DANGER: Data, Numbers and Geometry
11 AM, 8 Aug 2024 – 9 Aug 2024
The London Institute hosts a two-day workshop for theorists to discuss and explore the links between data science, AI and pure mathematics.
Conjectures can inspire new branches of pure mathematics and theoretical physics. They usually come from spotting patterns and applying instinct. Recently, there has been a surge of interest in using automated pattern detection to help humans form conjectures. Because in mathematics there are no coincidences, mathematical data is immune from the false positives and false negatives that plague physical measurement.
In this two-day workshop, the London Institute brings together physicists and mathematics to explore how AI can speed up theoretical research. The topics addressed range from geometry to string theory to representation theory.
This is the fourth in the series of annual DANGER workshops (Data, Numbers, Geometry and Representation theory). The series was created by Alexander Kasprzyk from Nottingham University, Thomas Oliver from Westminster University, and Yang-Hui He from the London Institute. This is a hybrid workshop. Those unable to attend in person can join online via Zoom.
Event info
This workshop takes place on Thursday 24 and Friday 25 August at the London Institute for Mathematical Sciences, which is on the second floor of the Royal Institution in Mayfair. To register to attend this workshop, please visit the conference website.
Programme
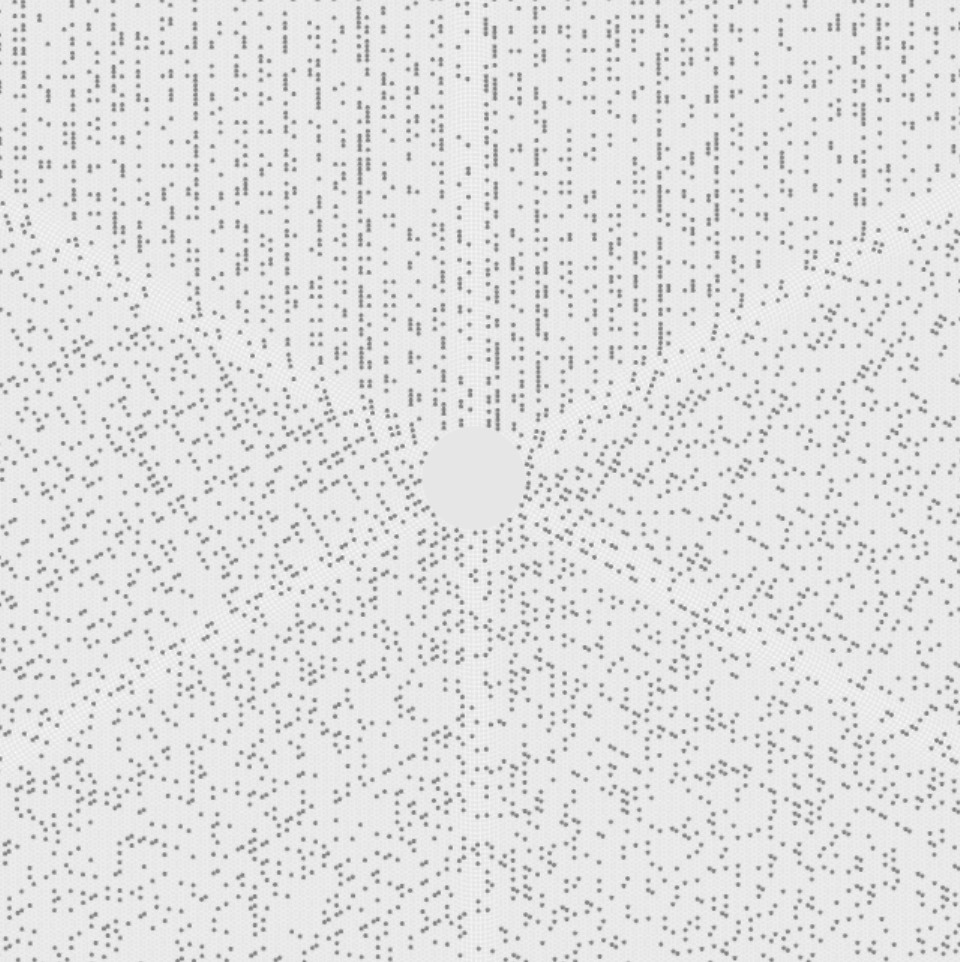
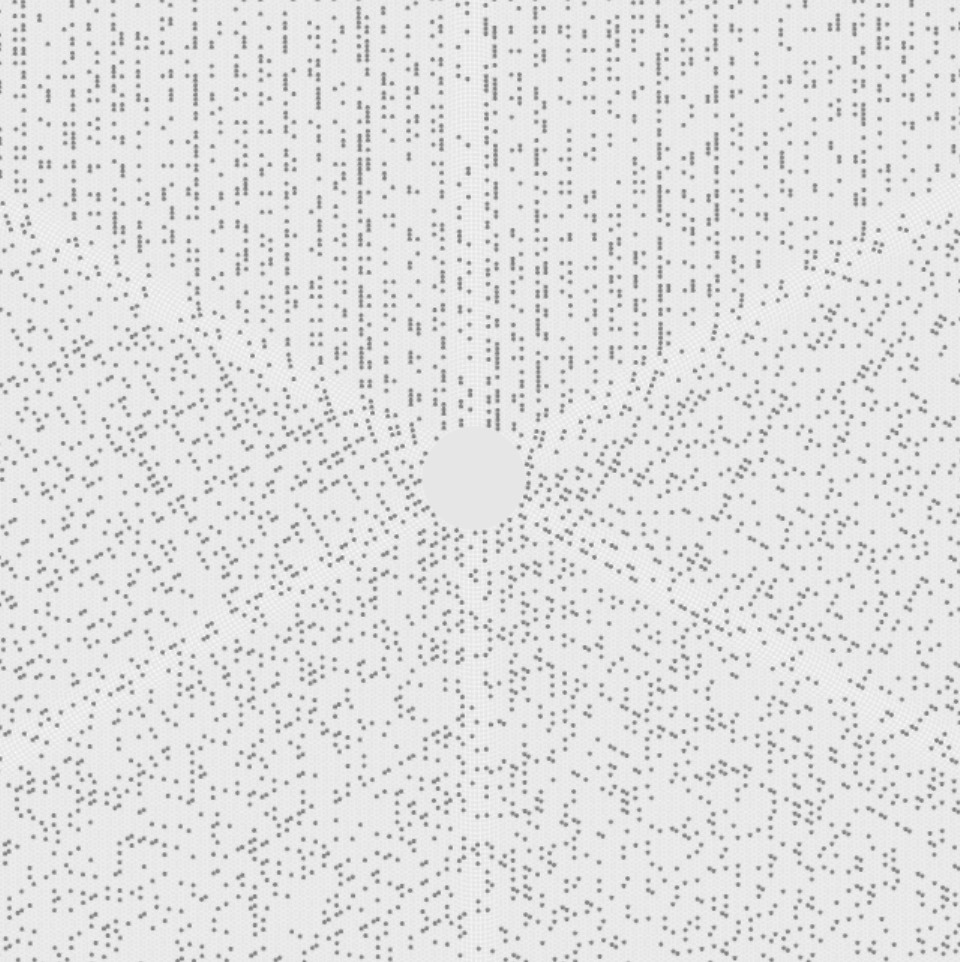
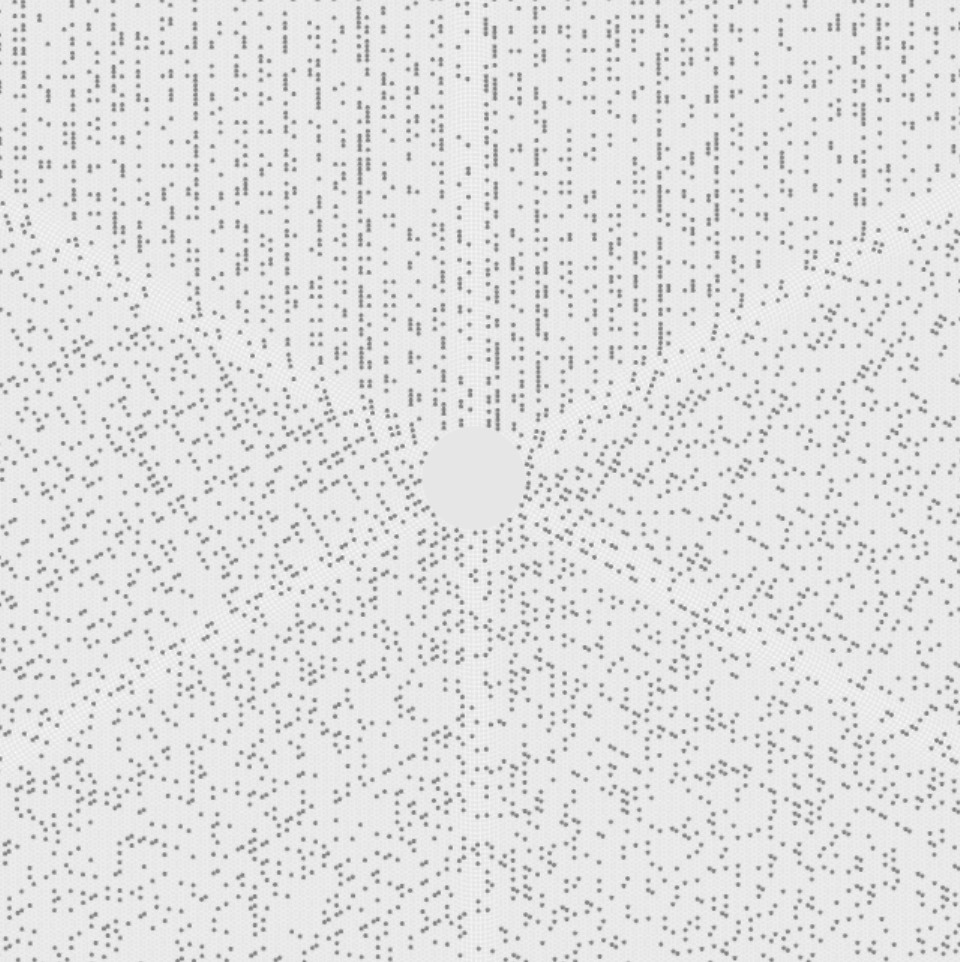
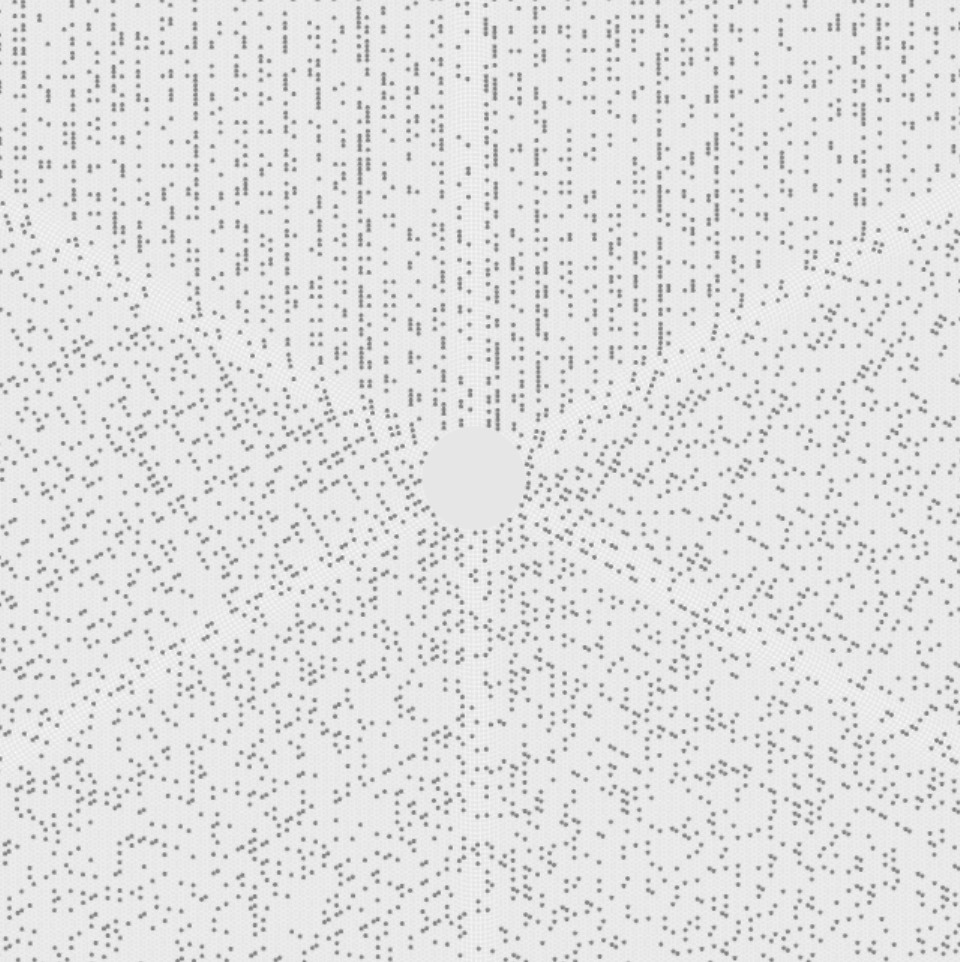
LCP
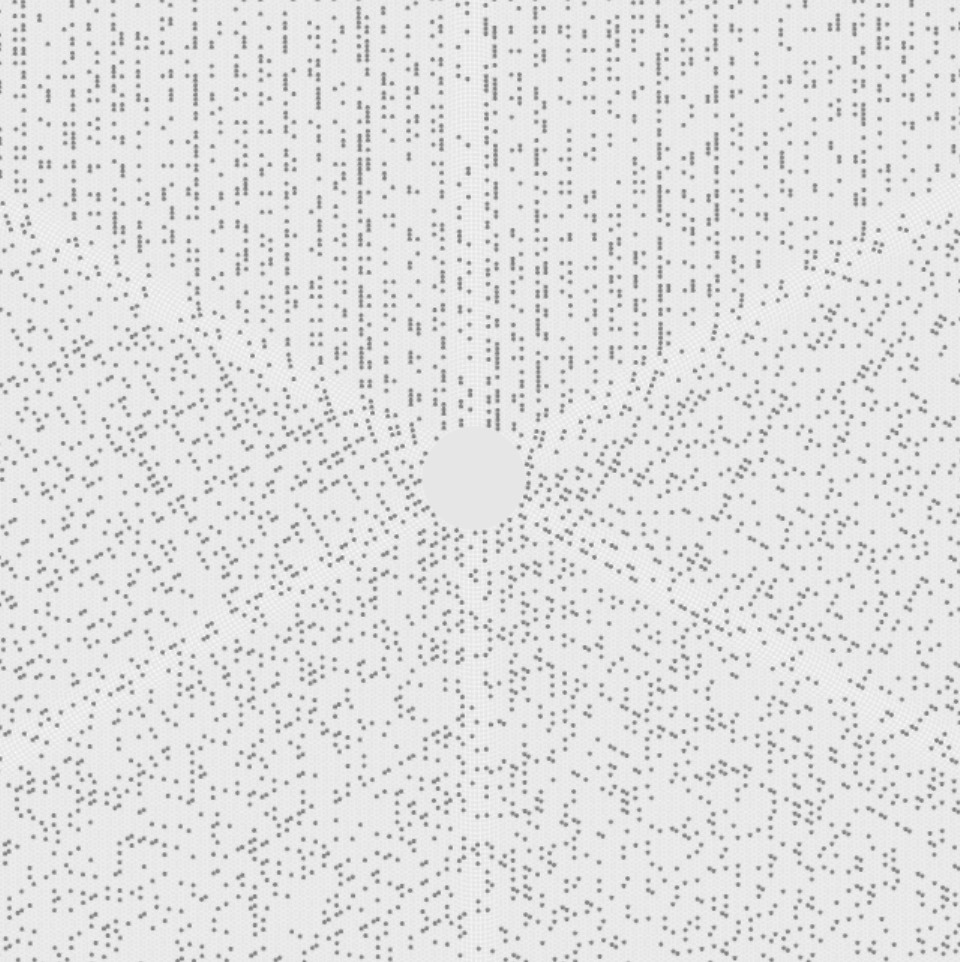
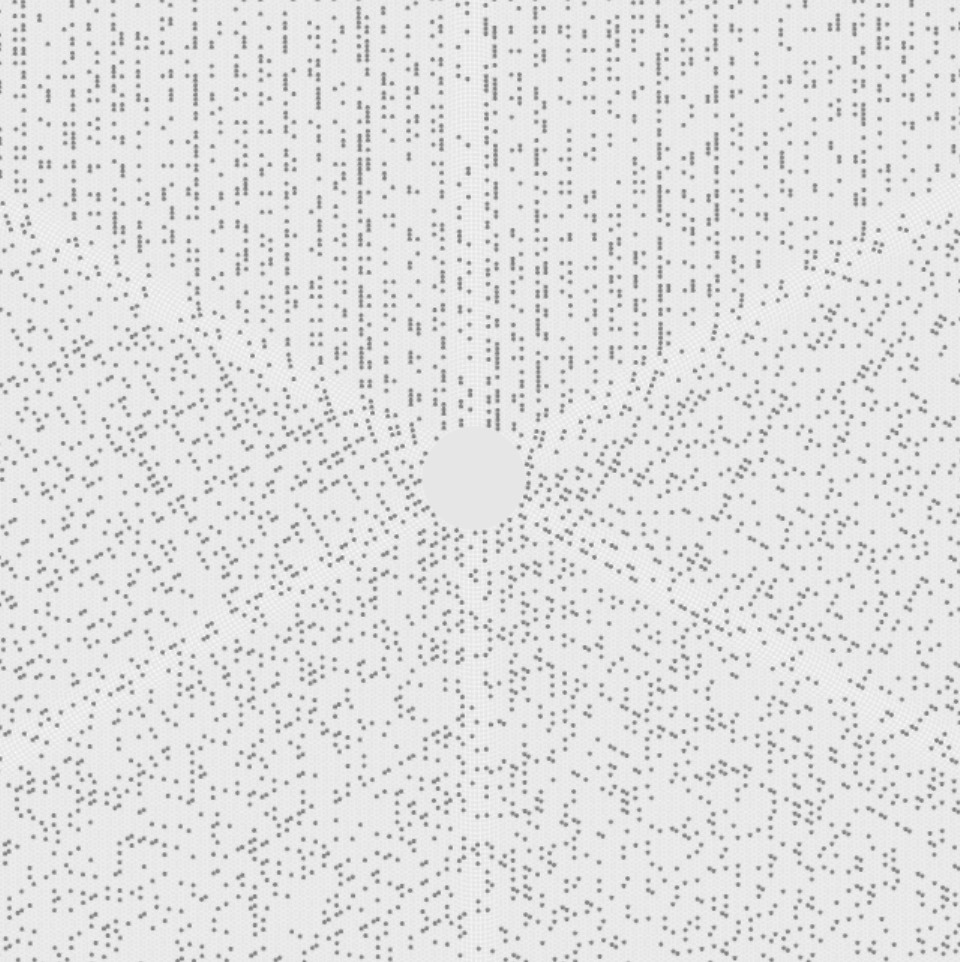
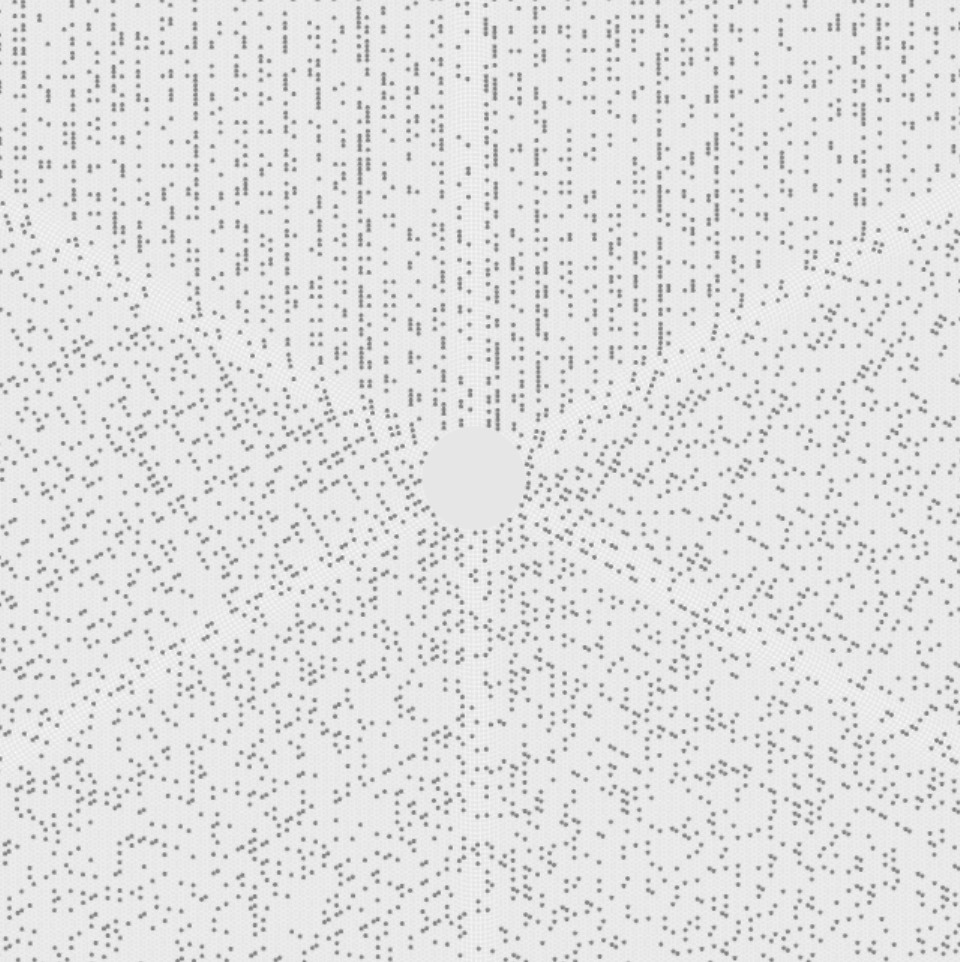
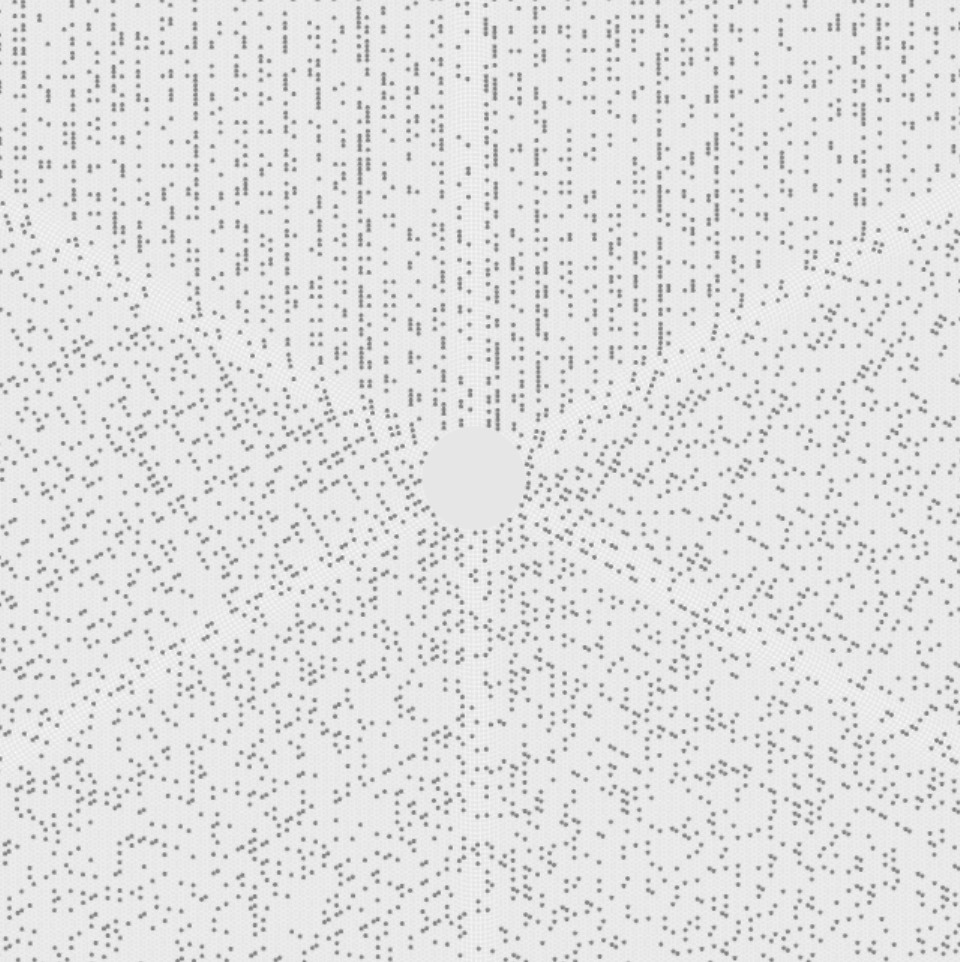
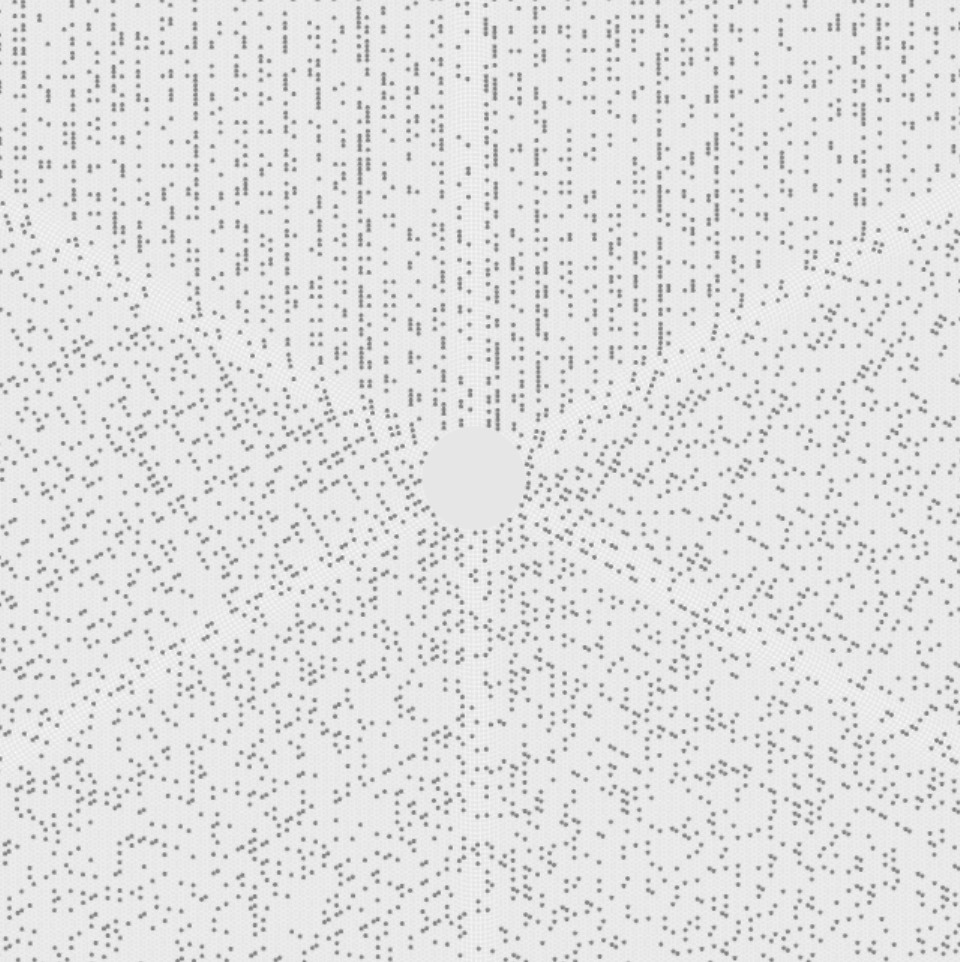
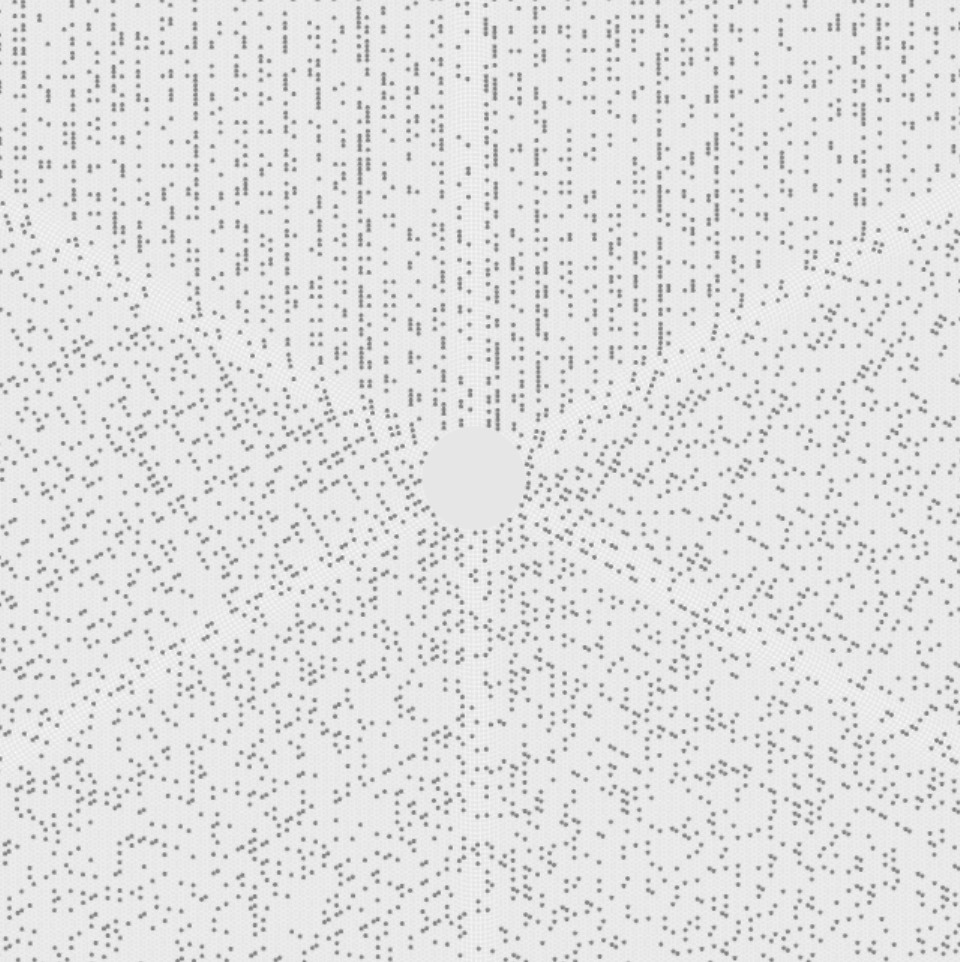
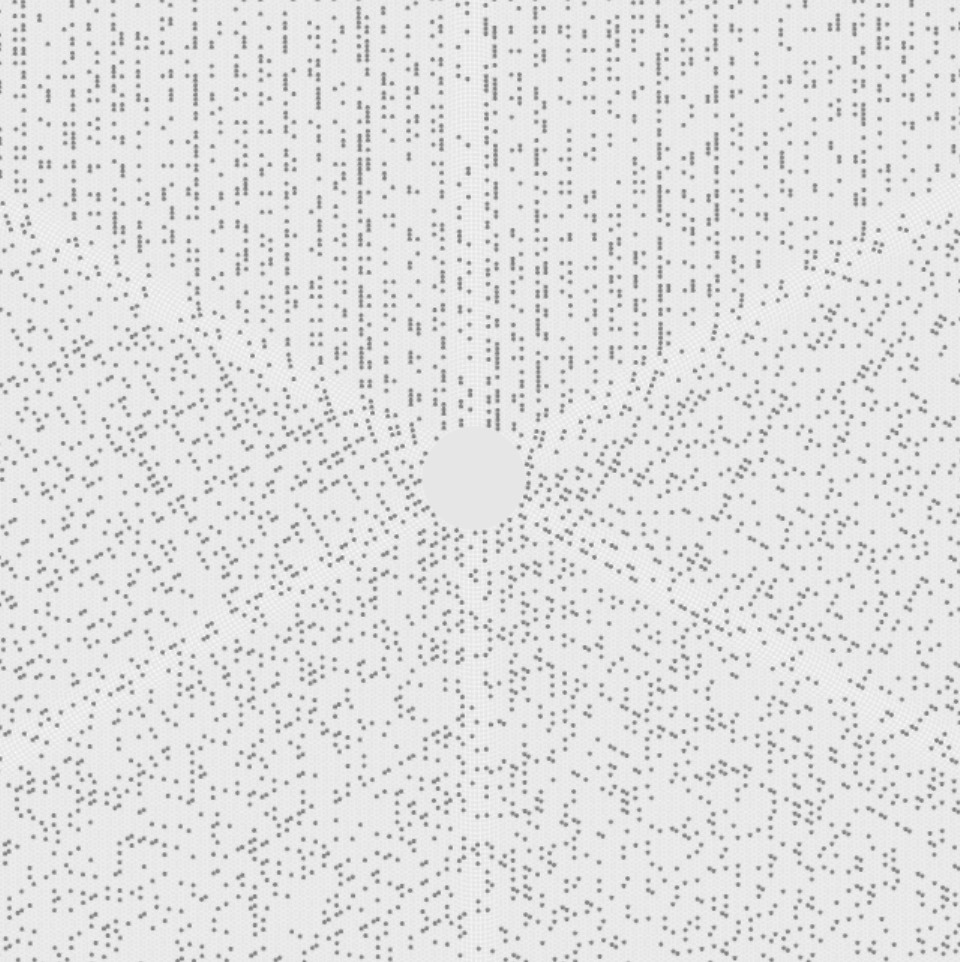
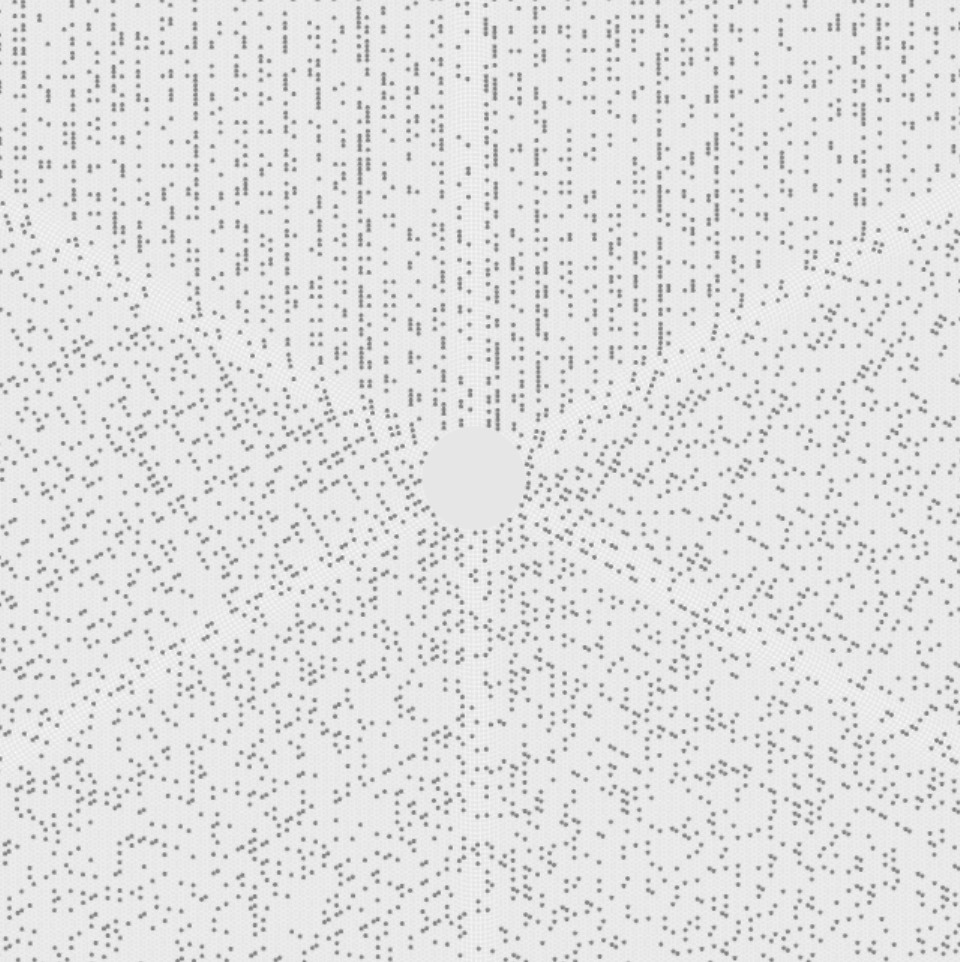
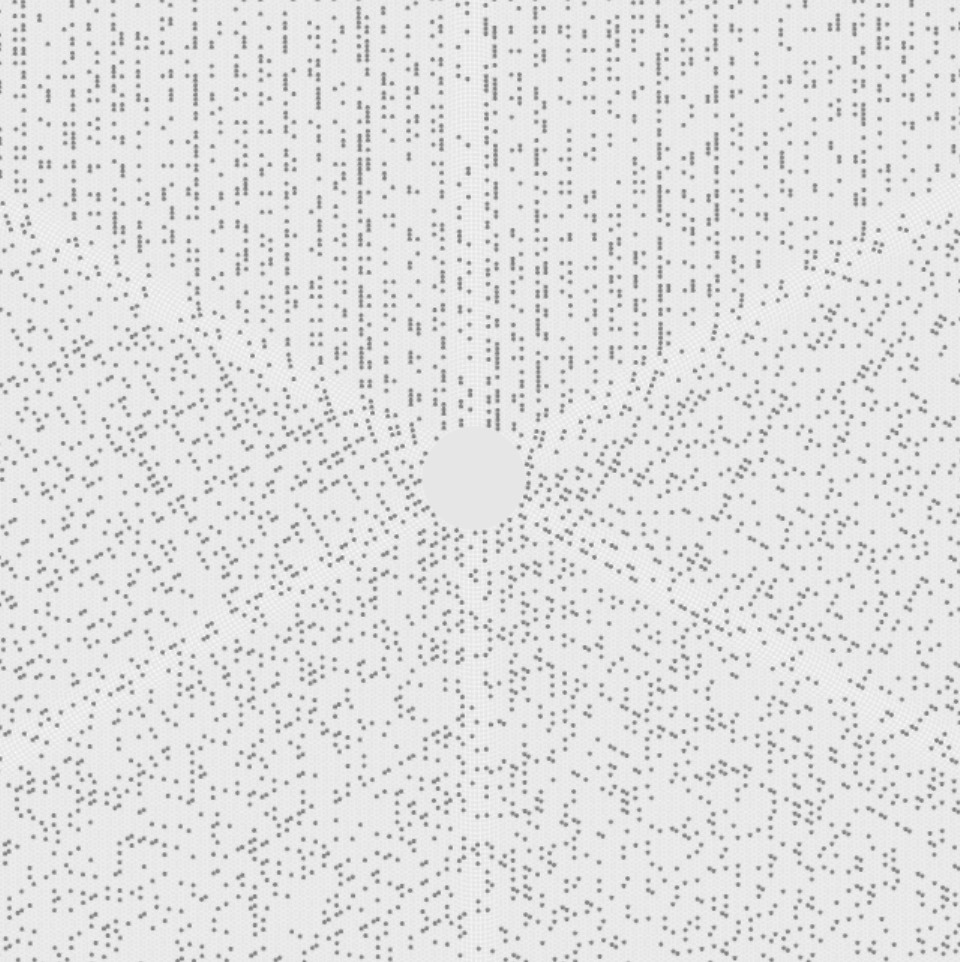
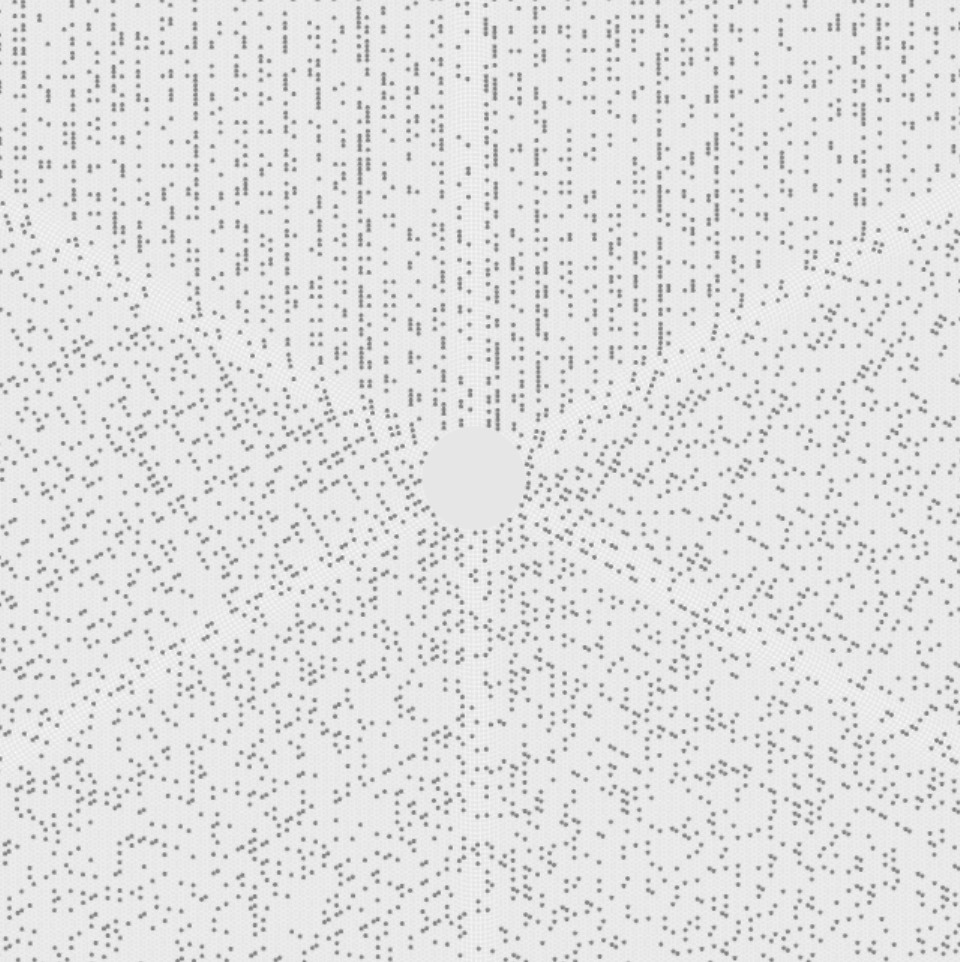
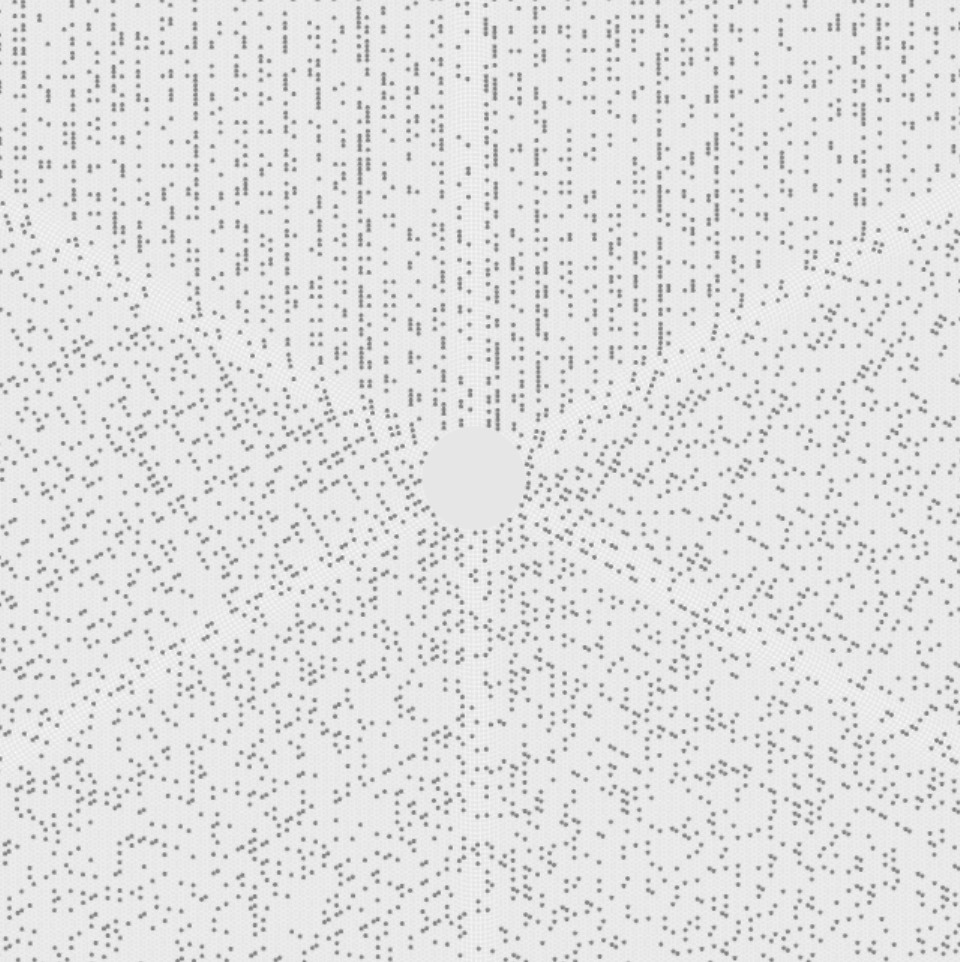
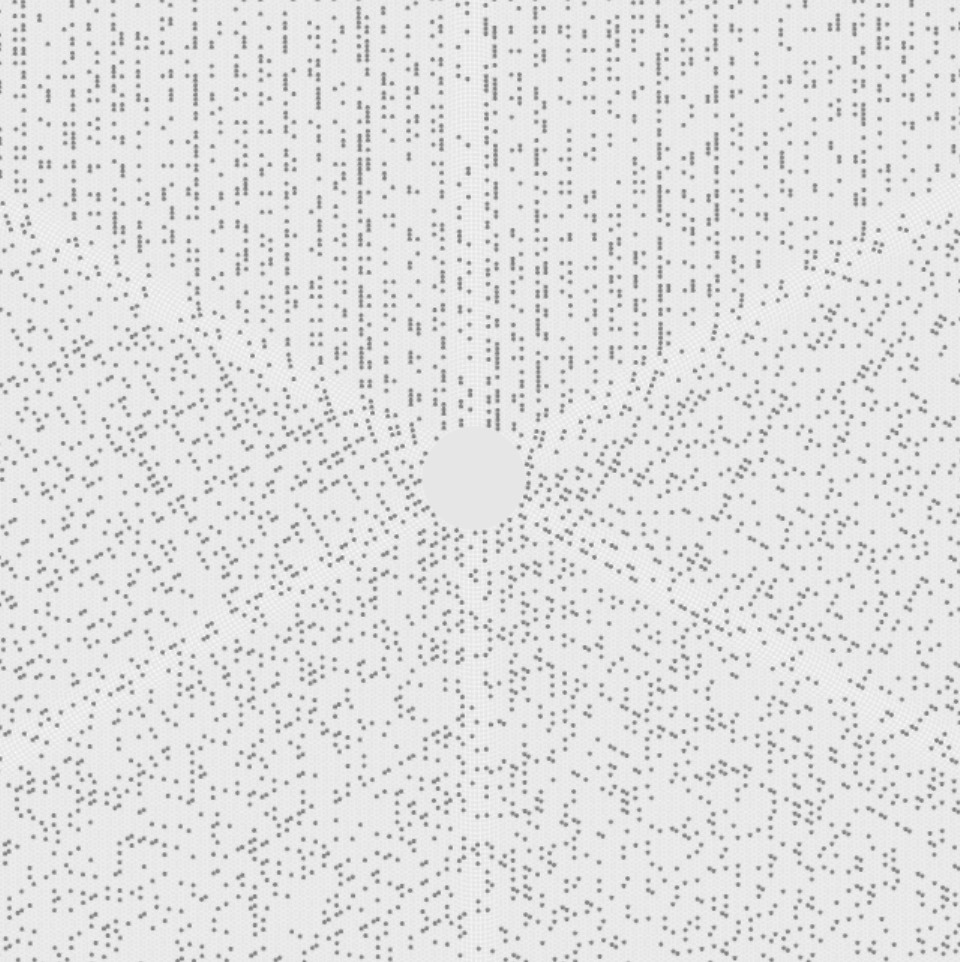
Thursday 8 August
10:45
Arrival
11:00
Computer-aided conjecture generation in maths and physics
Proposing good conjectures is at least as valuable as proving theorems. Good conjectures capture our attention and orient our efforts, acting like guide posts on the 'mazy paths to hidden truths' (Hilbert). This talk will touch on three aspects of computer-aided conjecture generation: matching numerical values, symbolic regression and generative models. I will present a Zipf-type law for Physics equations, discuss how genetic algorithms can be used to identify new identities of Rogers-Ramanujan type and present a number of conjectural generating functions for holomorphic line bundle cohomology on certain complex projective varieties.
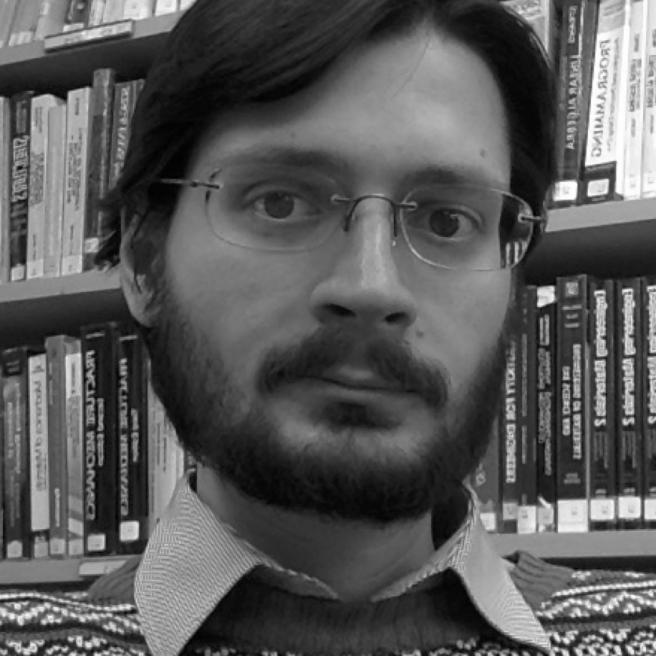
Andrei Constantin
Dr Andrei Constantin is a Royal Society Dorothy Hodgkin Fellow, a research fellow and a tutor in physics and maths at Oxford, where he did his PhD. He works on developing tools to investigate string theory and its implications for particle physics, cosmology and quantum gravity.
12:00
Lunch
13:00
Asymptotic formulae for the regularized quantum period
In this talk, we explore the asymptotic behaviour a certain sequence, which contains the coefficients of a series called the regularized quantum period. This is conjectured to be a complete invariant of Fano varieties and its can be considered as a numerical fingerprint of each Fano variety. We discuss how these results originate from the study of large datasets using data analysis and machine learning techniques and show how they can be used to visualise the landscape of these objects. This is joint work with Tom Coates and Alexander Kasprzyk.
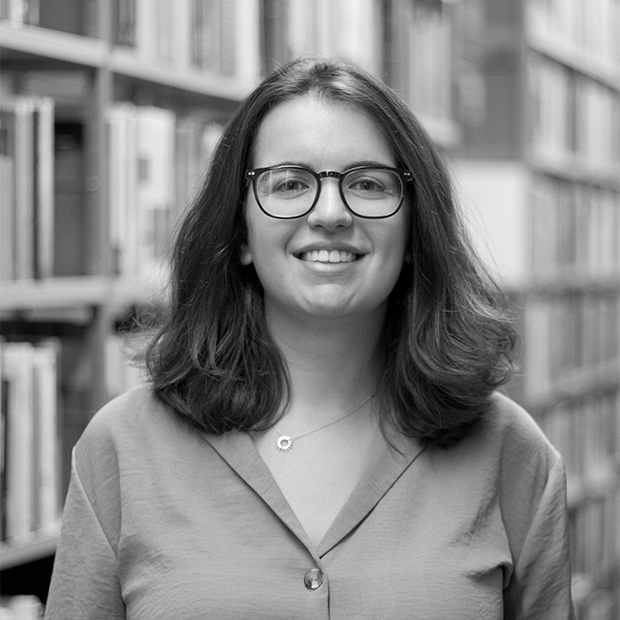
Sara Veneziale
Sara Veneziale is a PhD student at Imperial College London. Her research focuses on applying machine learning and data analysis tools to pure mathematics problems, with the aim of helping conjecture formulation. Before her PhD, she studied mathematics at the University of Warwick.
14:00
Modelling machine learning systems
How can we make sure that a machine learning system gives results as it should, is not biased or is safe to use–as we would like for self-driving cars, for example? We cannot. Not fully. But we can try! This talk will be an incursion in theoretical computer science and formal verification. We will explain ways one can model machine learning systems to try to obtain partial guarantees on them, and how to enforce some safety property.
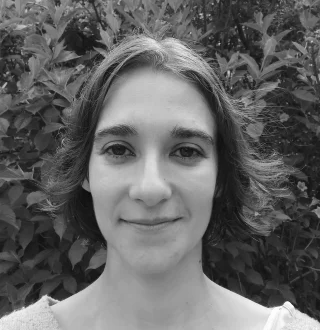
Laure Daviaud
Dr Laure Daviaud is Associate Professor in the School of Computing Sciences at the University of East Anglia. She did her PhD at the Institut de recherche en informatique fondamentale at the CNRS and Université Paris Cité. She develops mathematical models for computer systems.
14:30
Formalising topological data analysis in Cubical Agda
Topological data analysis (TDA) offers a plethora of methods and tools to study the “shape of data”. This talk is about work towards implementing a TDA tool inside a theorem prover, leading to software that is formally proved to implement the intricate mathematical theory underlying TDA. The theorem prover of choice for this project is Cubical Agda, which implements ideas from Homotopy Type Theory and offers a logic to directly reason about (homotopy types of) topological spaces. We give a taste of what it’s like to reason in this theorem prover, presenting a formalisation of discrete Morse theory for graphs, and discuss challenges for turning this formalisation into a fully fledged tool for TDA.
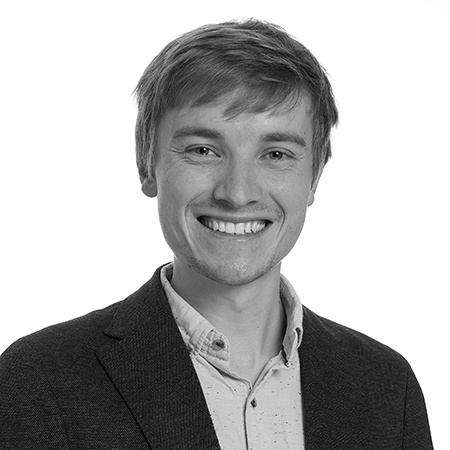
Maximilian Doré
Dr Maximilian Doré will join the Department of Computer Science at Oxford as a lecturer this autumn. He did his PhD at Oxford. His research focuses on how computer proof assistants are changing mathematics, and on foundations and applications that could help to shape this change.
15:00
Break
15:30
Machine Learning of the Prime Distribution
We provide a theoretical argument explaining the experimental observations of Yang-Hui He about the learnability of primes, and posit that the Erdős-Kac law would very unlikely be discovered by current machine learning techniques. Numerical experiments that we perform corroborate our theoretical findings. This is joint work with A. Alistair Rocke.
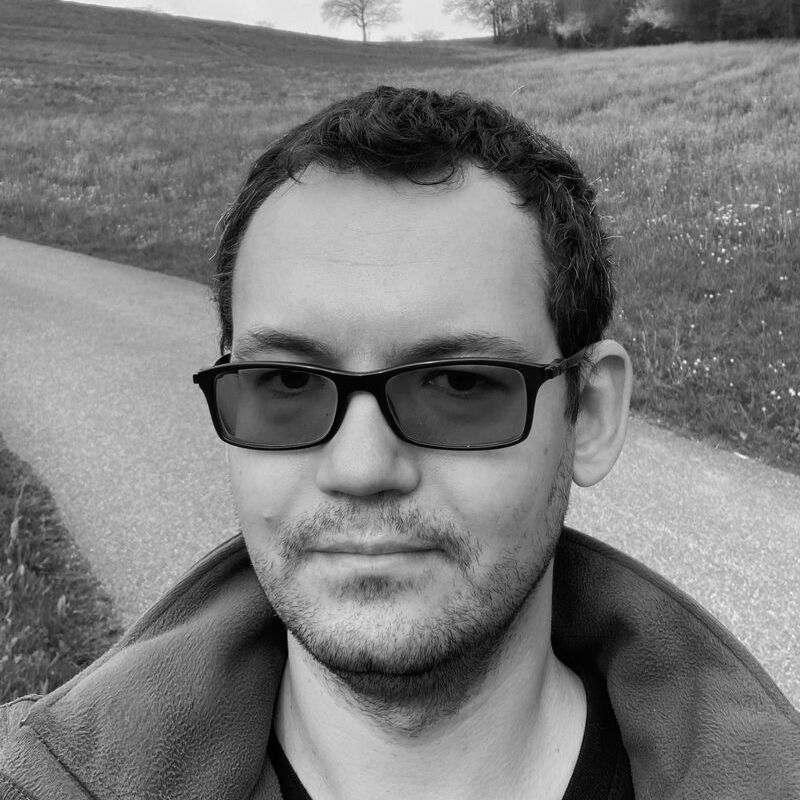
Alexander Kolpakov
Prof. Alexander Kolpakov is an Associate Professor at the University of Austin. He did his PhD at the University of Fribourg in Switzerland. His work ranges from combinatorics to Riemannian geometry, to applications in data science, machine learning and computer vision.
16:30
Precision string phenomenology
Calabi-Yau compactifications of string theory lead to quantum field theories in four dimensions with chiral matter. Calculating parameters of the low-energy effective theory in general compactifications requires the Ricci-flat metric on the Calabi-Yau manifold. Such metrics are not known analytically. In this talk, we discuss how to approximate the Ricci-flat metric using neural networks. The accuracy of the numerical metrics is assessed for K3 and the quintic threefold. In the standard embedding, we calculate Yukawa couplings for compactifications on various Calabi-Yau geometries. This is an initial step toward a first principles calculation of particle masses from string theory.
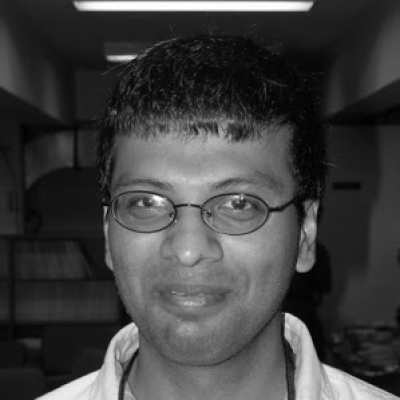
Vishnu Jejjala
Prof. Vishnu Jejjala is the South African Research Chair in Theoretical Particle Cosmology at Johannesburg's University of the Witwatersrand. He did his PhD at the University of Illinois Urbana-Champaign. He works on quantum gravity and the structure of quantum field theories.
Friday 9 August
10:00
Math+AI=AGI
In this talk, we explore the transformative potential of off-the-shelf reinforcement learning (RL) algorithms in accelerating solutions to complex, research-level mathematical challenges. We begin by illustrating how these algorithms have achieved a 10× improvement in areas where previous advances of the same magnitude required many decades. A comparative analysis of different network architectures is presented to highlight their performance in this context. We then delve into the application of RL algorithms to exceptionally demanding tasks, such as those posed by the Millennium Prize problems and the smooth Poincaré conjecture in four dimensions. Drawing on our experiences, we discuss the prerequisites for developing new RL algorithms and architectures that are tailored to these high-level challenges.
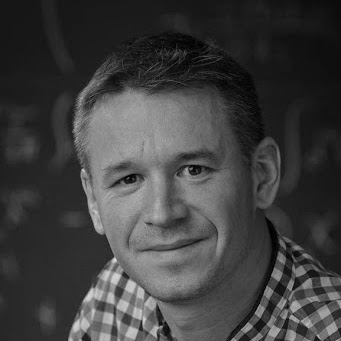
Sergei Gukov
Prof. Sergei Gukov is the John D. MacArthur Professor of Theoretical Physics and Mathematics at Caltech. He did his PhD at Princeton. He works across wide-ranging areas of mathematical physics and pure mathematics, including quantum topology, mirror symmetry and gauge theory.
11:00
Reproducing kernel Hilbert C*-module for data analysis
Reproducing kernel Hilbert C*-module (RKHM) is a generalisation of Reproducing kernel Hilbert space (RKHS) and is characterised by a C*-algebra-valued positive definite kernel and the inner product induced by this kernel. The advantages of applying RKHMs to data analysis instead of RKHSs are that we can enlarge representation spaces, construct positive definite kernels using the product structure in the C*-algebra, and use the operator norm for theoretical analyses. We show fundamental properties in RKHMs for data analysis, such as a minimization property of the orthogonal projection and representer theorems. Then, we propose a deep RKHM, which is constructed as the composition of multiple RKHMs. This framework is valid, for example, for analysing image data.
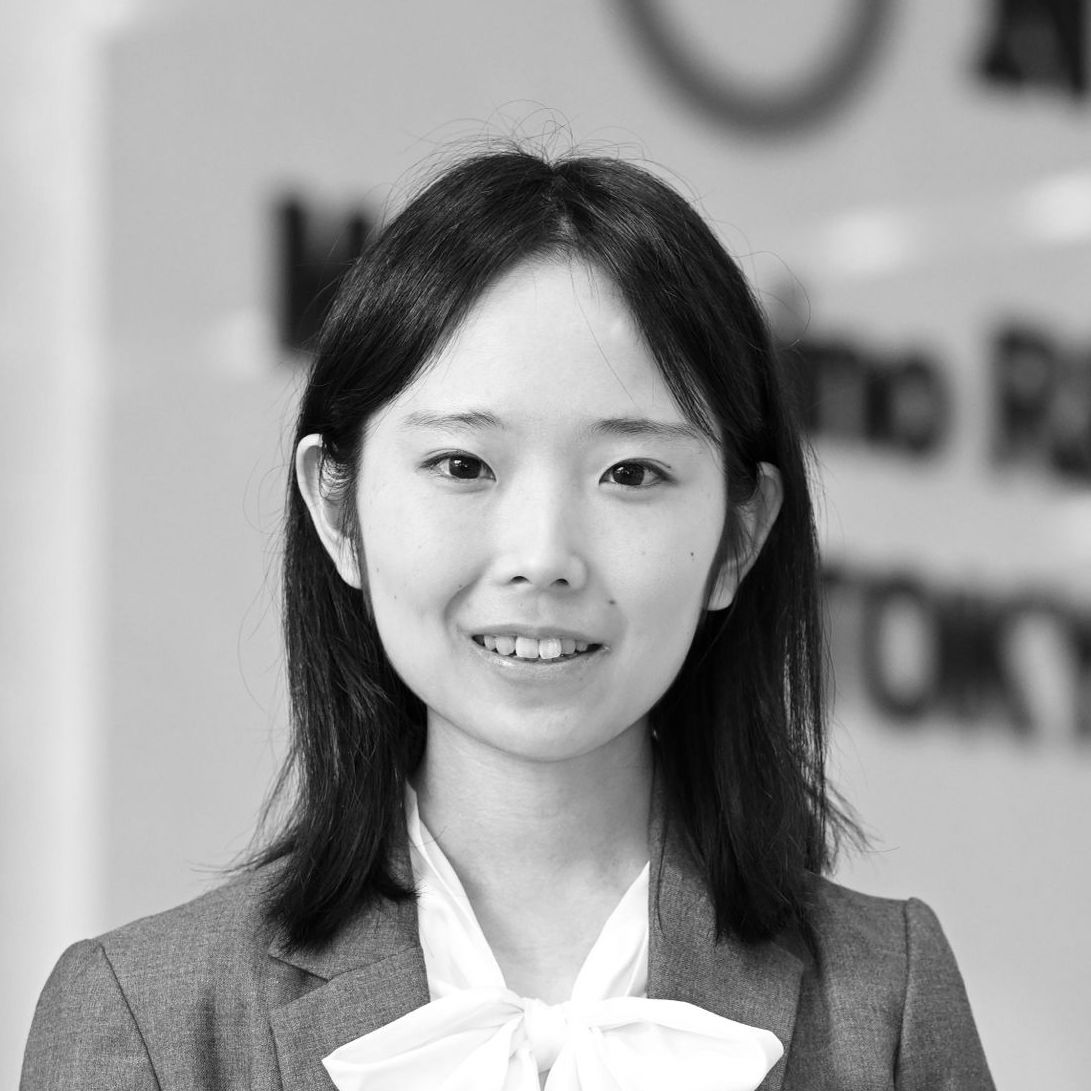
Yuka Hashimoto
Dr Yuka Hashimoto is a Distinguished Researcher at Japan’s NTT Network Systems Laboratories. She did her PhD at Keio University. In her work she is interested in automation technologies for network operation, operator theoretic data analysis and numerical linear algebra.
12:00
Lunch
13:00
Machine models for weighted spaces
We explore the idea of using machine learning to determine rational points on weighted projective varieties. Our work leads to many interesting questions on the geometry of weighted projective spaces and how machine learning models can be used to study weighted spaces. While mathematically it seems that such models would be superior to more traditional models, it is still an open question to provide computational evidence to support such claims.
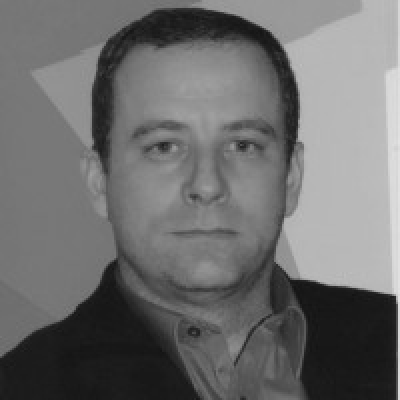
Prof. Tony Shaska
Prof. Tony Shaska is a professor at Oakland University, working on algebra and its applications. His most recent research concerns the use of artificial neural networks in problems of pure mathematics such as invariant theory, computational algebraic geometry, and Galois theory.
14:00
Break
14:15
Machine learning in the moduli space of genus two curves
We use machine learning to study the moduli space of genus two curves. During the talk, we will focus on the locus Lₙ of genus two curves with (n,n)-split Jacobian. More precisely, we design a transformer model which, given moduli points (i.e., values for the Igusa invariants), determines if the corresponding genus two curve is in the locus Lₙ, for n = 2, 3, 5, and 7. During this study we discover some interesting arithmetic properties which seem difficult to guess otherwise. For example we show that there are no rational points p∈ Lₙ with weighted moduli height ≤ 2 in any of L₂, L₃, and L₅.
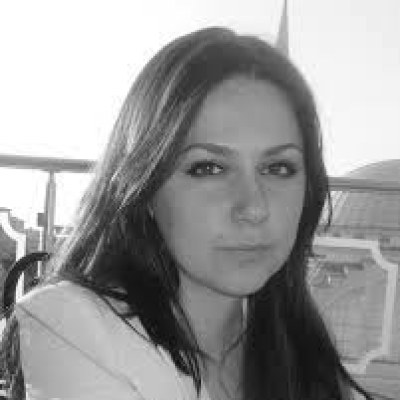
Elira Shaska
Elira Shaska is a PhD student at Oakland University. She uses machine learning in computational algebraic geometry, specifically to study moduli spaces of curves and weighted varieties. Before her PhD, she completed a masters in computer science, with a focus on AI, at Oakland.
15:15
Break
15:30
Applying a computational algebra and data science pipeline to Clifford algebra and cluster algebra data
Rather than relying on existing data sets to data mine, the combination of computational algebra and data science techniques allows one to simultaneously create algebraic data sets and to then apply standard data science techniques in order to try to find patterns in this generated data through exploratory data analysis. Ultimately, this could help complement mathematical intuition in guiding hypothesis formulation and theorem proving. We will show examples from recent work on cluster algebras and Clifford algebras, such as machine learning interesting new Clifford invariants of linear functions, using the example of Coxeter elements as transformations of interest with links to other, well-known, geometric invariants.
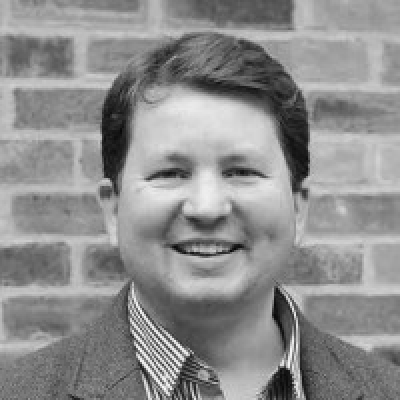
Pierre-Philippe Dechant
Dr Pierre-Philippe Dechant is a lecturer at the University of Leeds, where he is setting up a BSc in data science. He did his PhD in Cambridge in theoretical astrophysics. He is interested in the intersection of algebra, data science and mathematical biology.