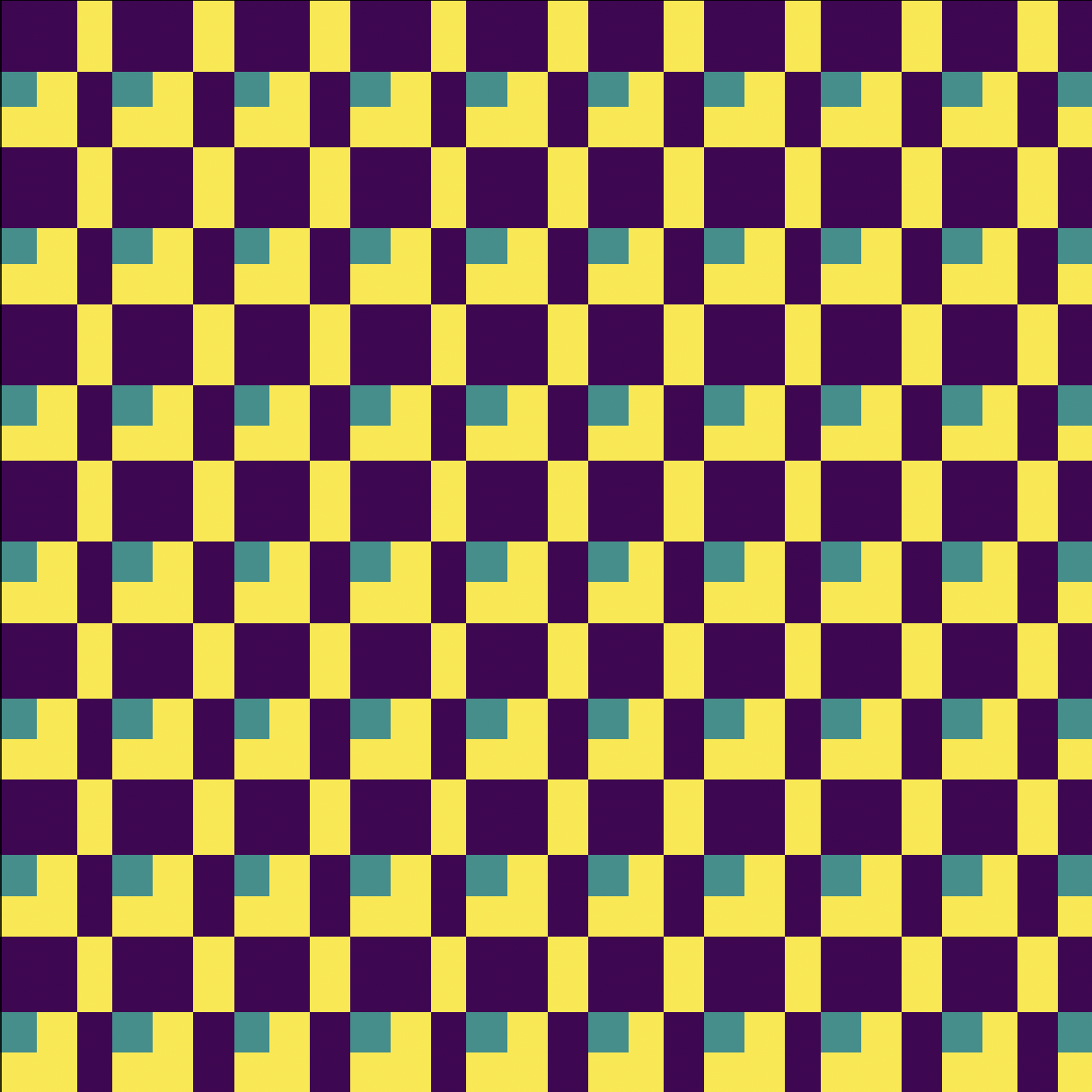
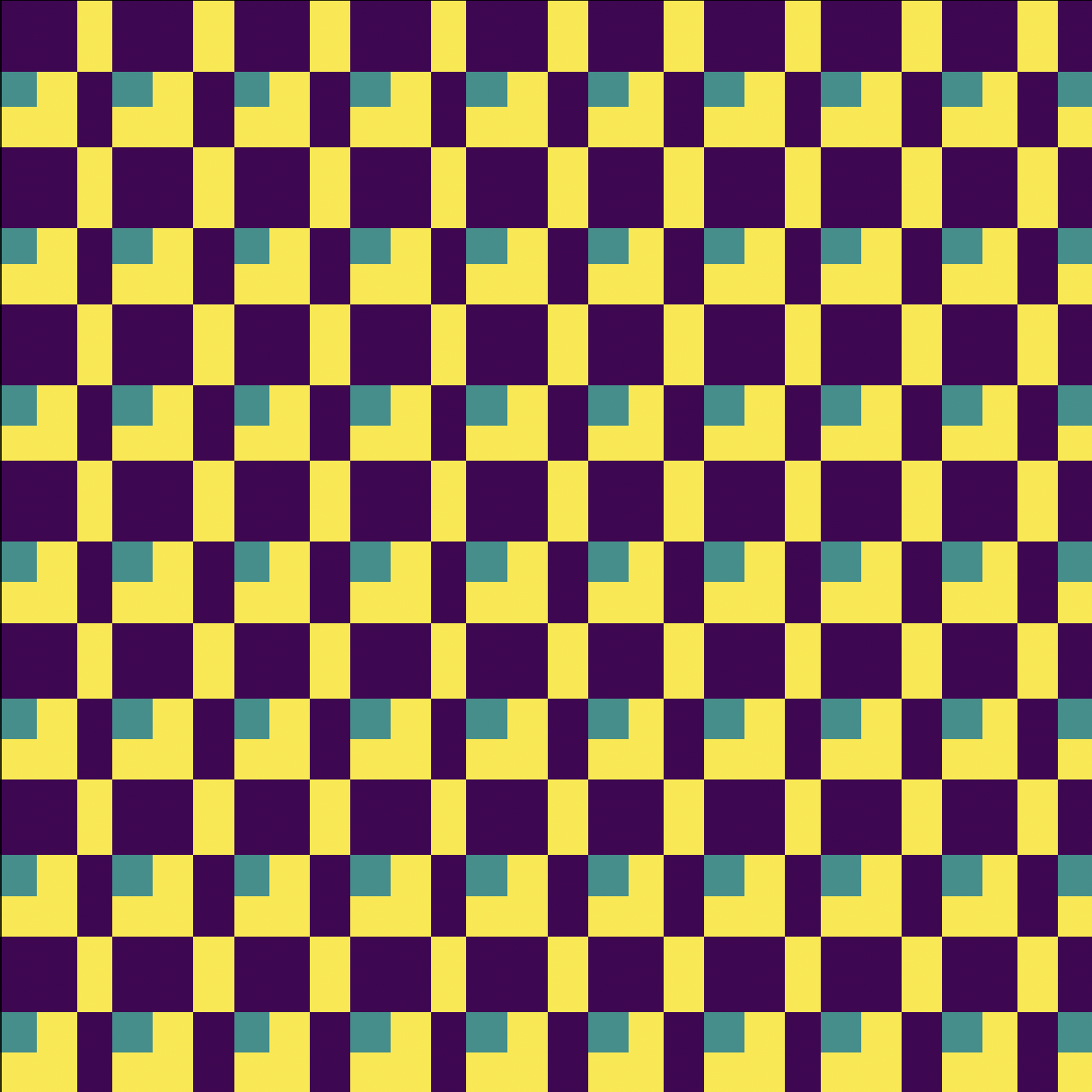
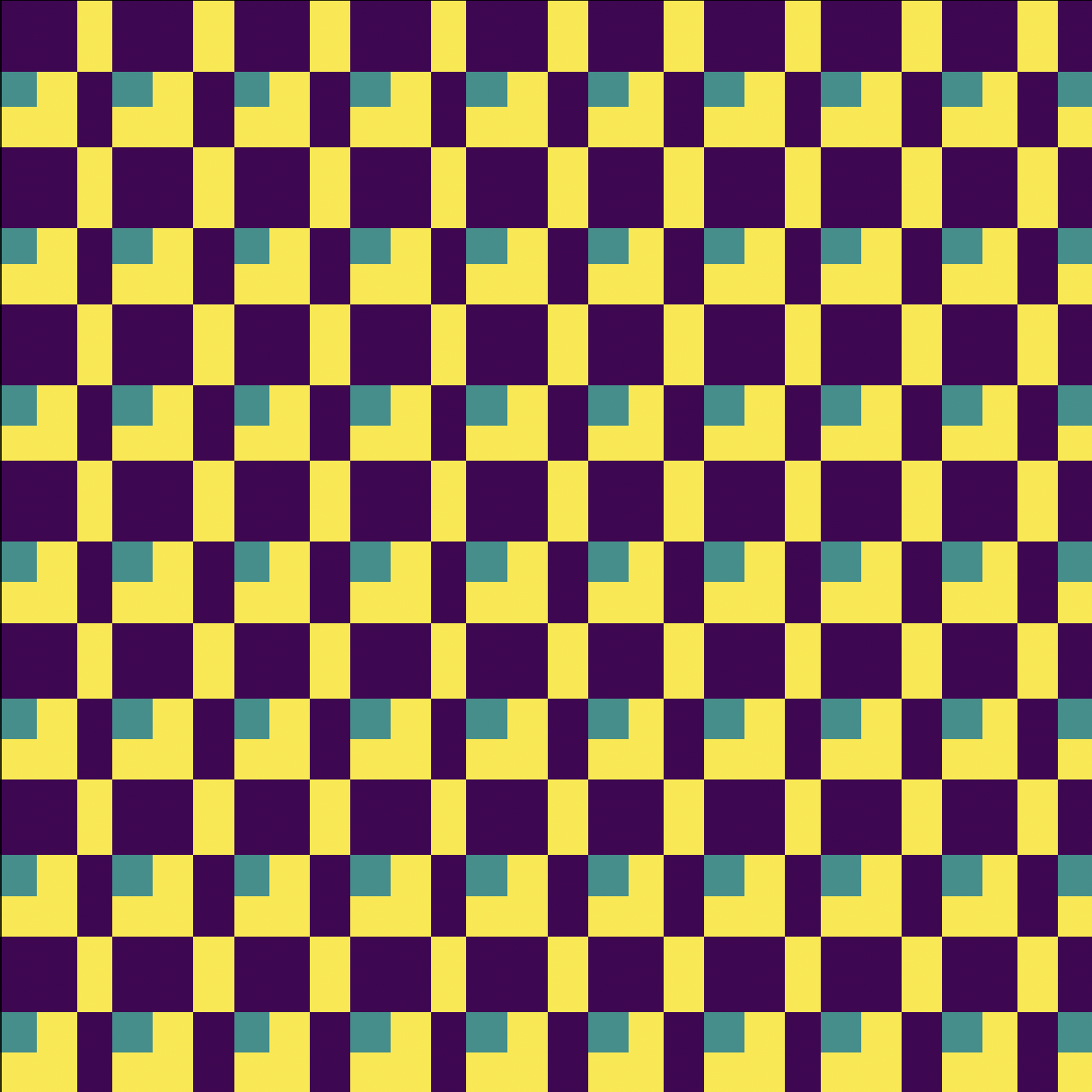
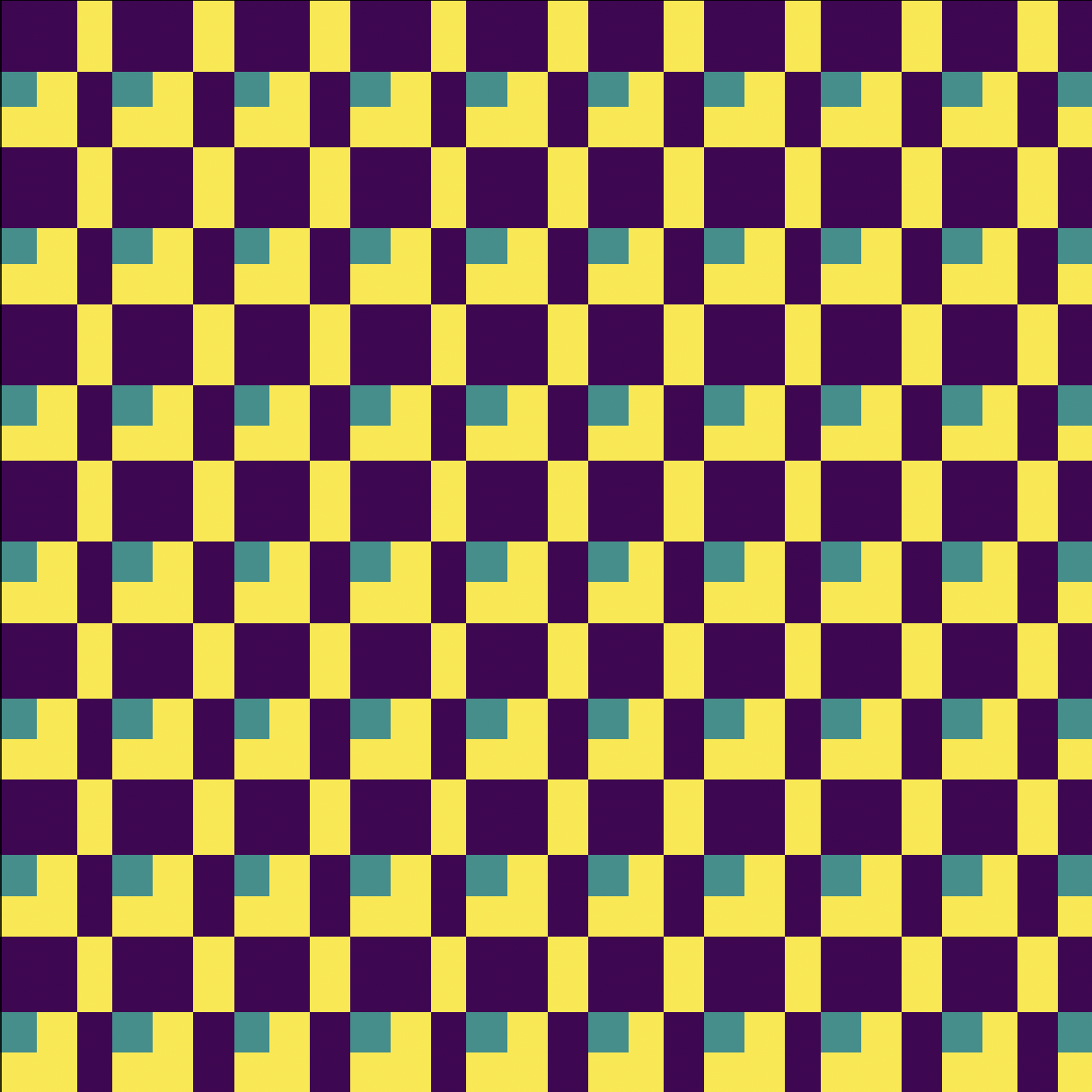
LCP
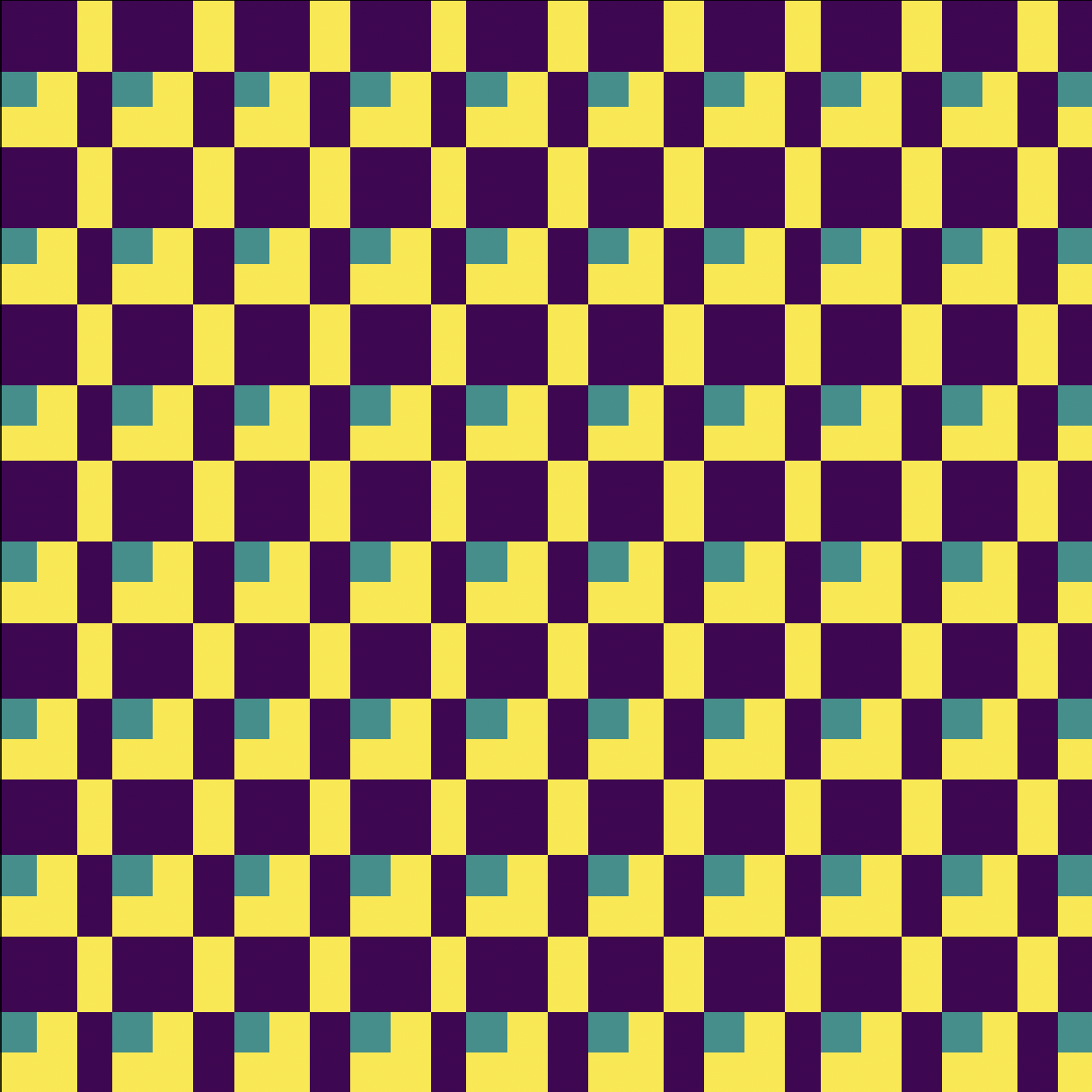
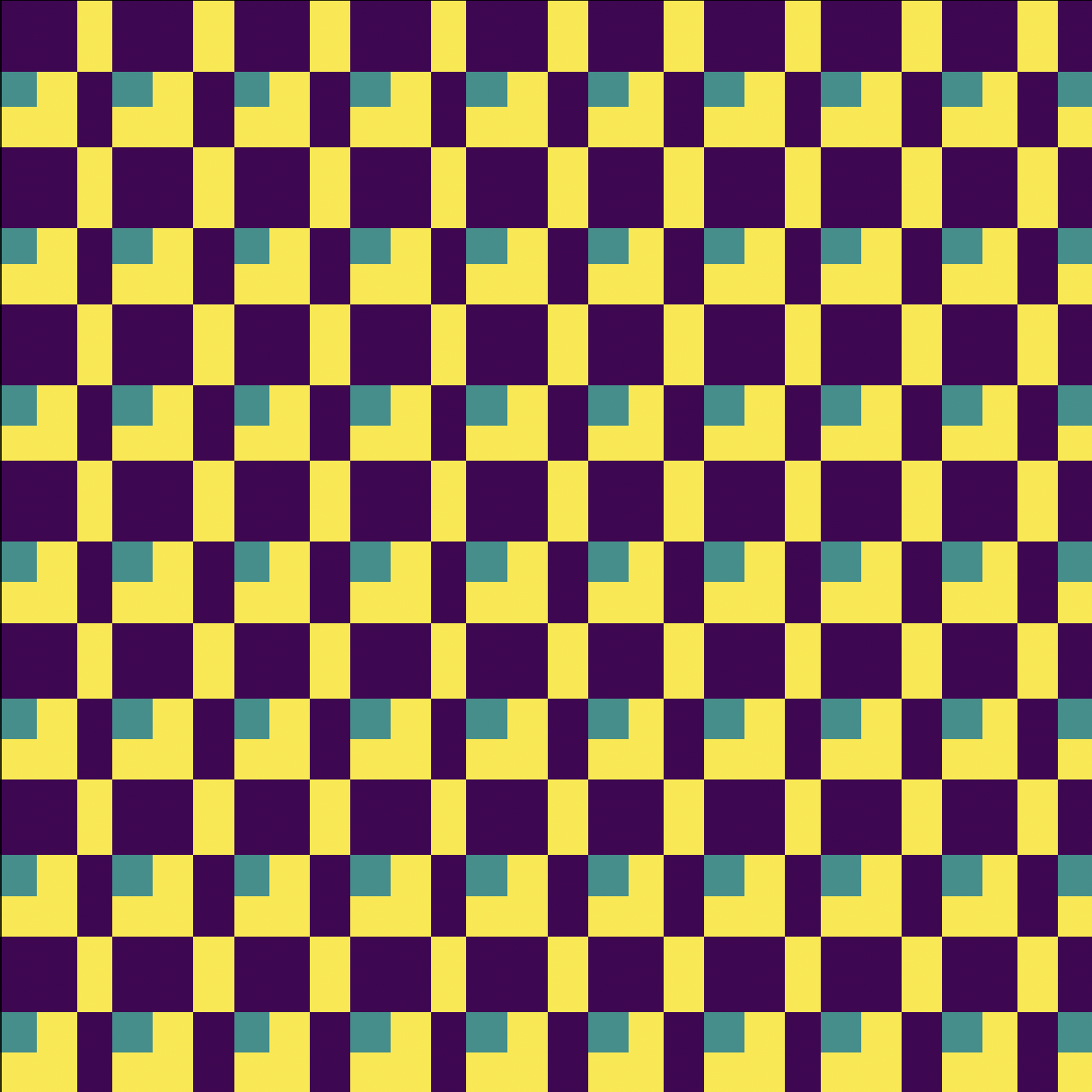
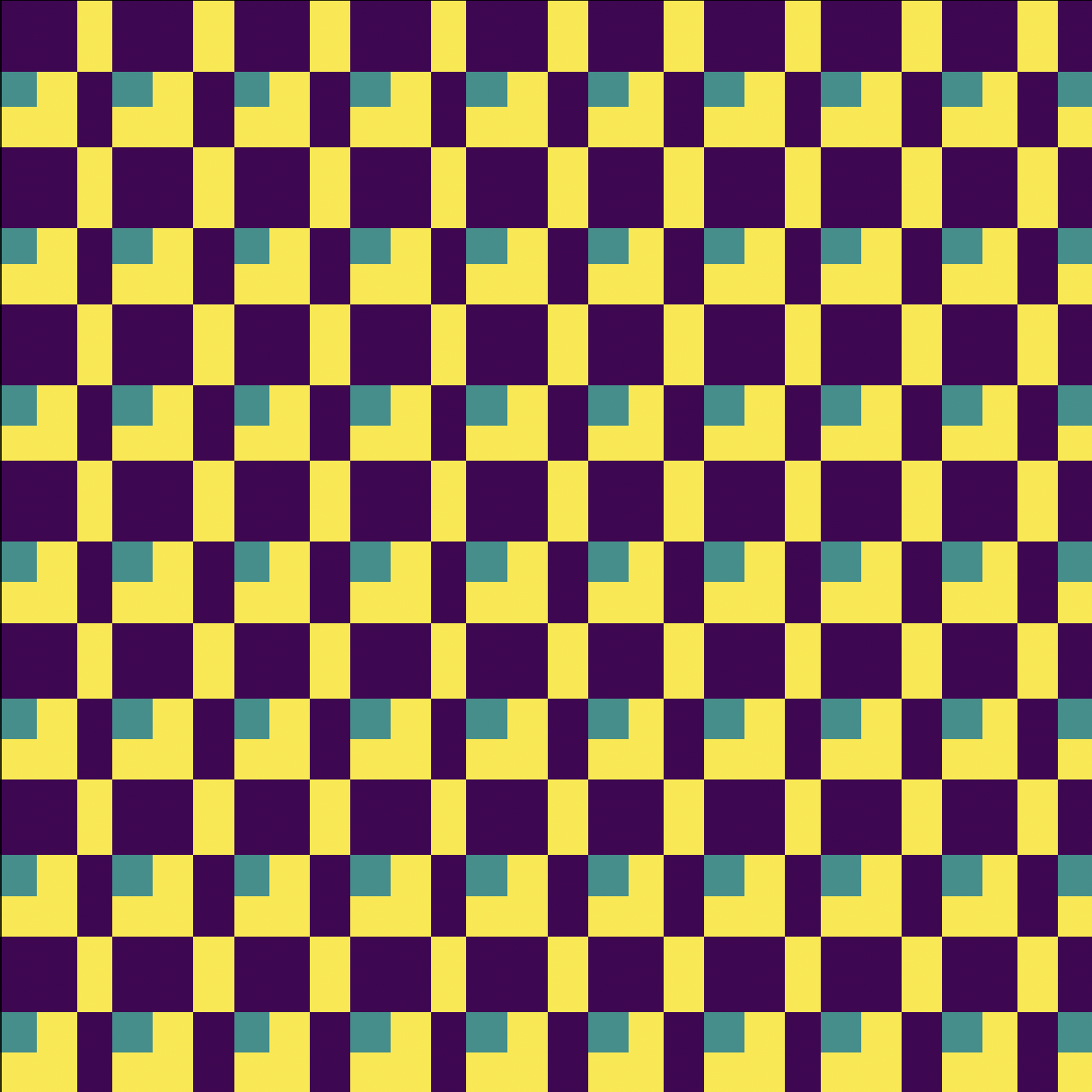
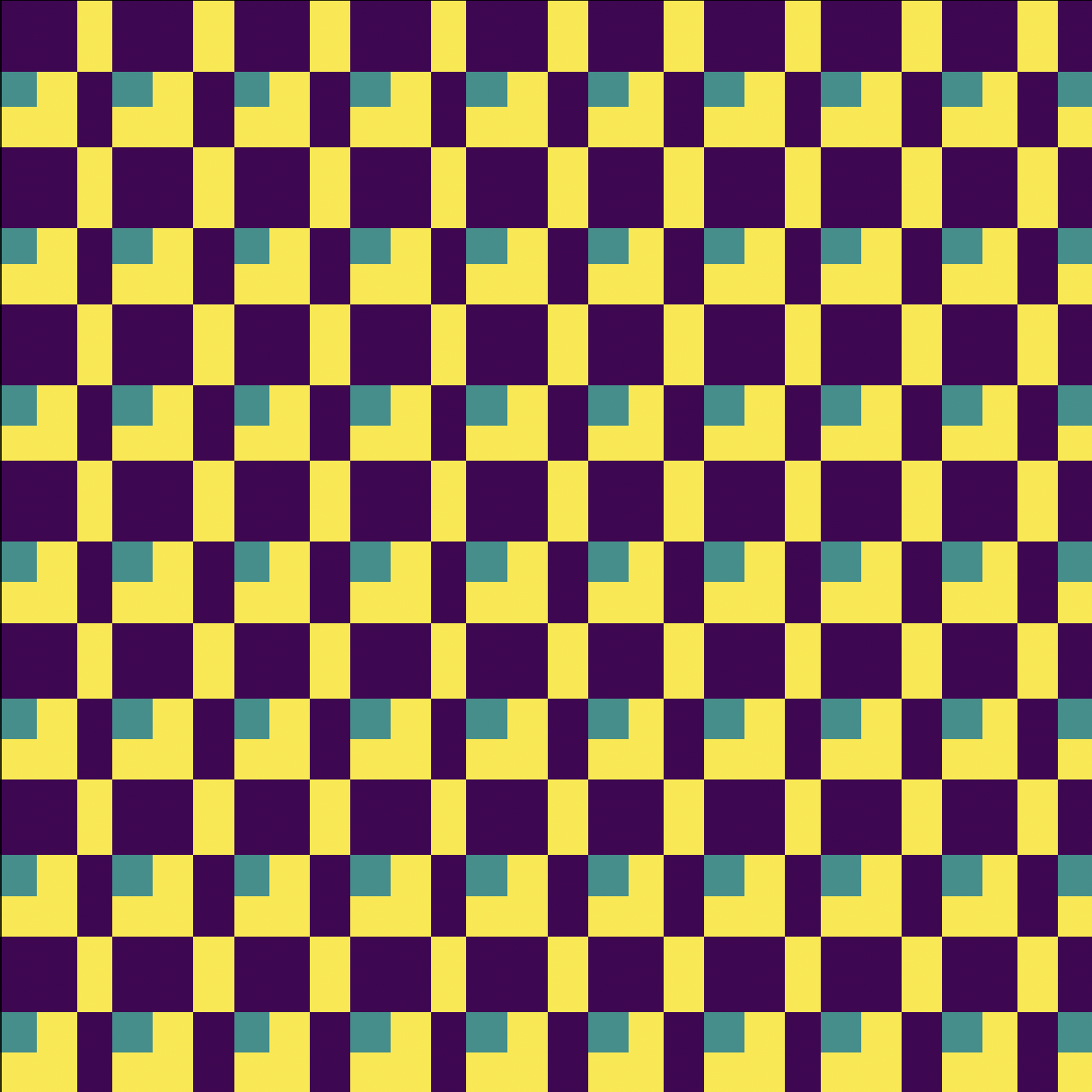
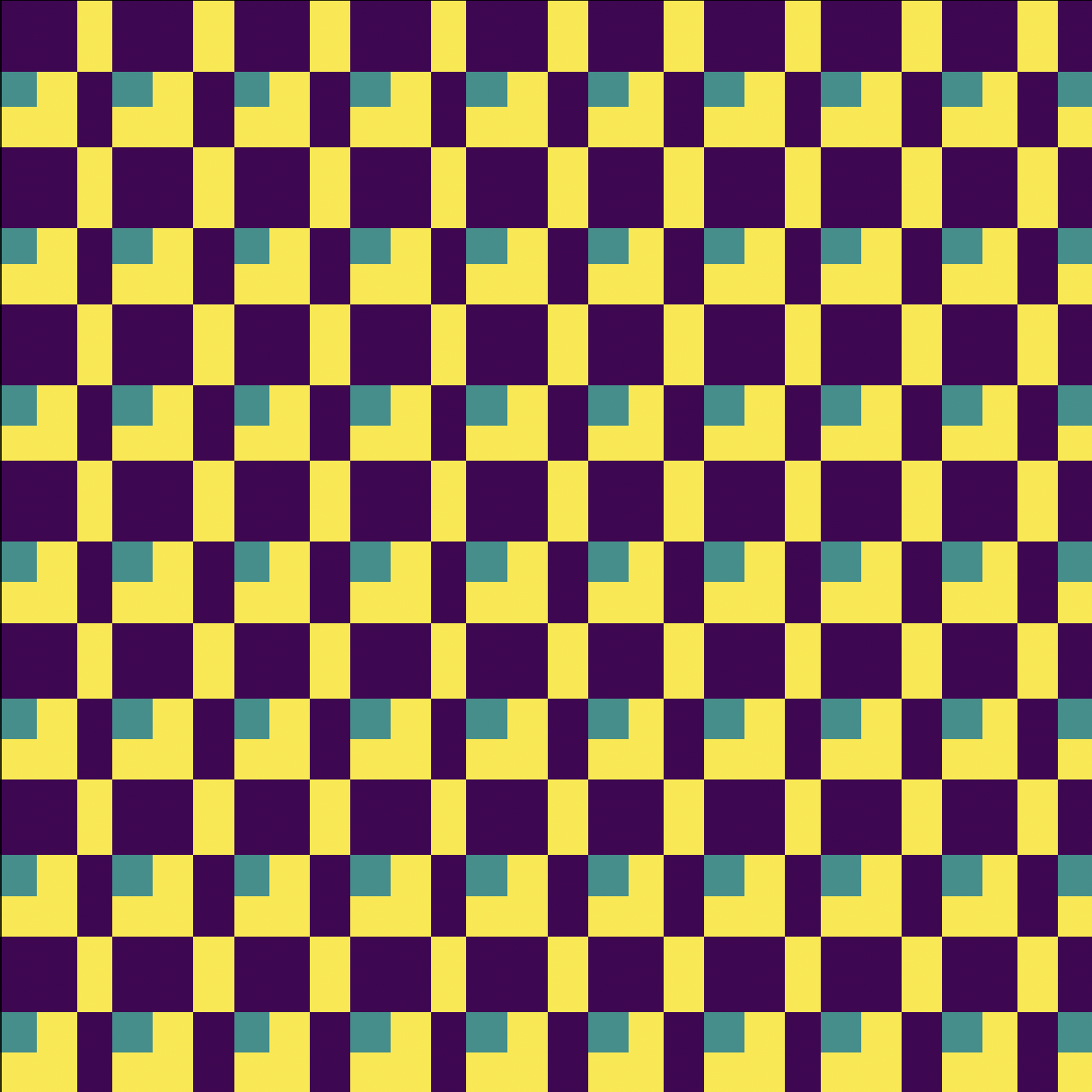
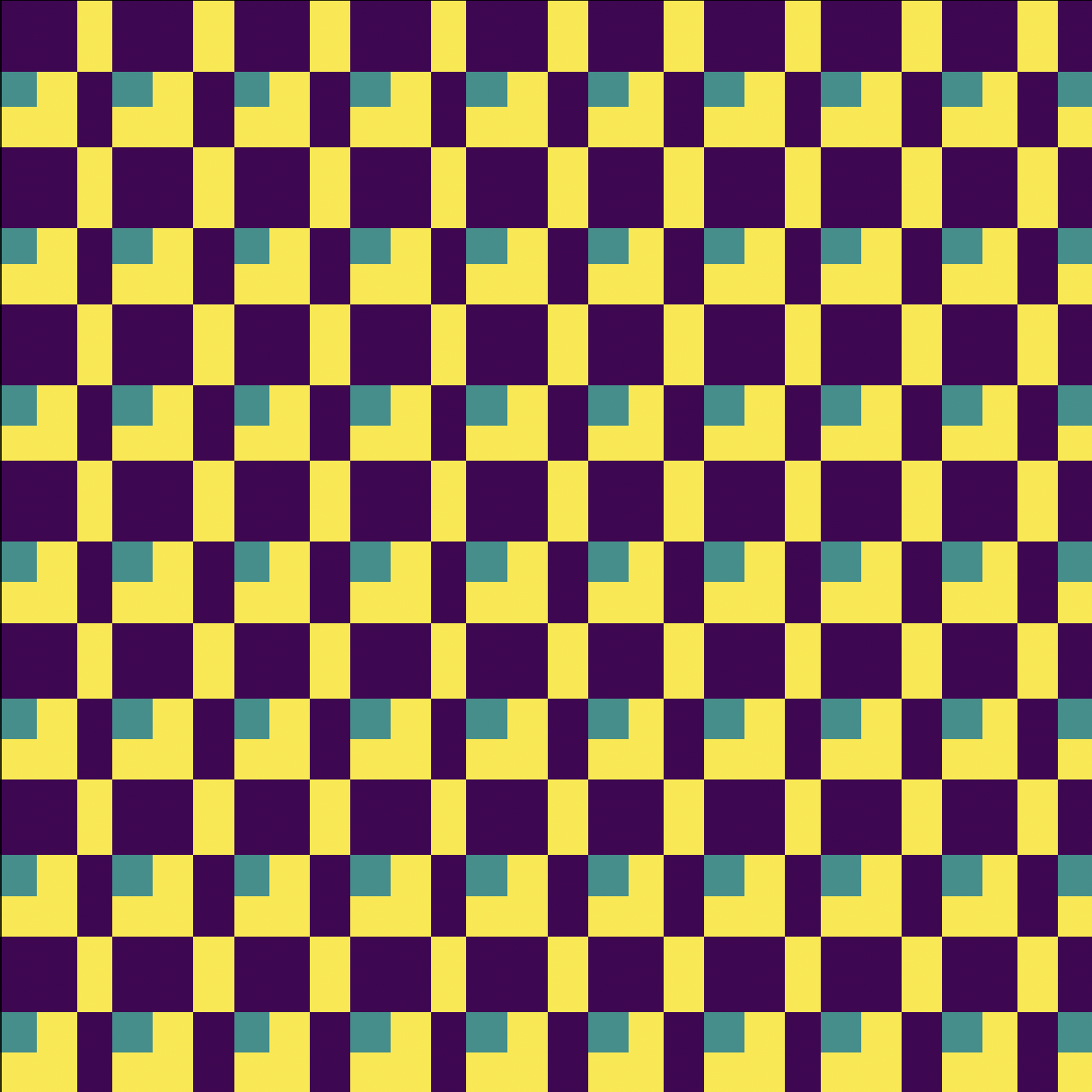
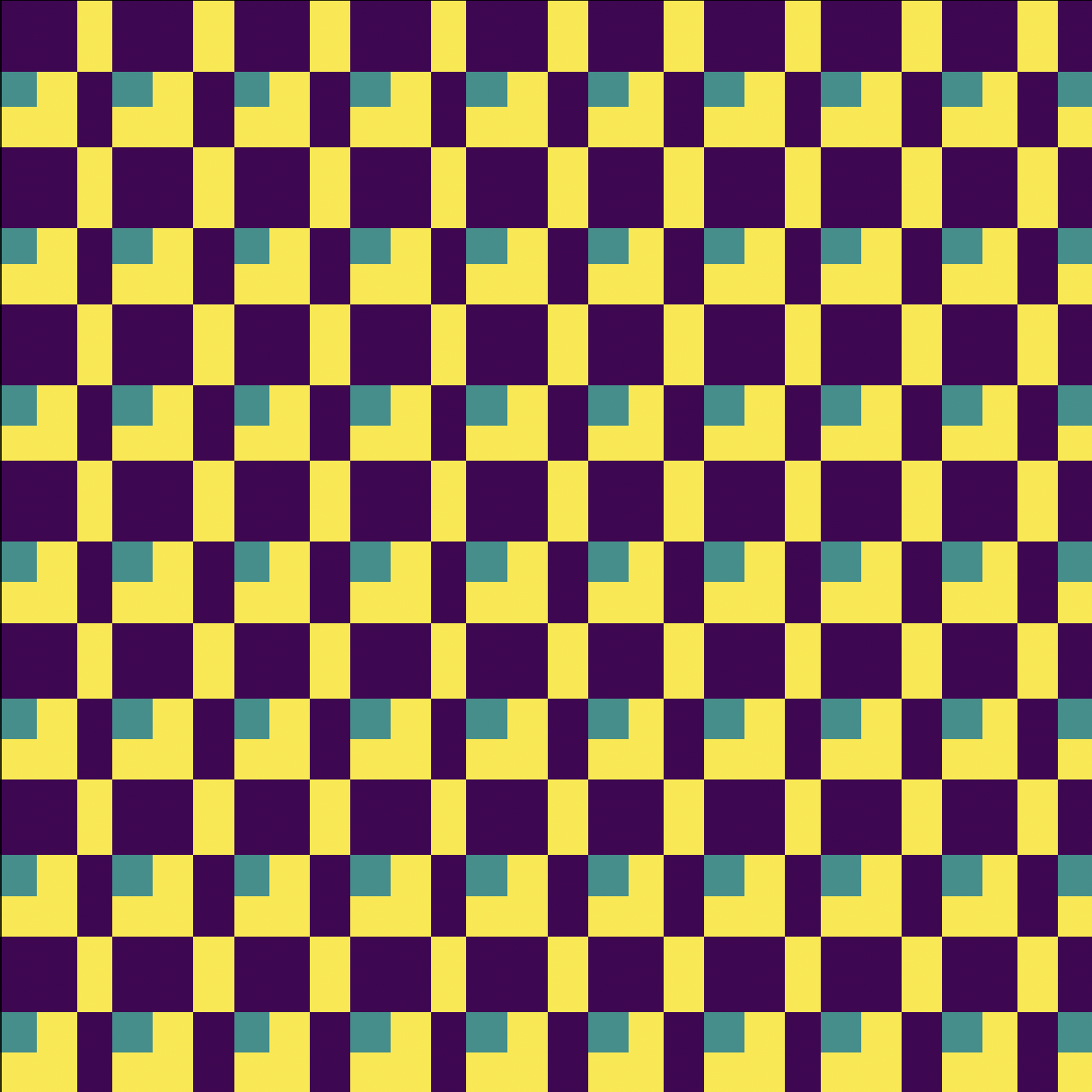
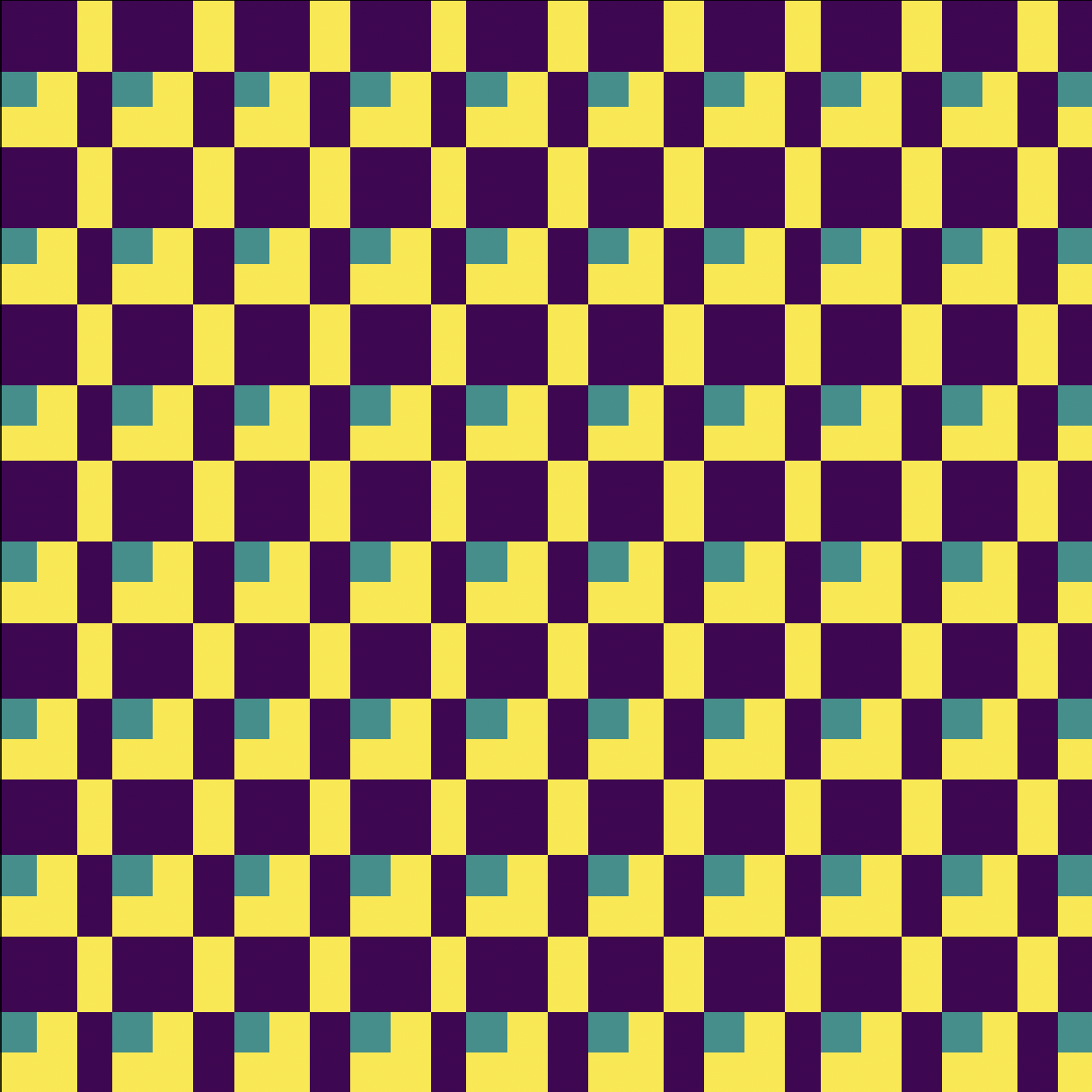
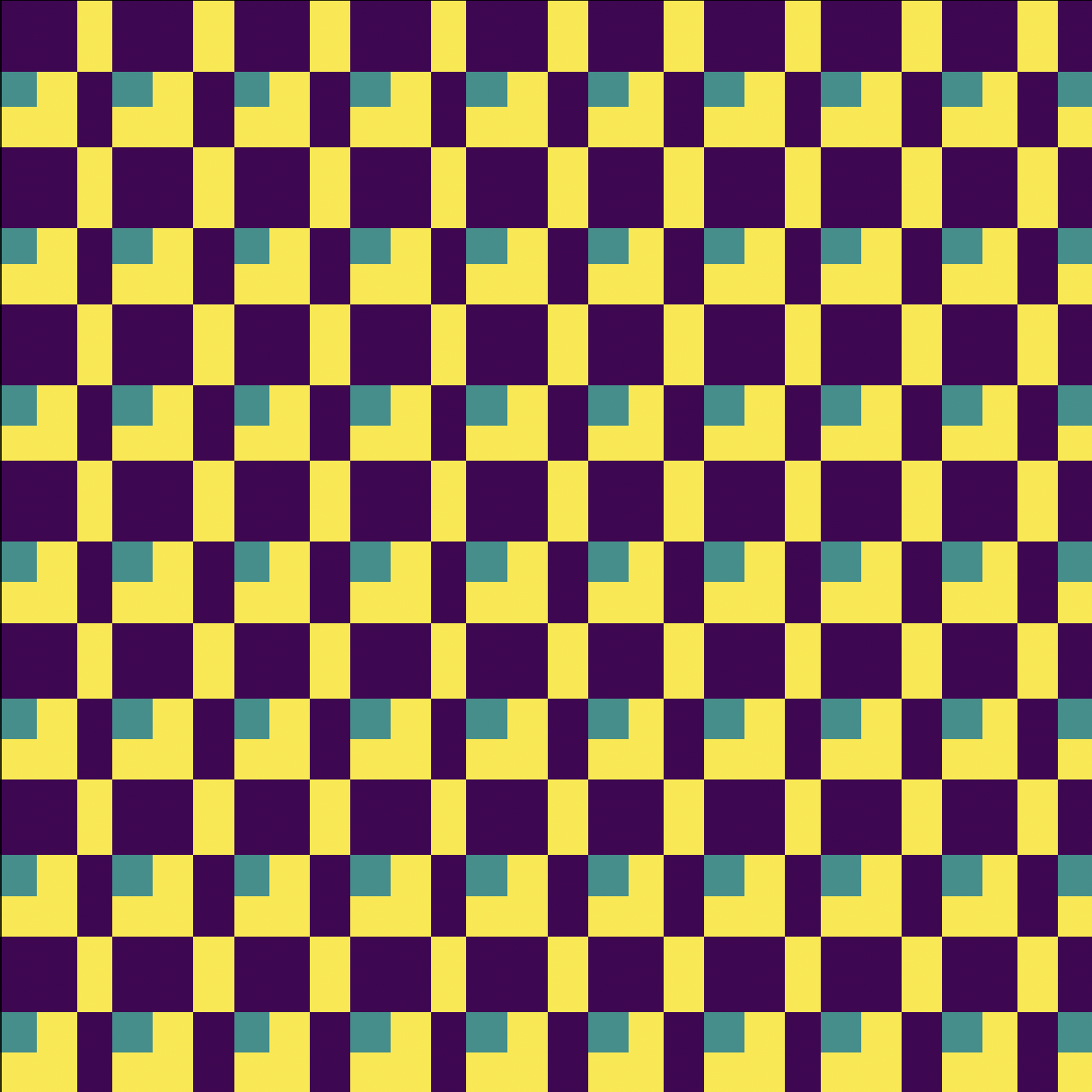
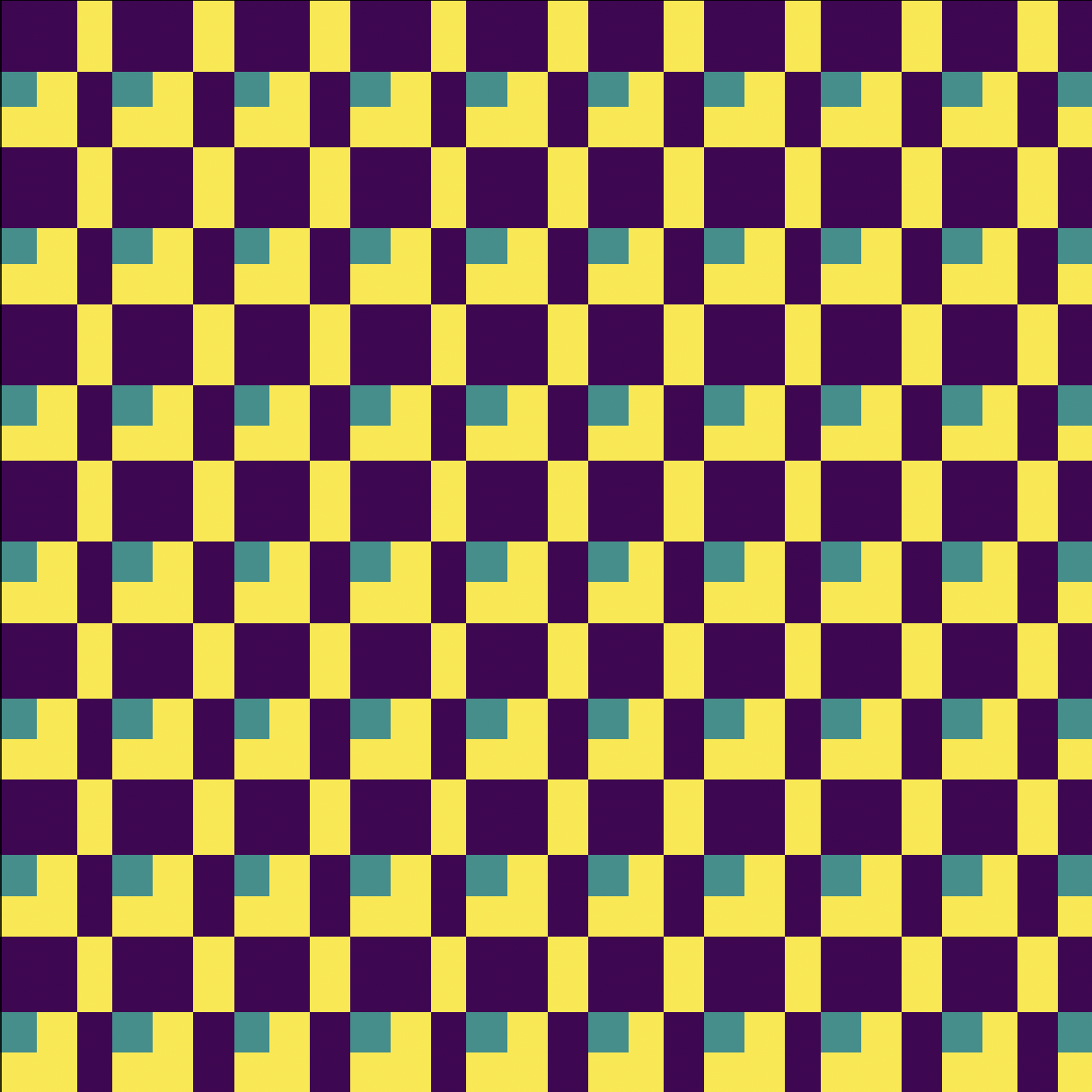
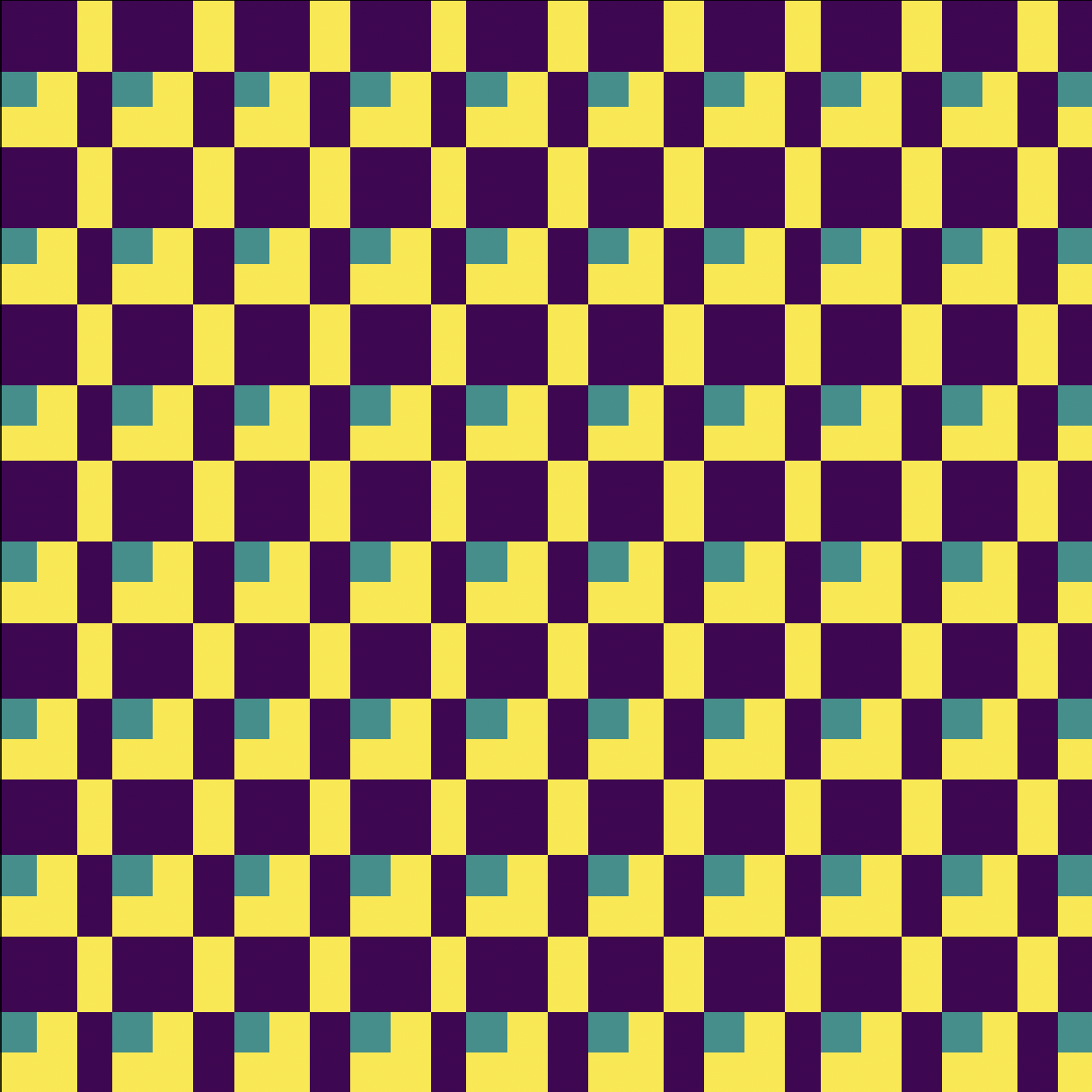
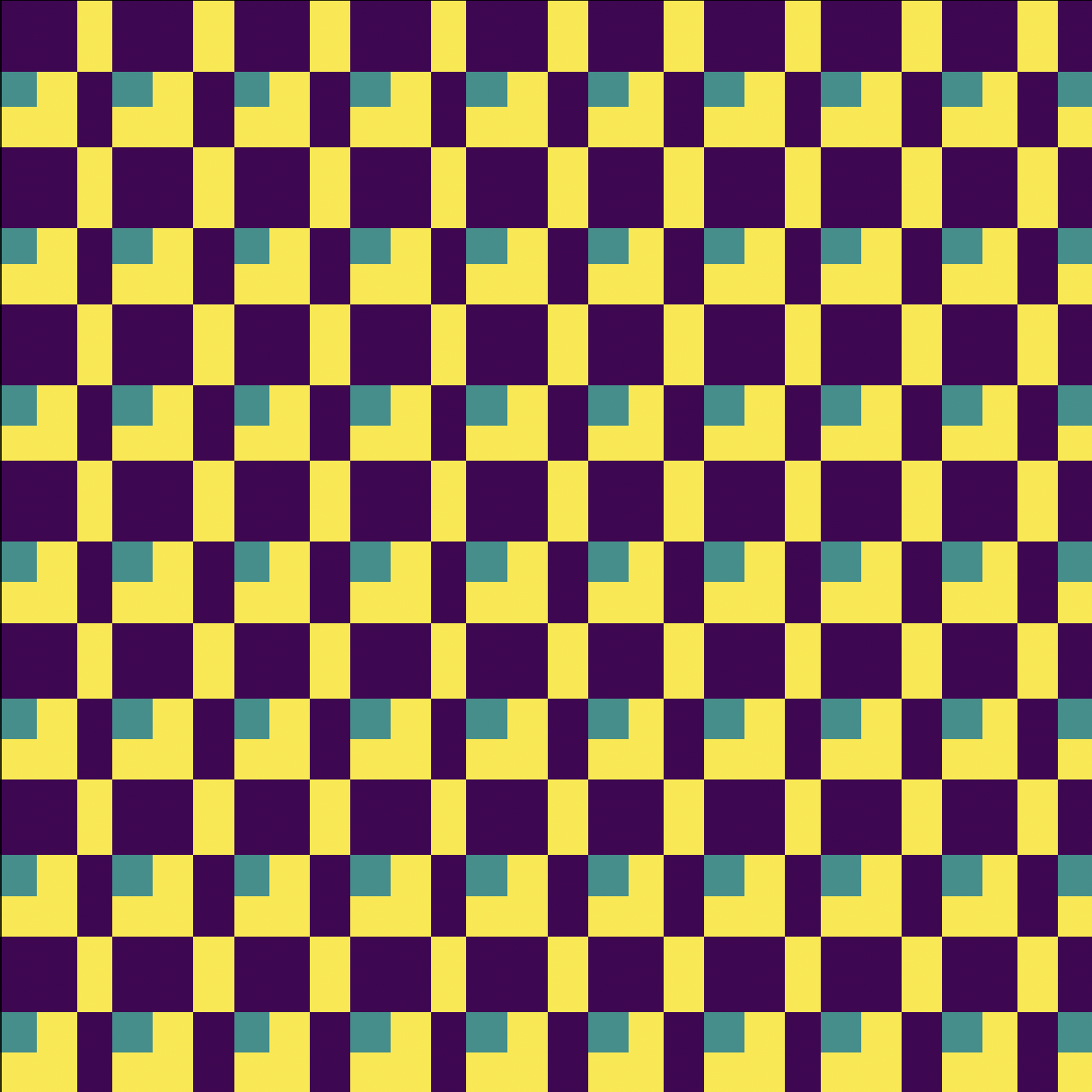
World in a grain of sand
String theory
An AI algorithm of few-shot learning finds that the vast string landscape could be reduced by only seeing a tiny fraction to predict the rest.
The world in a grain of sand: condensing the string vacuum degeneracy
The proliferation of vacuum solutions to string theory highly deter the search for the Standard Model. We propose a novel approach to this problem by finding an efficient measure of similarity of vacua. Using one million concrete examples, the paradigm of few-shot machine-learning represents them as points in Euclidean three-space with similar points clustered together. We thereby compress the search space for desired physics to within one percent of the original. Our analysis provides an explicit method for machine-learning the landscape and finding ‘typicality’, even with minuscule data.