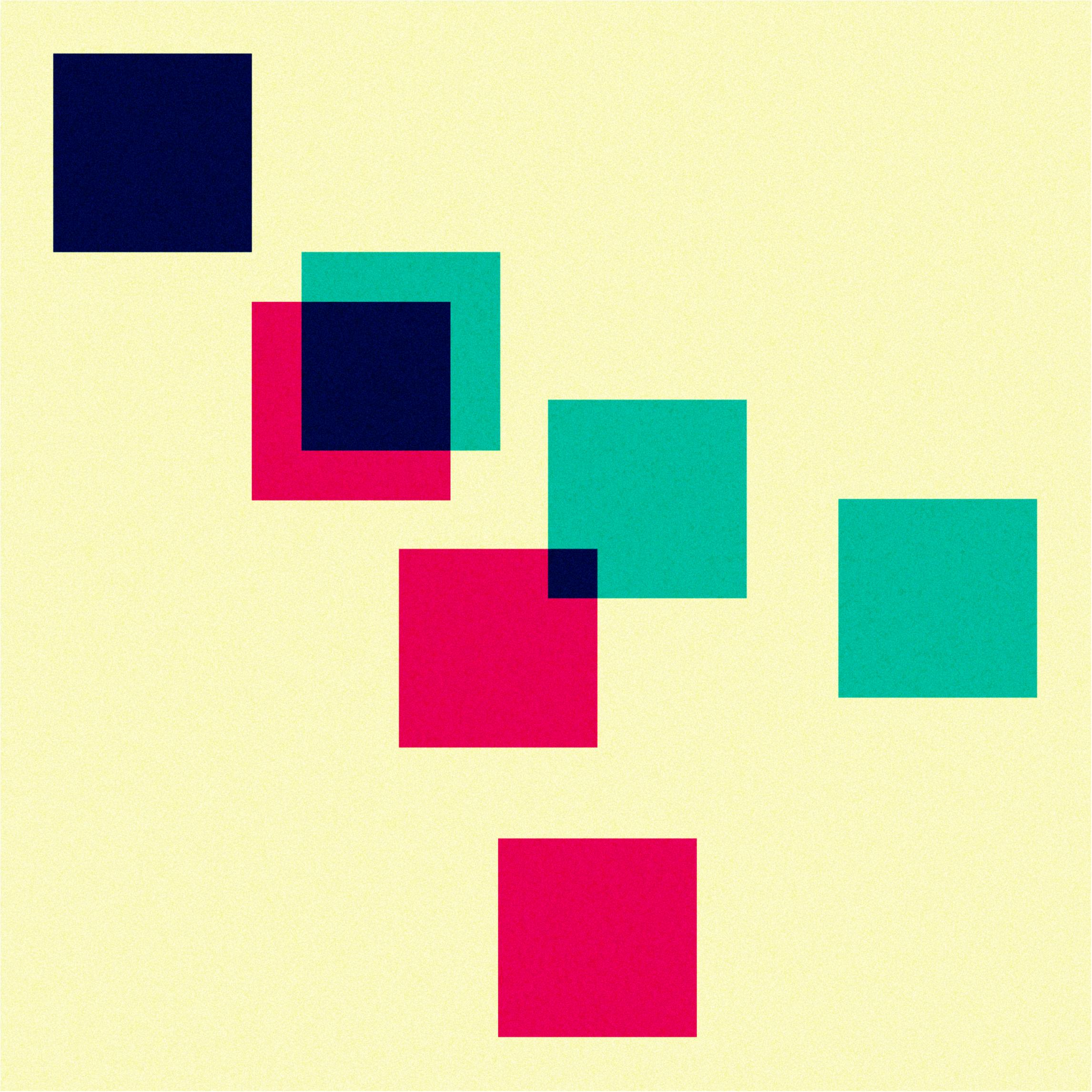
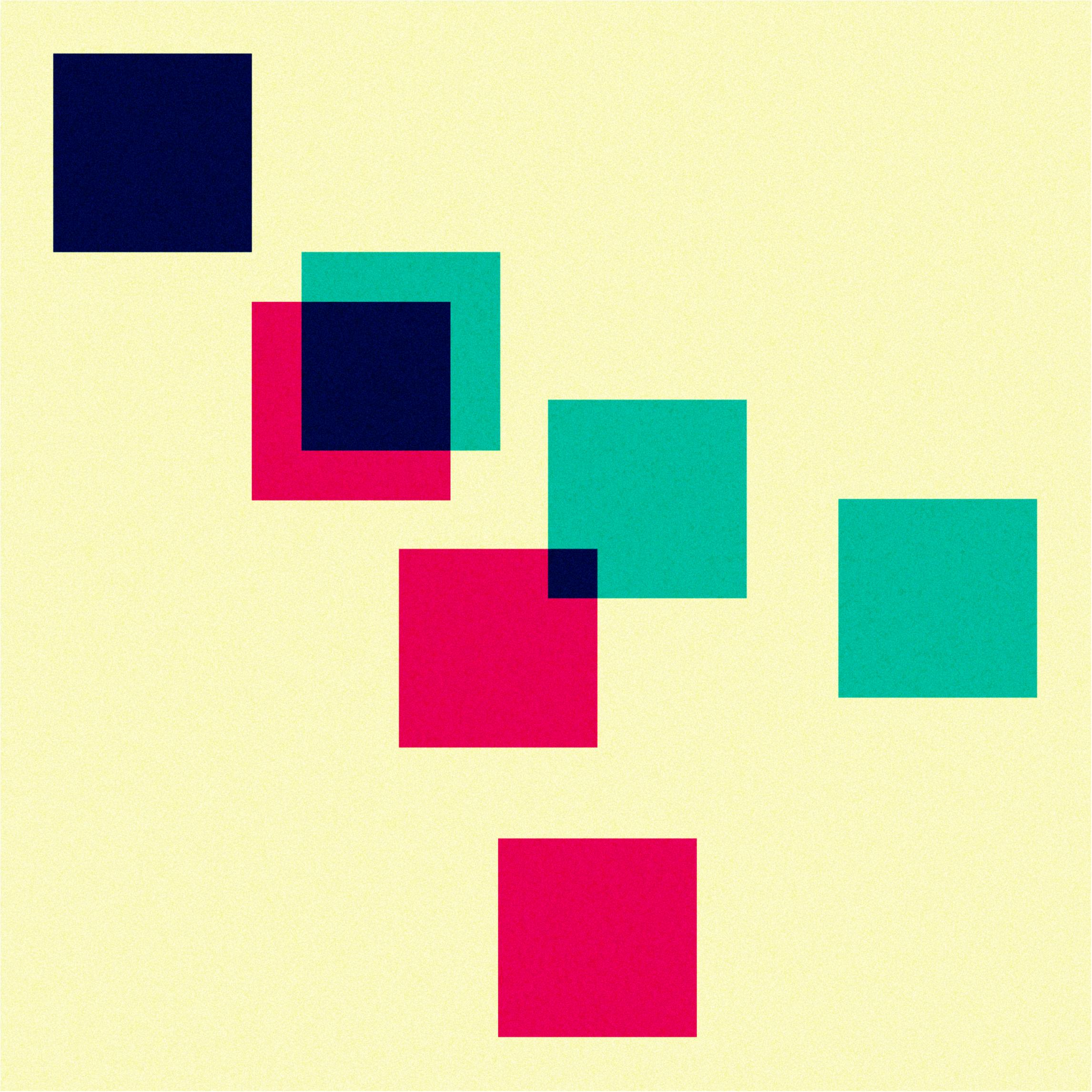
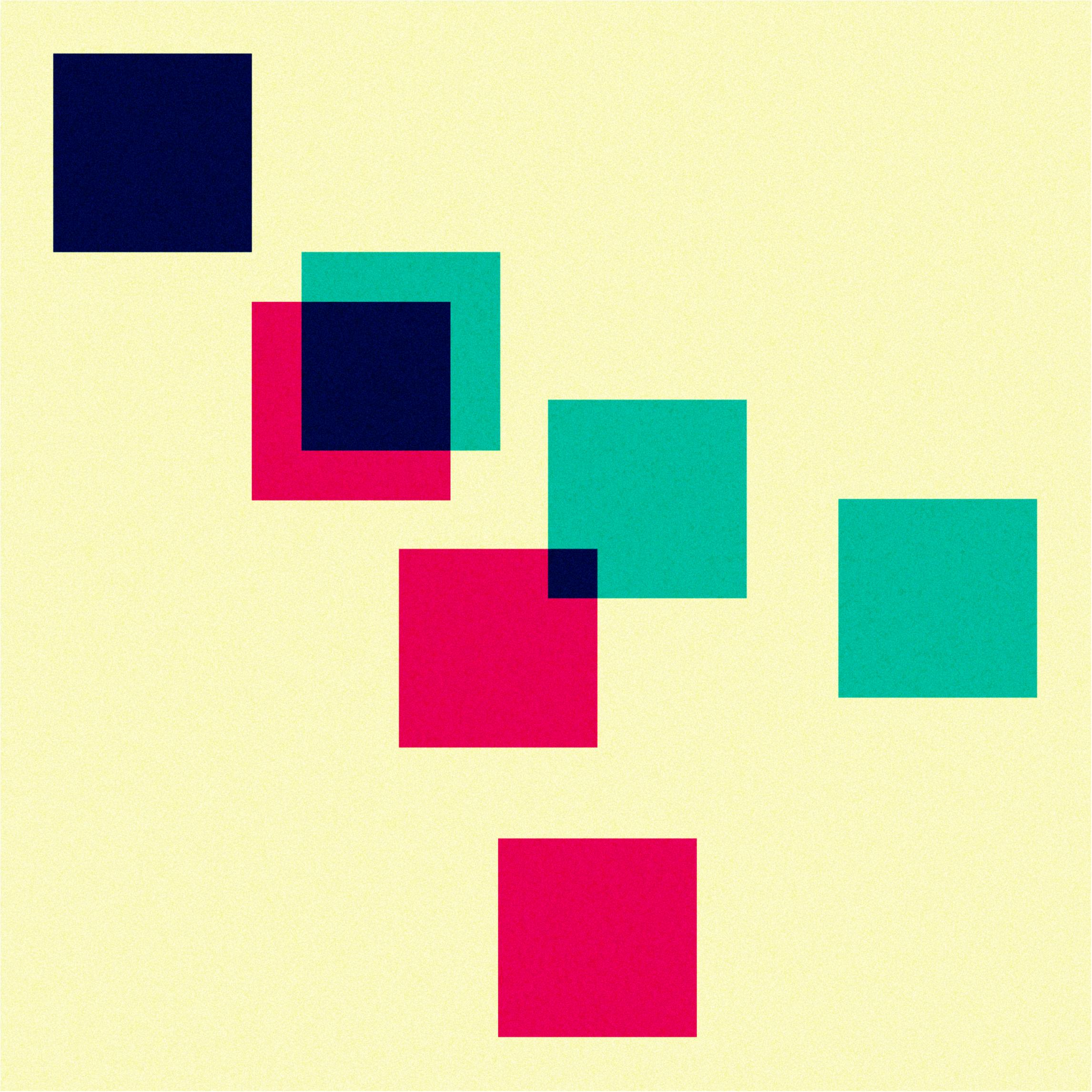
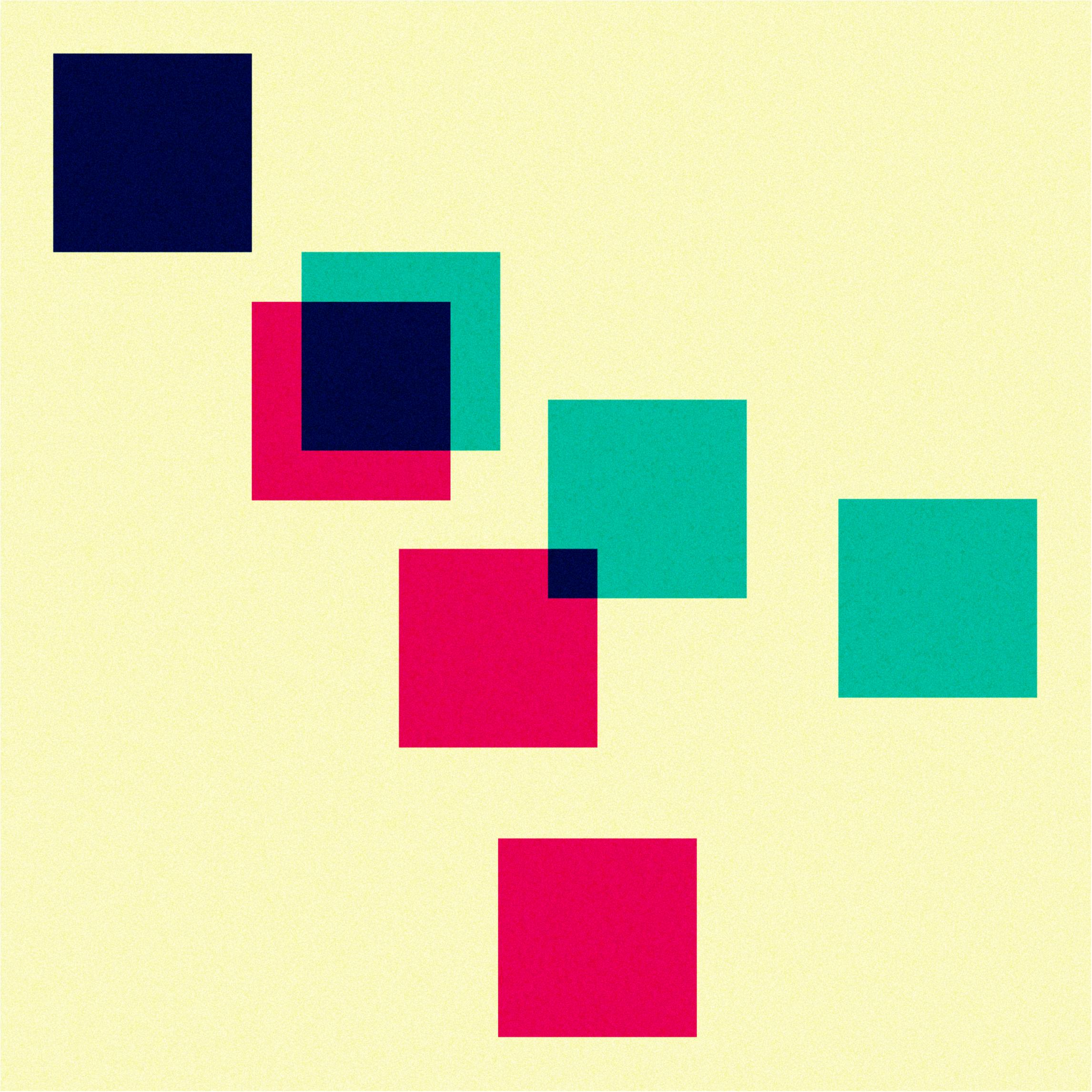
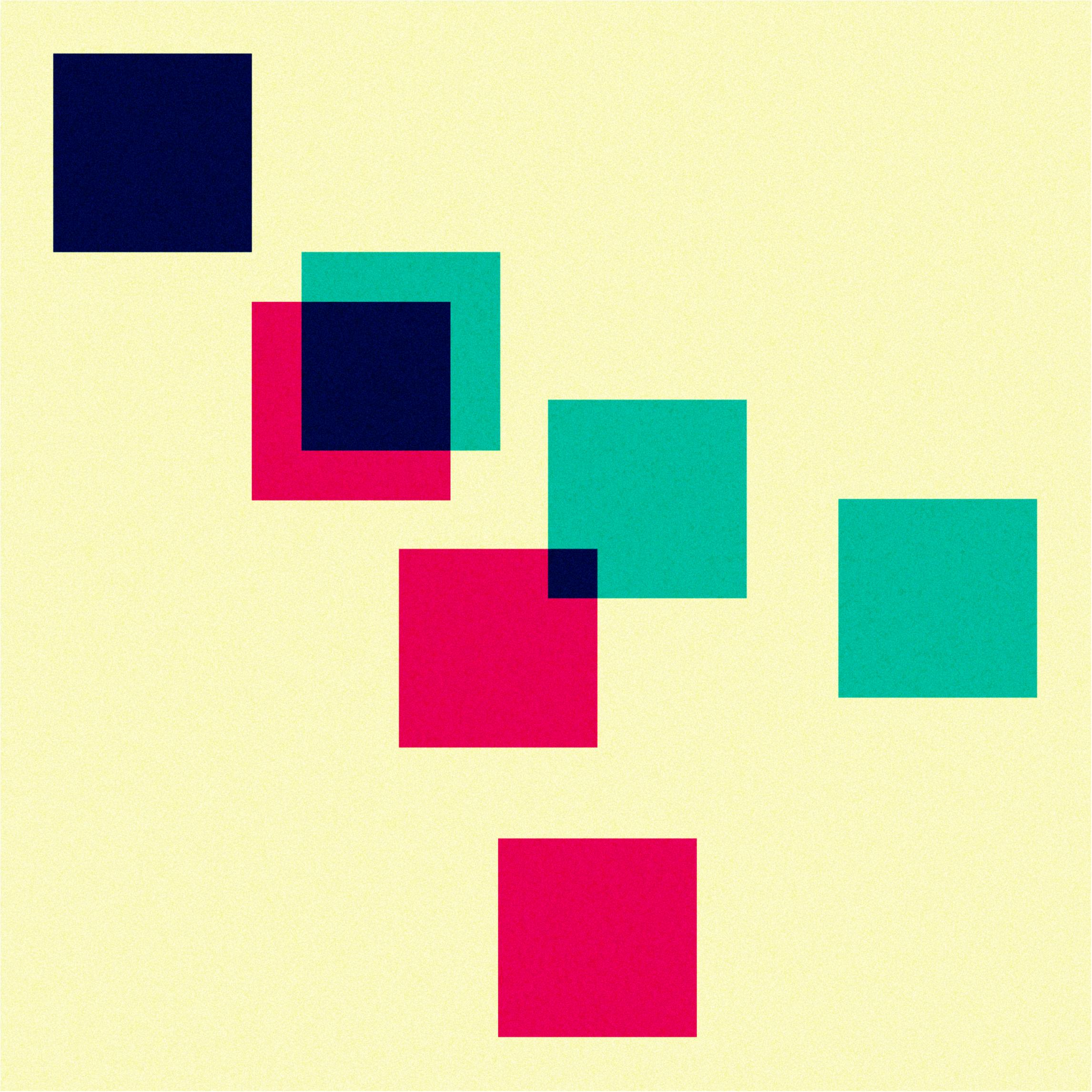
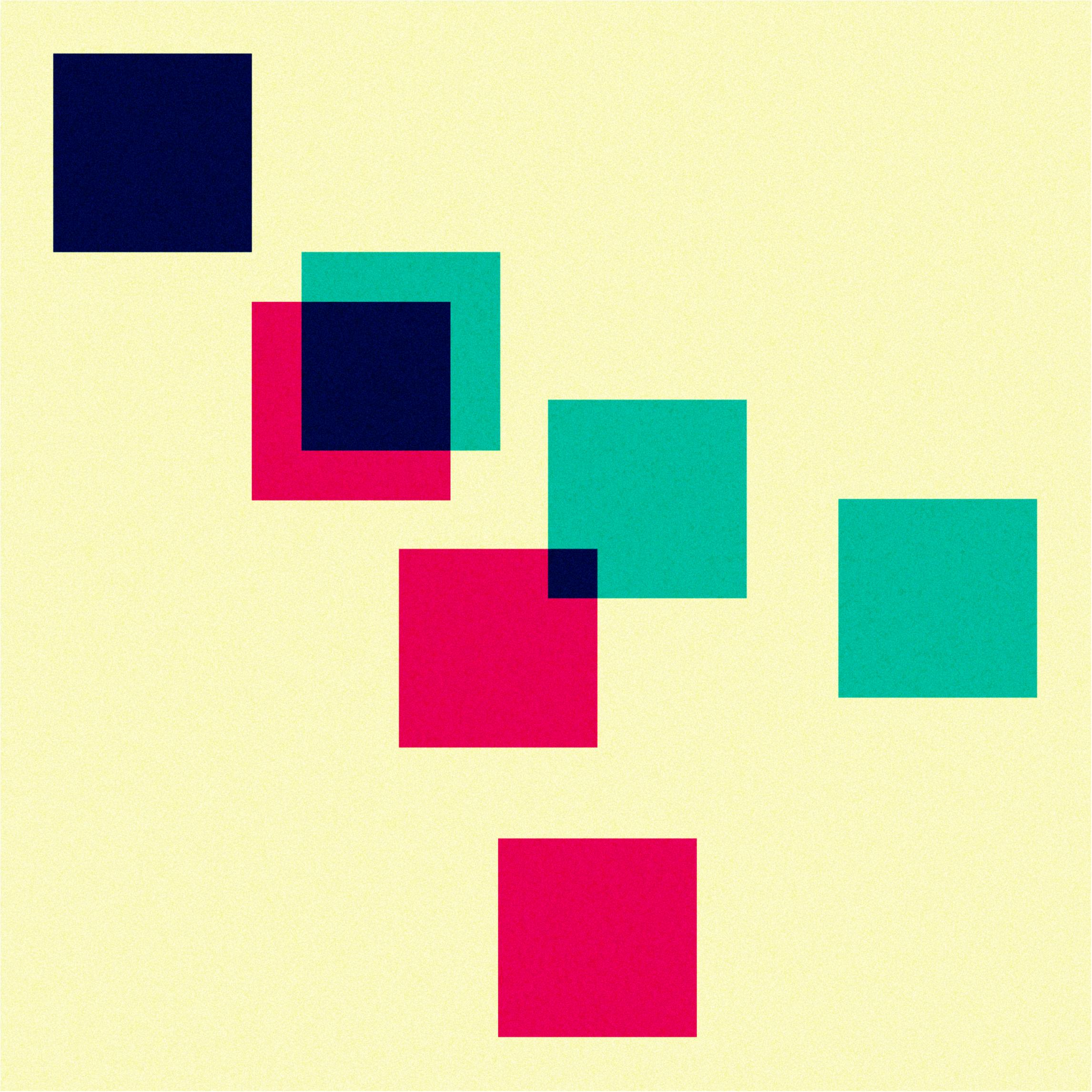
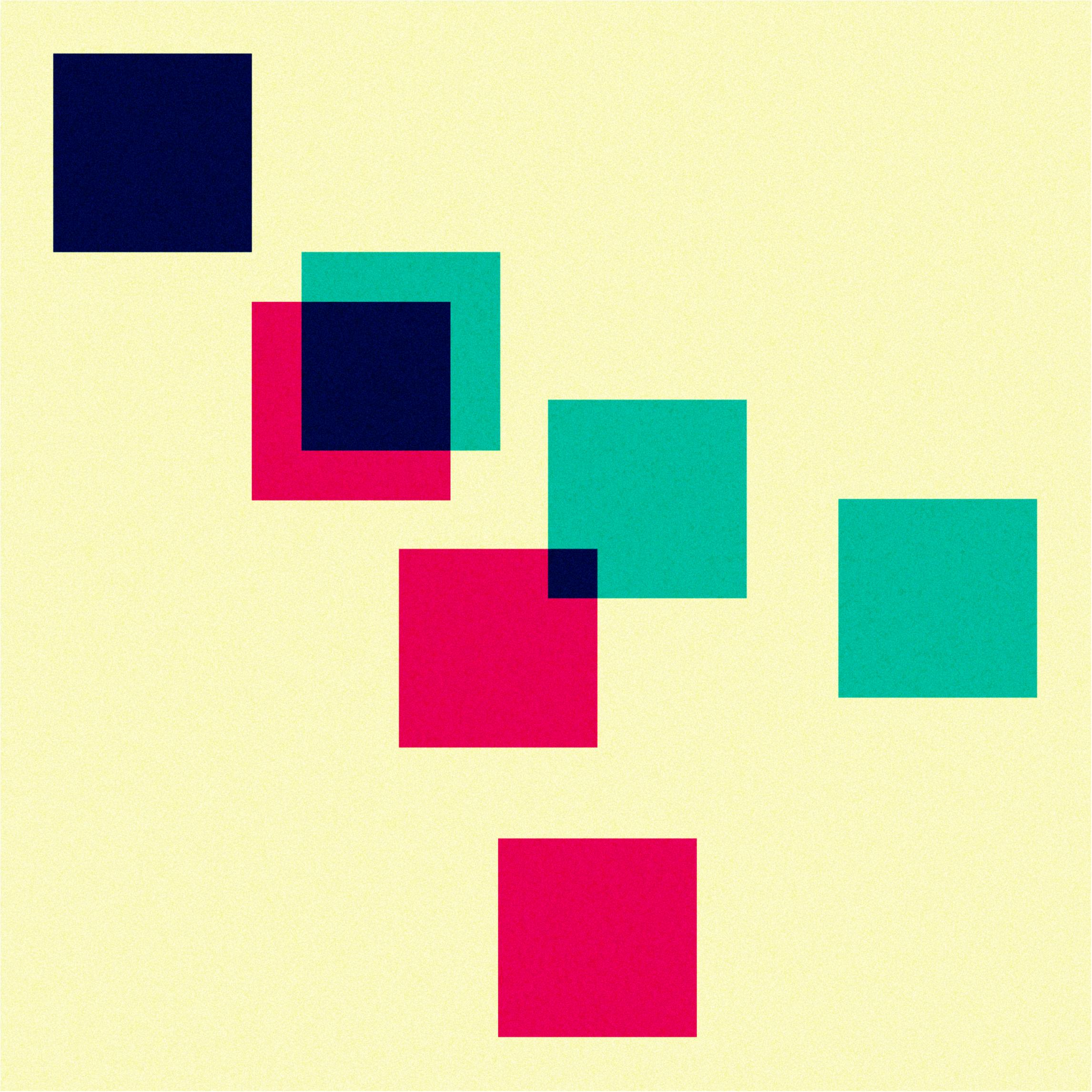
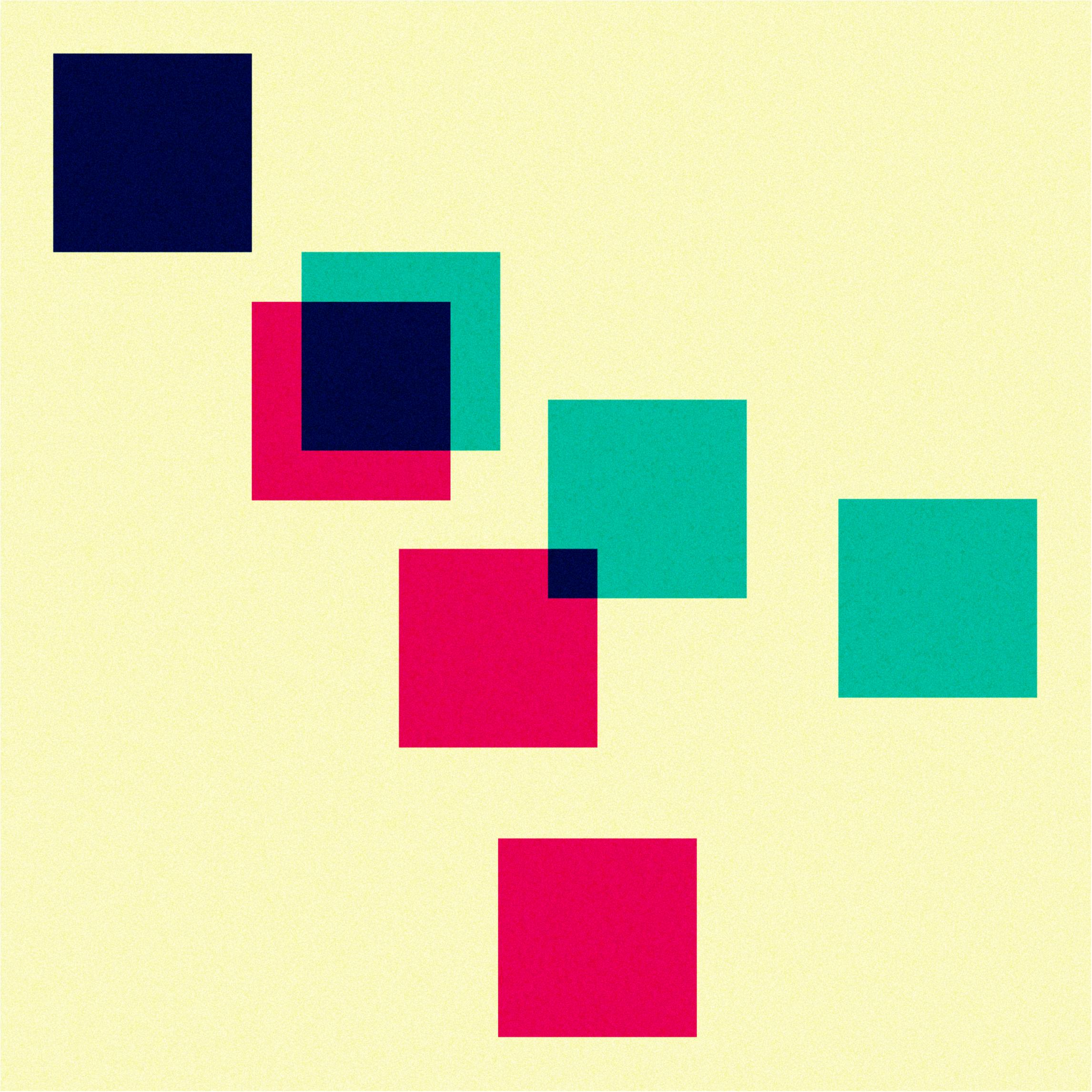
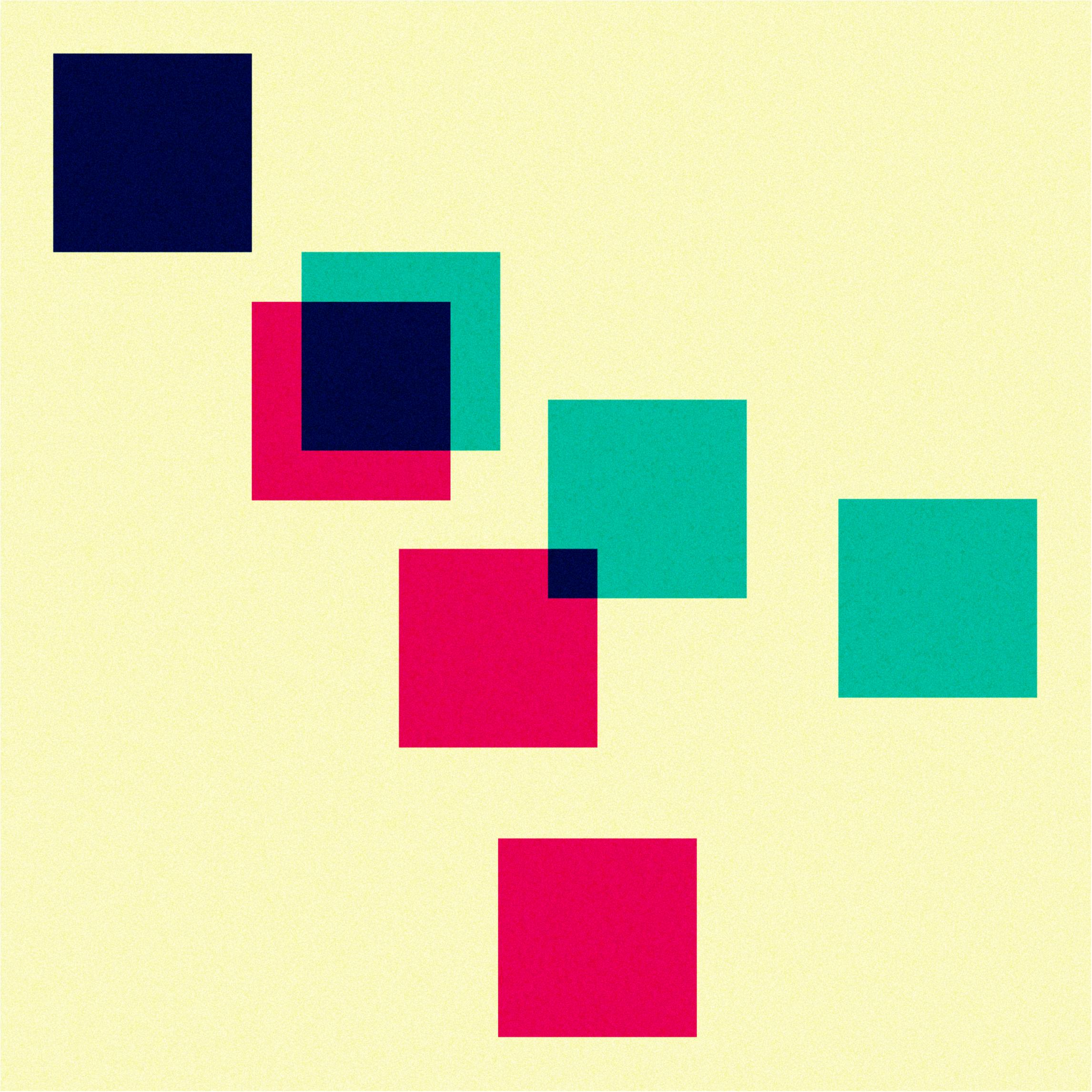
Graph partitioning problems emerge in a wide variety of complex systems, ranging from biology to finance, but can be rigorously analyzed and solved only for a few graph ensembles. Here, an ensemble of equitable graphs, i.e. random graphs with a block-regular structure, is studied, for which analytical results can be obtained. In particular, the spectral density of this ensemble is computed exactly for a modular and bipartite structure. Kesten-McKay’s law for random regular graphs is found analytically to apply also for modular and bipartite structures when blocks are homogeneous. Exact solution to graph partitioning for two equal-sized communities is proposed and verified numerically, and a conjecture on the absence of an efficient recovery detectability transition in equitable graphs is suggested. Final discussion summarizes results and outlines their relevance for the solution of graph partitioning problems in other graph ensembles, in particular for the study of detectability thresholds and resolution limits in stochastic block models.