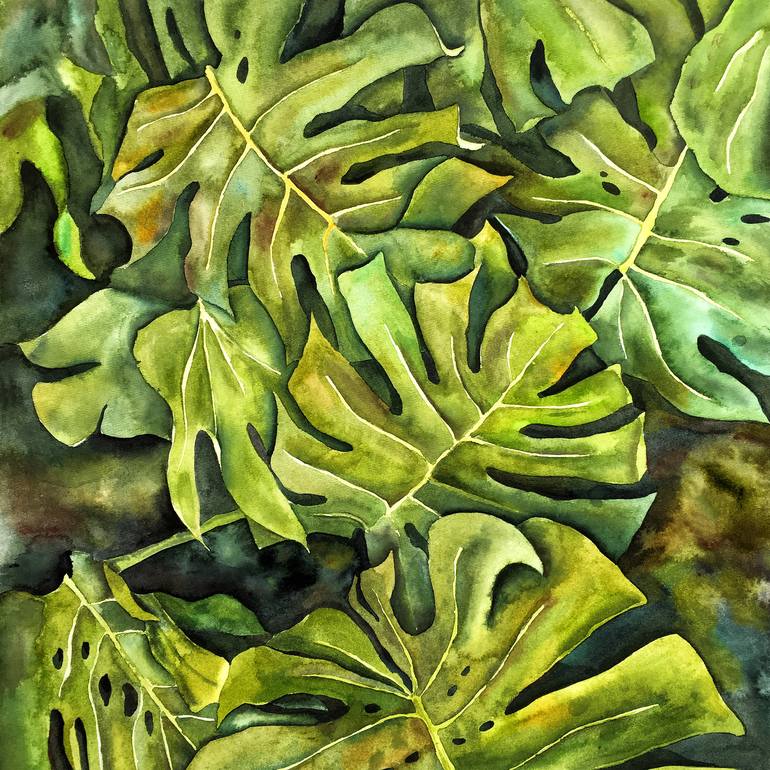
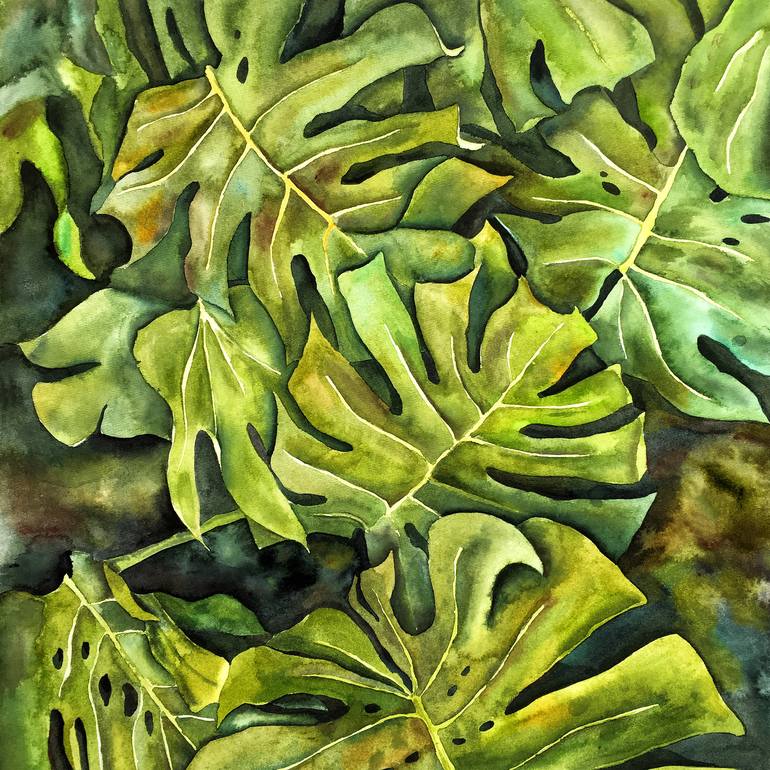
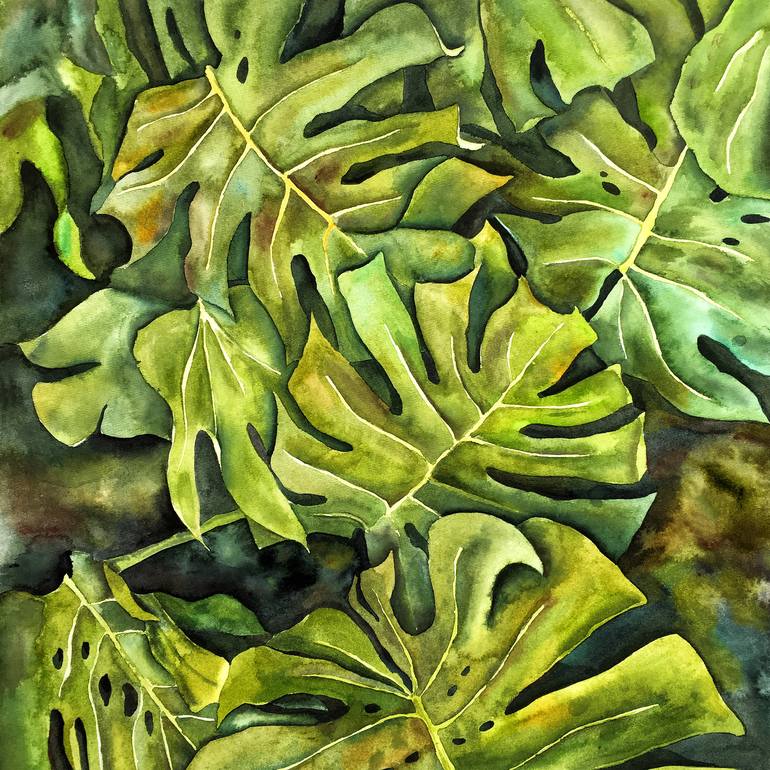
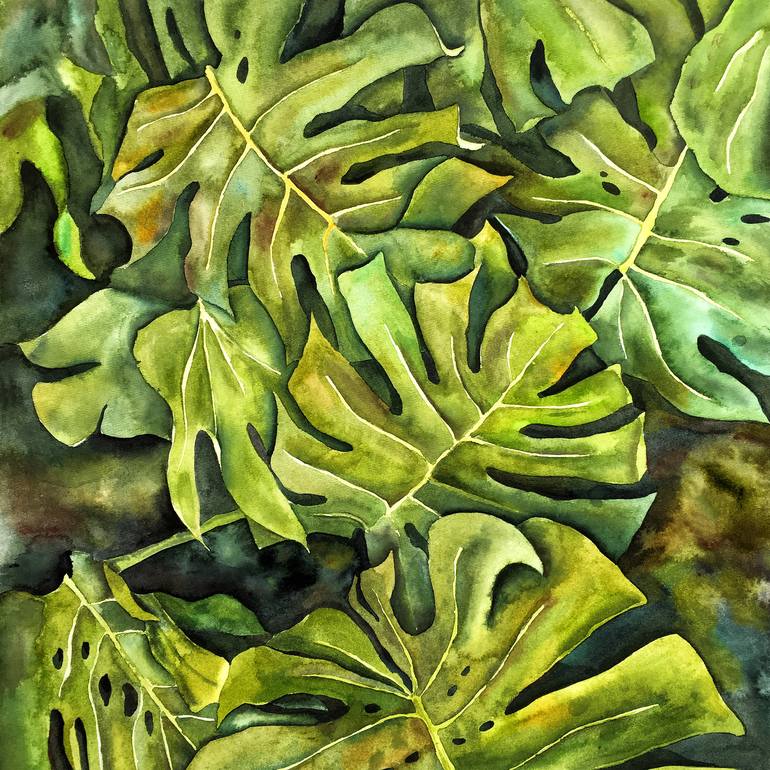
LCP
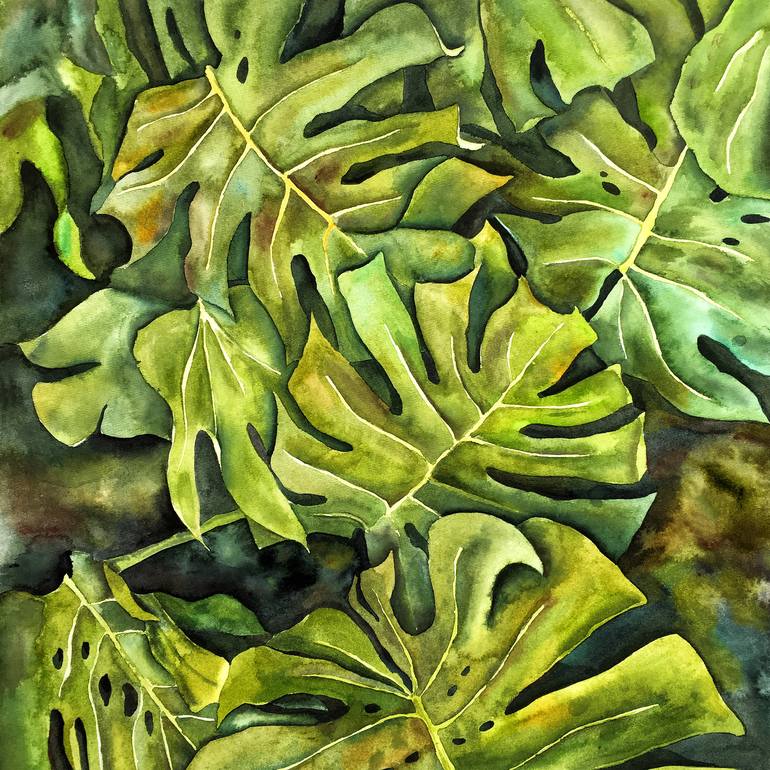
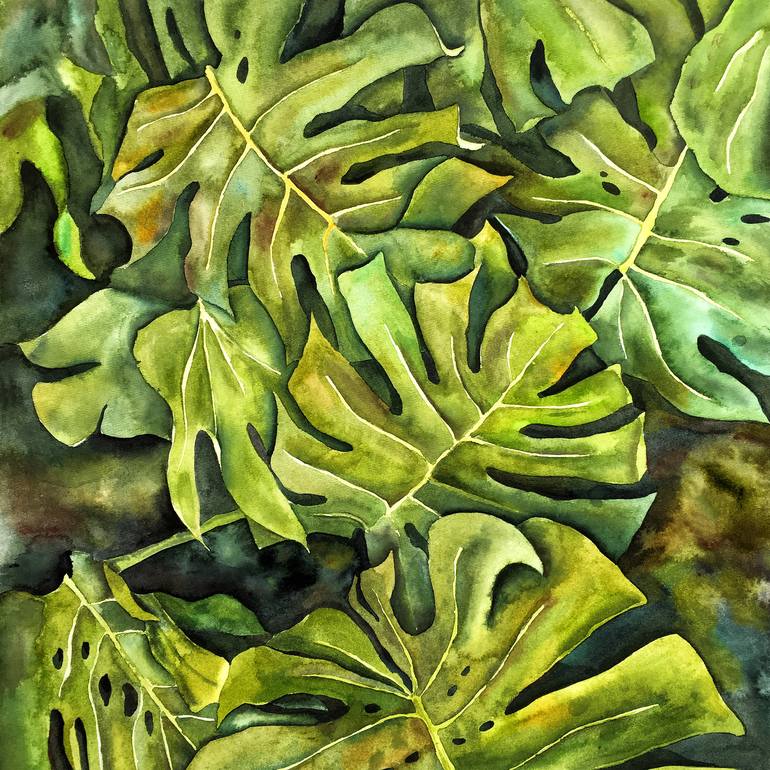
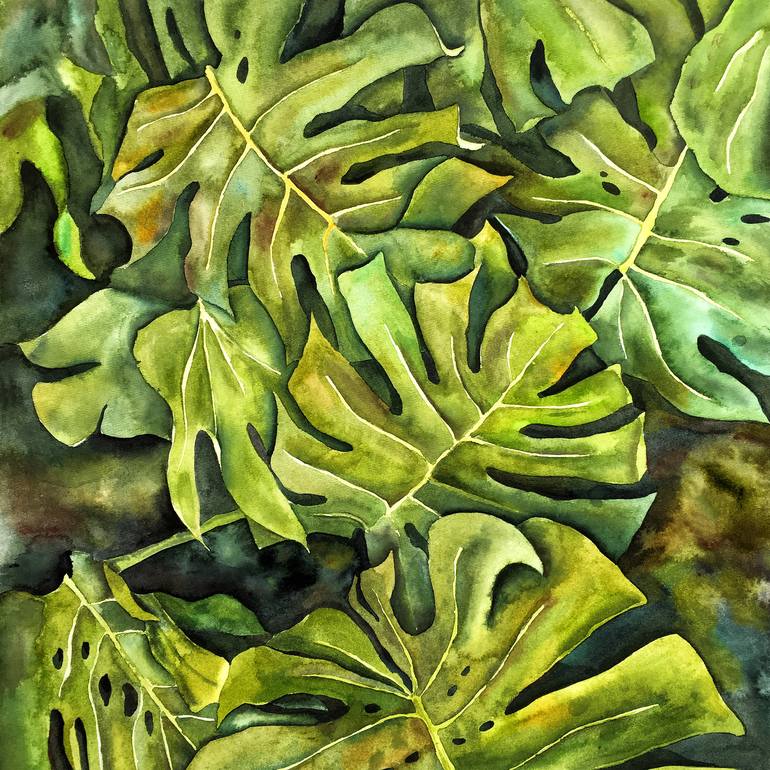
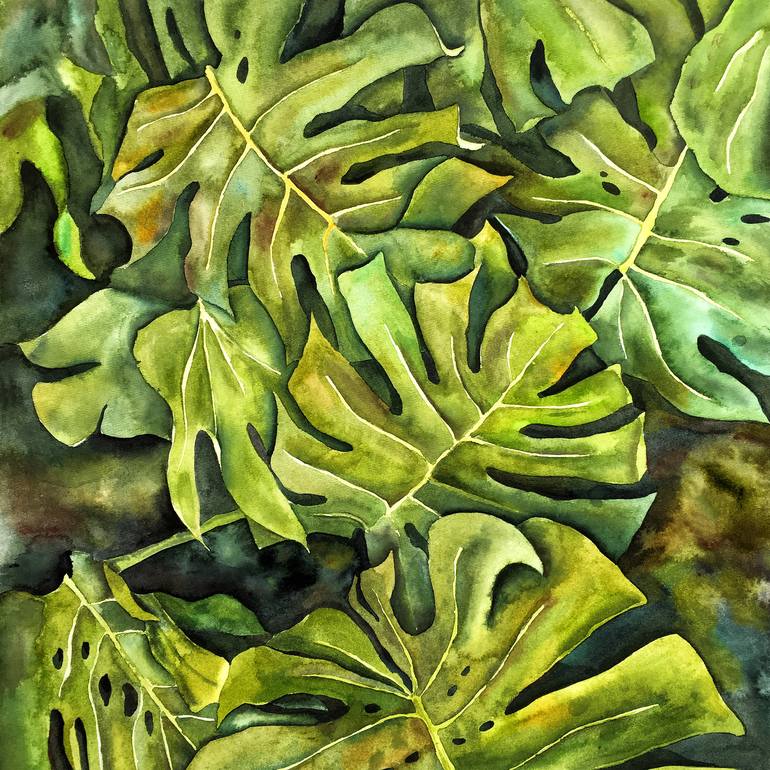
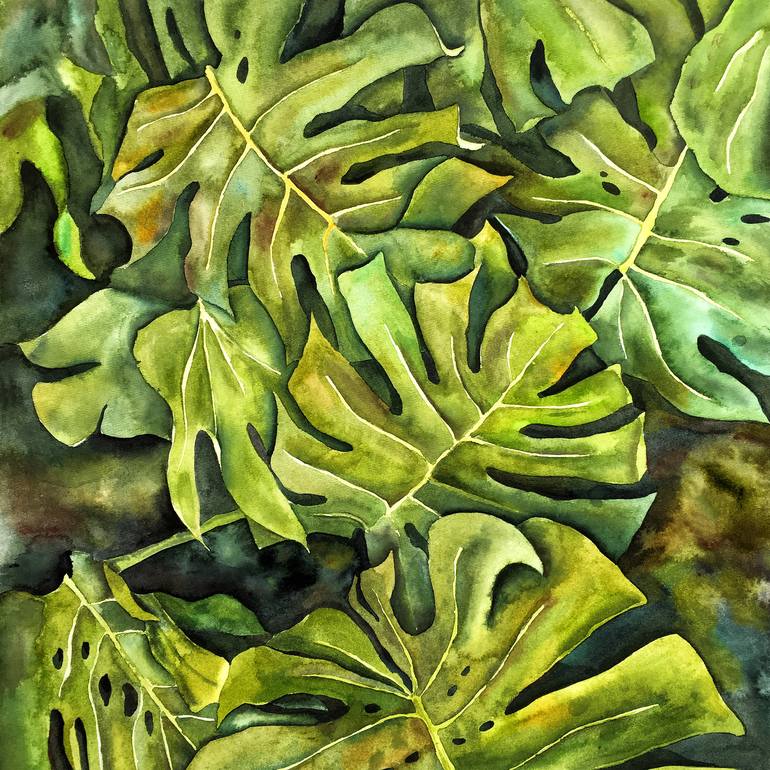
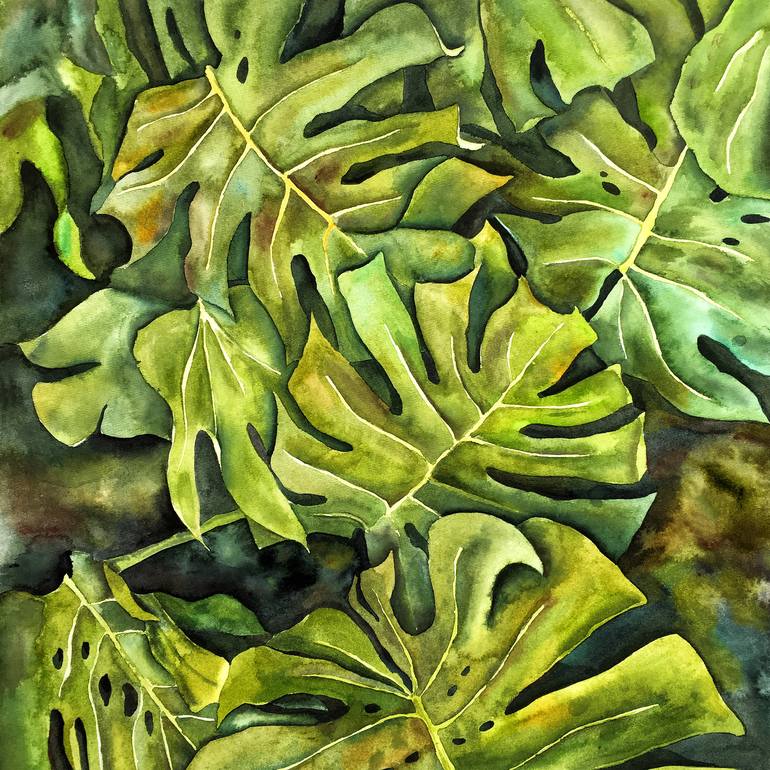
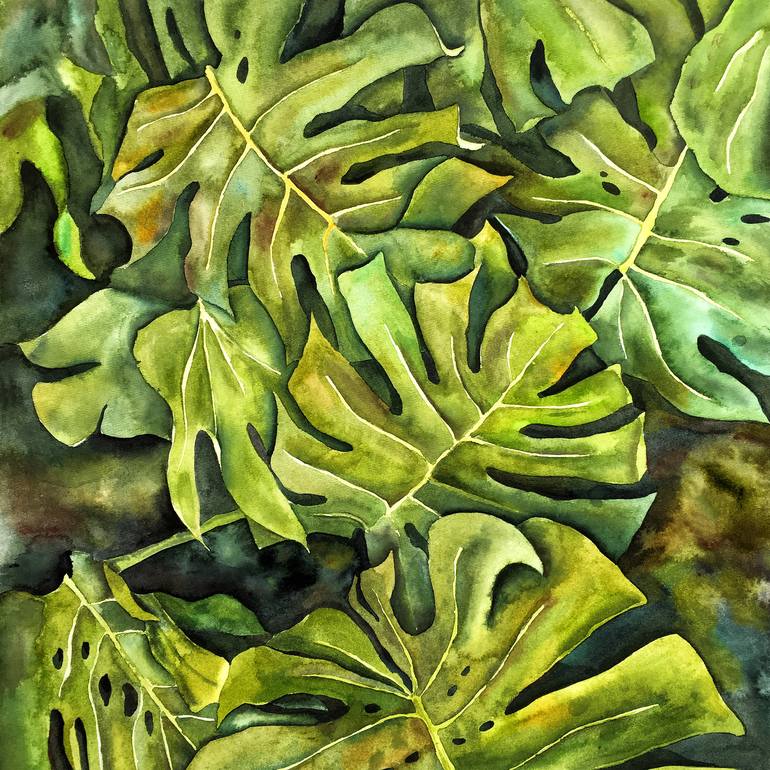
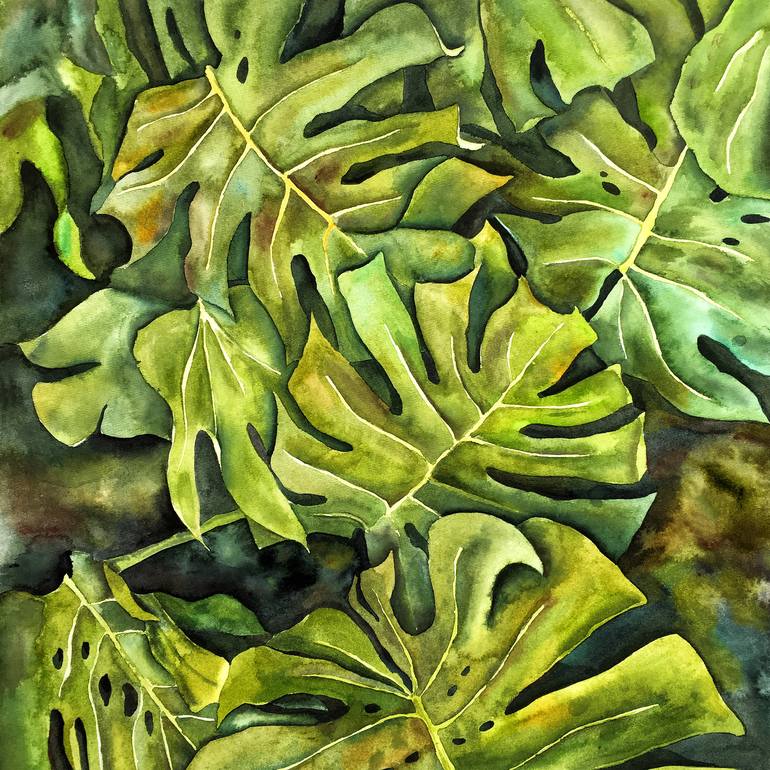
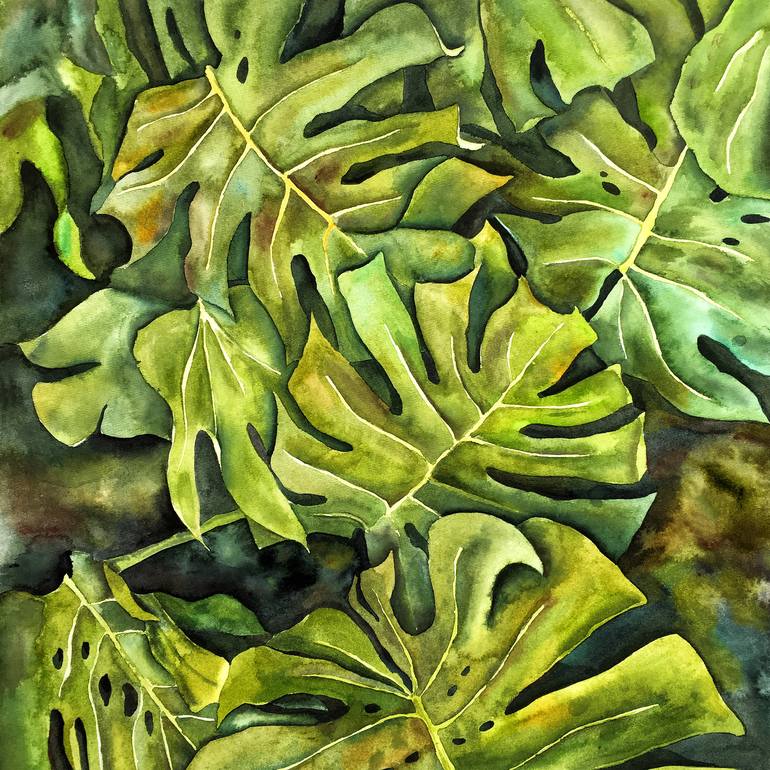
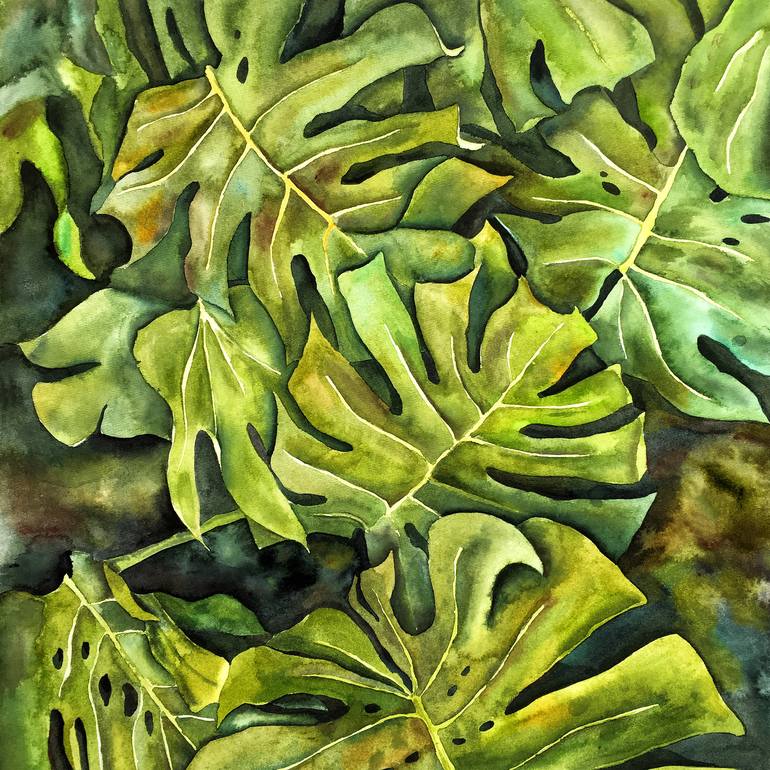
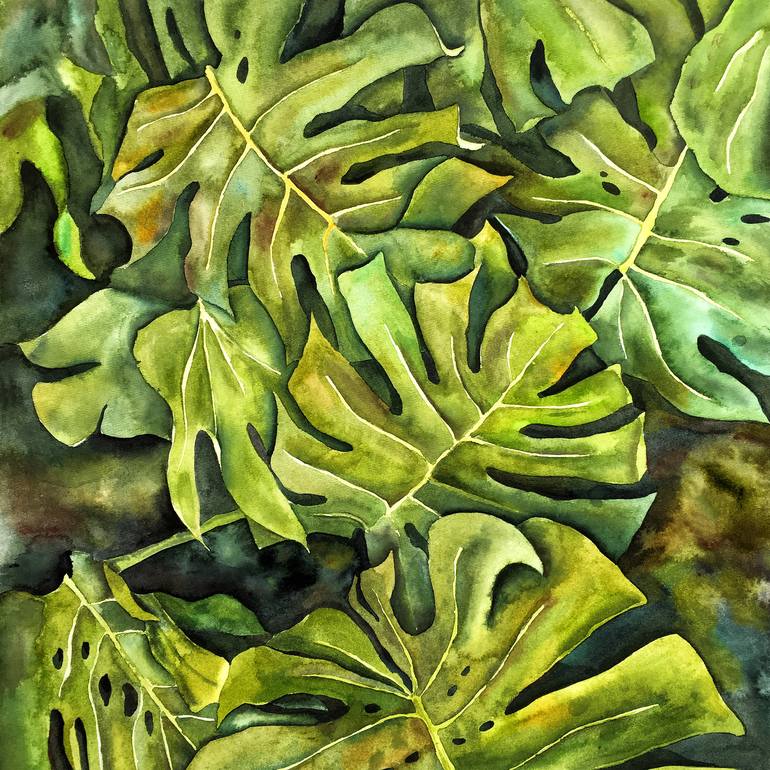
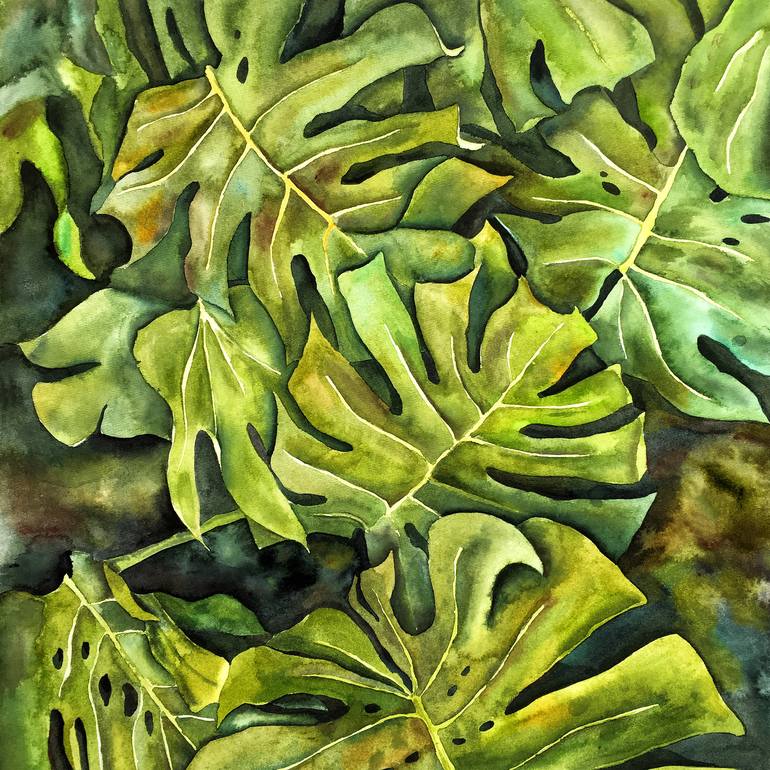
Neurons on amoebae
Machine learning
Machine-learning 2-dimensional amoeba in algebraic geometry and string theory is able to recover the complicated conditions from so-called lopsidedness.
Neurons on Amoebae
We apply methods of machine-learning, such as neural networks, manifold learning and image processing, in order to study 2-dimensional amoebae in algebraic geometry and string theory. With the help of embedding manifold projection, we recover complicated conditions obtained from so-called lopsidedness. For certain cases it could even reach % accuracy, in particular for the lopsided amoeba of with positive coefficients which we place primary focus. Using weights and biases, we also find good approximations to determine the genus for an amoeba at lower computational cost. In general, the models could easily predict the genus with over 90% accuracies. With similar techniques, we also investigate the membership problem, and image processing of the amoebae directly.