AI-assisted mathematical discovery
10 AM – 10 PM, 28 Oct 2022
The London Institute hosts a day symposium on using AI to speed up mathematical discovery, followed by a panel discussion, drinks and dinner.
Good conjectures can inspire new branches of mathematics and theoretical physics. They usually come from spotting patterns and applying instinct. Because in mathematics there are no coincidences, automated pattern detection is immune to the false positives that plague experimental science. Can machines help us make mathematical discoveries and speed up theoretical research?
There has been a recent surge of interest in using machine intelligence to study various aspects of geometry, topology and representation theory. Theoretical physics has helped drive these developments, through the percolation of ideas and analogies and by providing an effective testbed for learning methods. One pertinent example is machine-driven studies of the algebraic geometry of Calabi—Yau spaces, which appear as extra-dimensions in string theory.
In this one-day symposium, we discuss how to assist and automate new mathematical discovery by exploiting novel computation and deep learning, as well as insights into the theory of learning itself. At 4pm there is a high-level panel discussion for a broader audience on the future of AI-assisted discovery, and the roles of academia versus industry. This is followed by drinks and dinner on site.
A two-way street
Several of the success stories in AI-assisted mathematics have relied on the deep learning architectures of neural networks and their remarkable ability to act as universal function approximators. Well-known feature attribution techniques recently led to exciting new results in knot theory and representation theory. Even number theory, normally resistant to such approximations, is now proving susceptible to certain forms of learning.
But the reverse is also true: mathematics is shedding light on deep learning. As we develop better theories of learning, profound connections to well-studied areas of mathematics are becoming apparent. The Kolmogorov-Arnold representation theorem seems structurally similar to neural networks, with deep connections to Hilbert’s 13th problem. More recently, researchers established that training in neural networks, when viewed geometrically, is a variant of Ricci flow. Using such links to leverage modern machine learning algorithms will lead to surprising breakthroughs in mathematics and physics, as well as a better understanding of machine learning itself.
Programme
Old Post Room
- 10:00 Coffee, meet and greet
- Tyndall Parlour
- 10:20 LIMS, AI and mathematics: Thomas Fink
- 10:30 Opening remarks: Challenger Mishra
- 10:40 Knot theory and machine learning: Andraz Juhasz
- 11:00 Machine learning with mathematicians: Alex Davies
- 11:20 Teaching theorems to computers: Kevin Buzzard
- Old Post Room
- 11:40 Coffee and discussion
- Tyndall Parlour
- 12:00 Machine-Learning Mathematical Structures: Yang-Hui He
- 12:20 Towards a human-like reasoning system: Mateja Jamnik
- 12:40 Draft, sketch and prove: Wenda Li
- 13:00 Local pub lunch and sandwiches on site in Bragg’s Dining Room
- Tyndall Parlour
- 14:00 Peter Braam
- 14:20 Syllogistic reasoning and beyond: Tiansi Dong
- Old Post Room
- 14:40 Coffee and discussion
- Bragg’s Dining Room
- 15:00 Speed dating: AI vs mathematics
- 16:00 Break
- Tyndall Parlour
- 16:15 High-level panel discussion with business leaders and journalists
- 17:45 Drinks in the Old Post Room
- 19:00 Dinner in Faraday’s Study
Talks
To encourage understanding and discussion, talks are 20 minutes long and can have at most three slides. A blackboard is available to explain ideas and results.
Venue
We will meet in the Tyndall Parlour of the London Institute, which is on the second floor of the Royal Institution.
Financial support
This symposium is funded by the Henderson Institute at the Boston Consulting Group. For those outside of London, we can cover travel to London and overnight accommodation. Additional sponsorship comes from the Accelerate Program for Scientific Discovery at The Computer Laboratory, Cambridge.
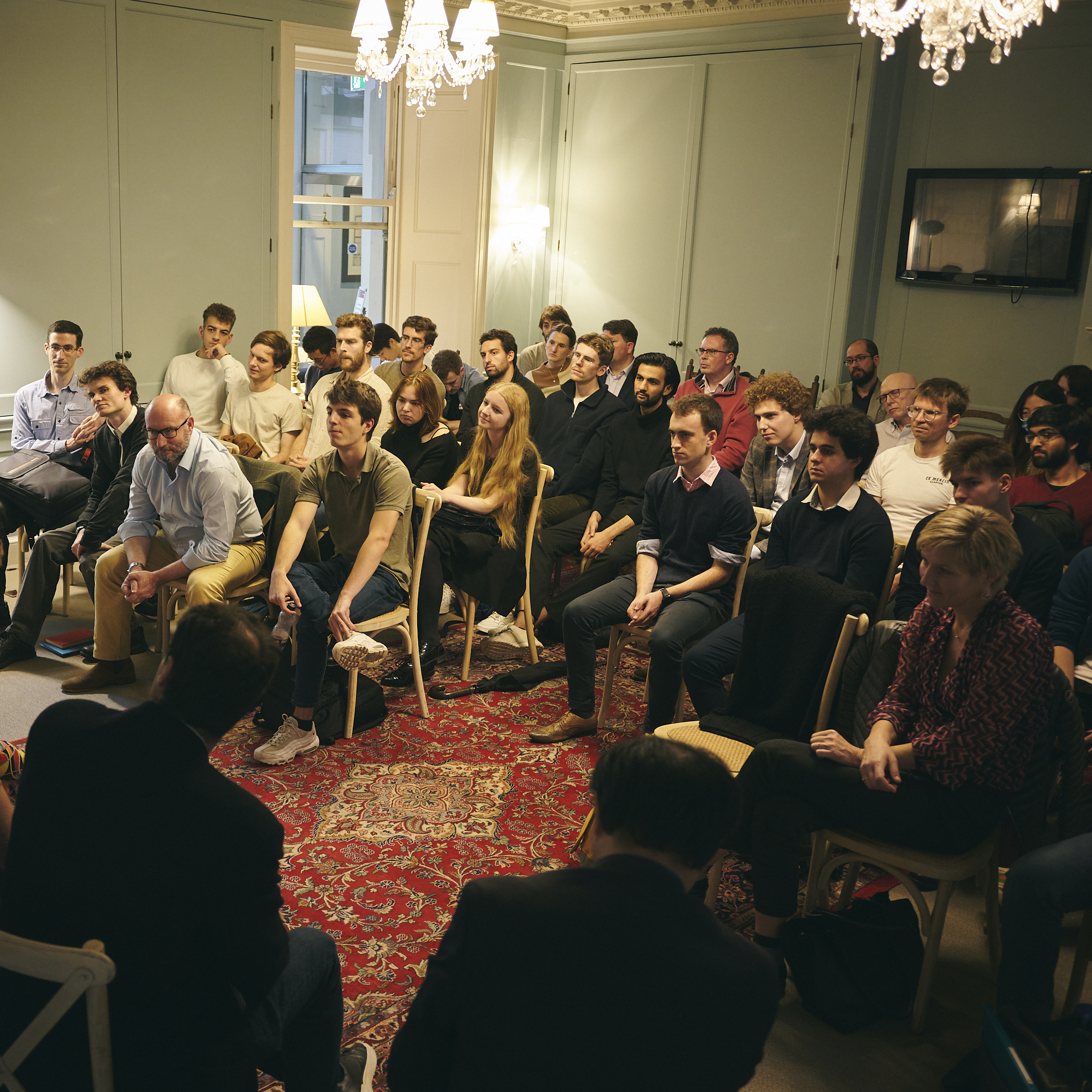
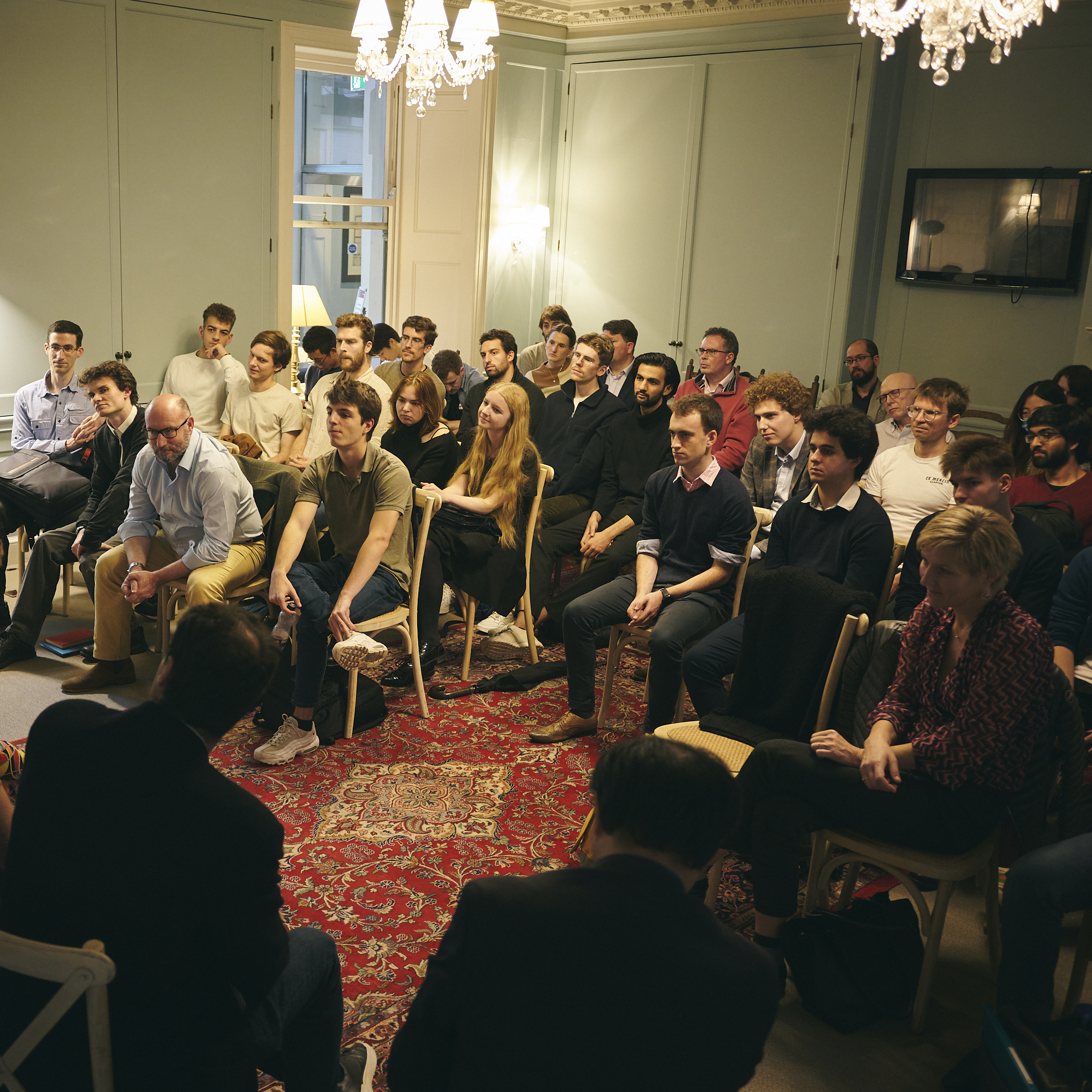
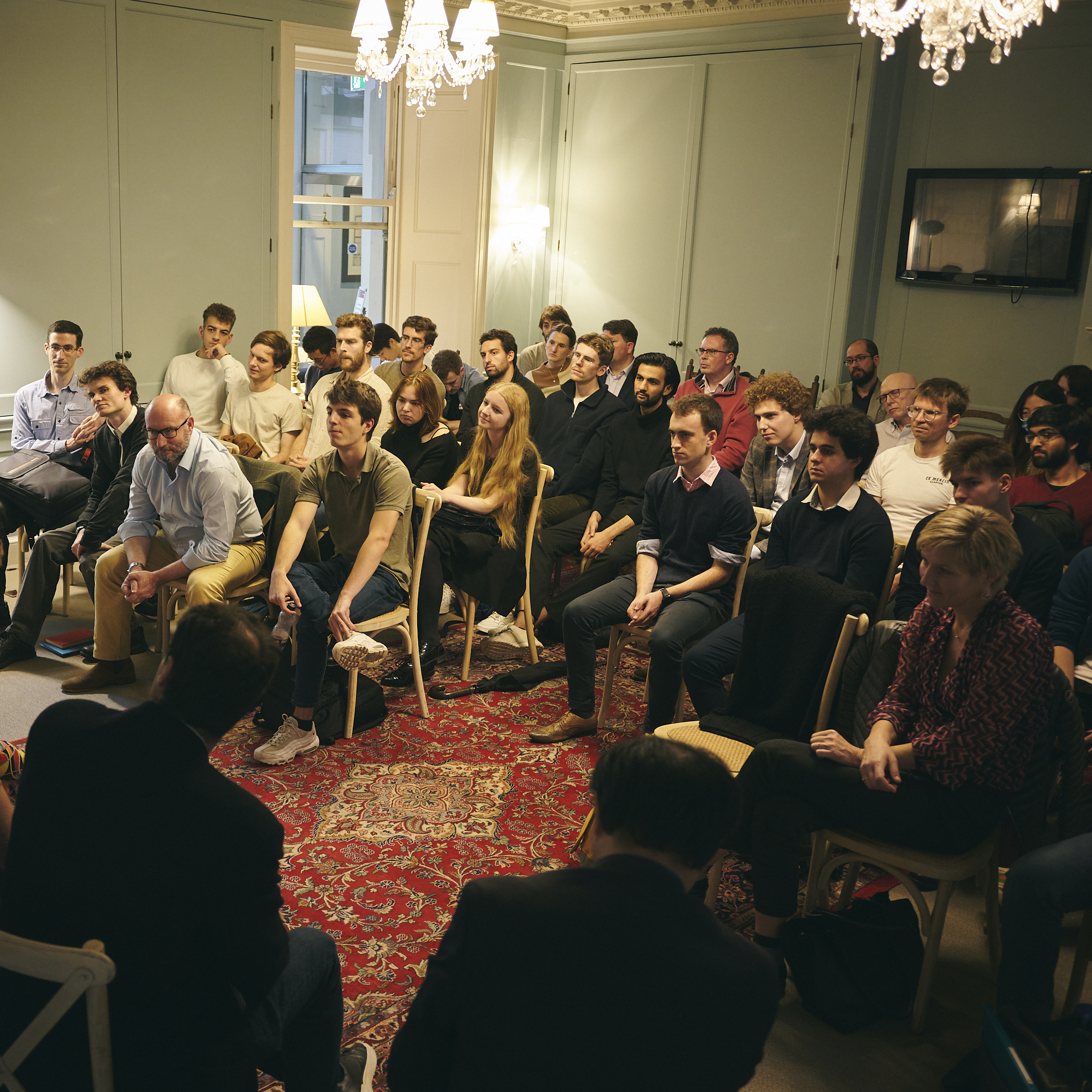
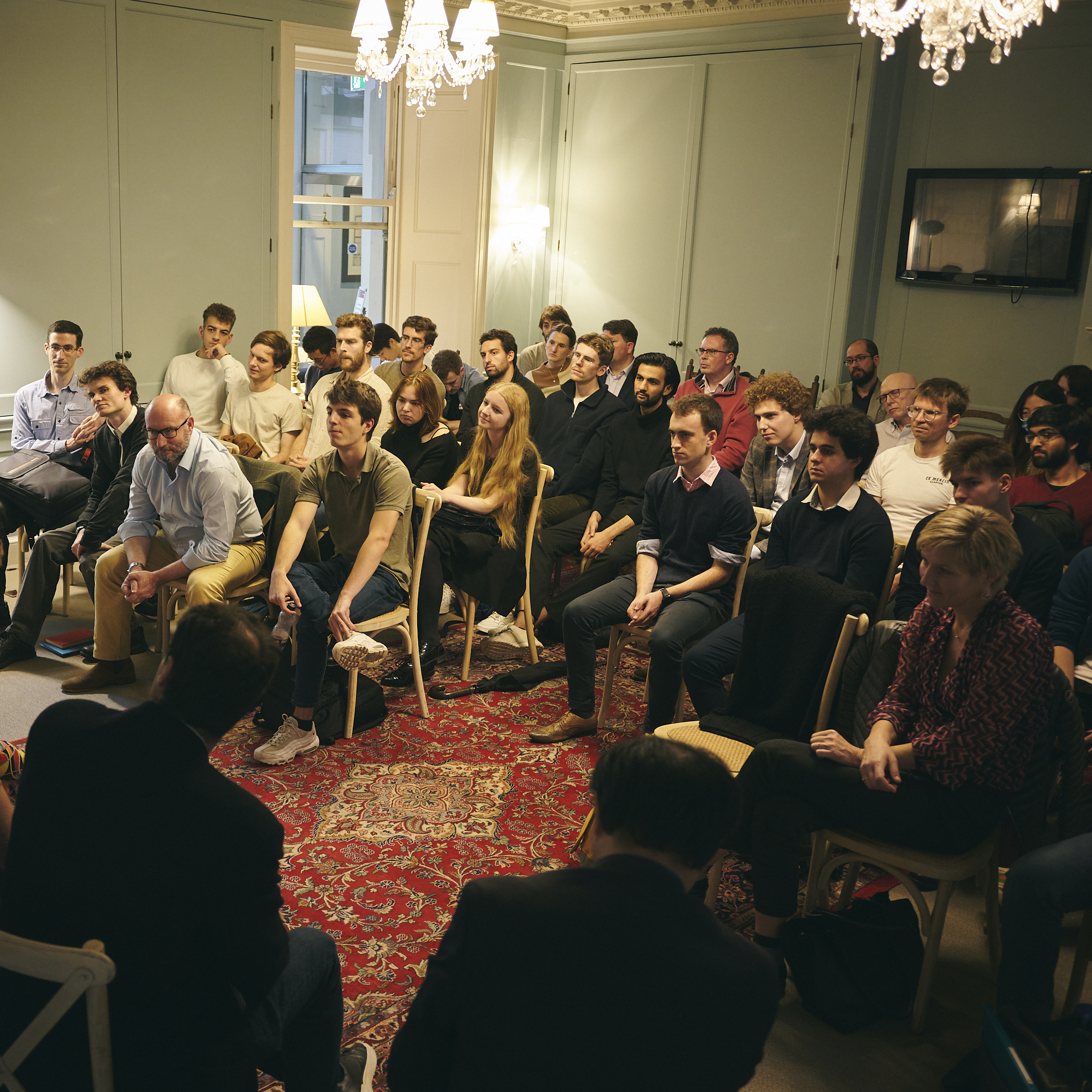
LCP
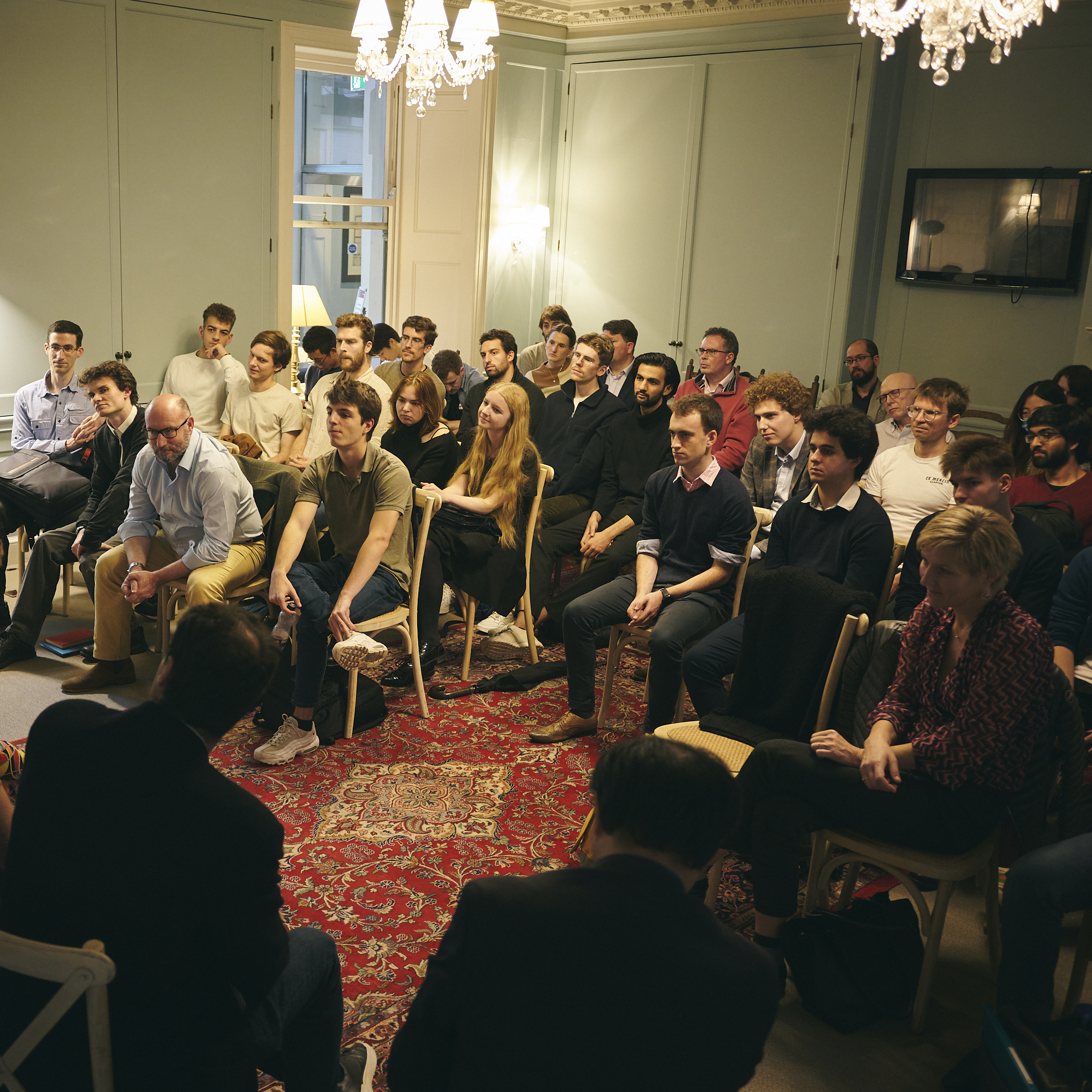
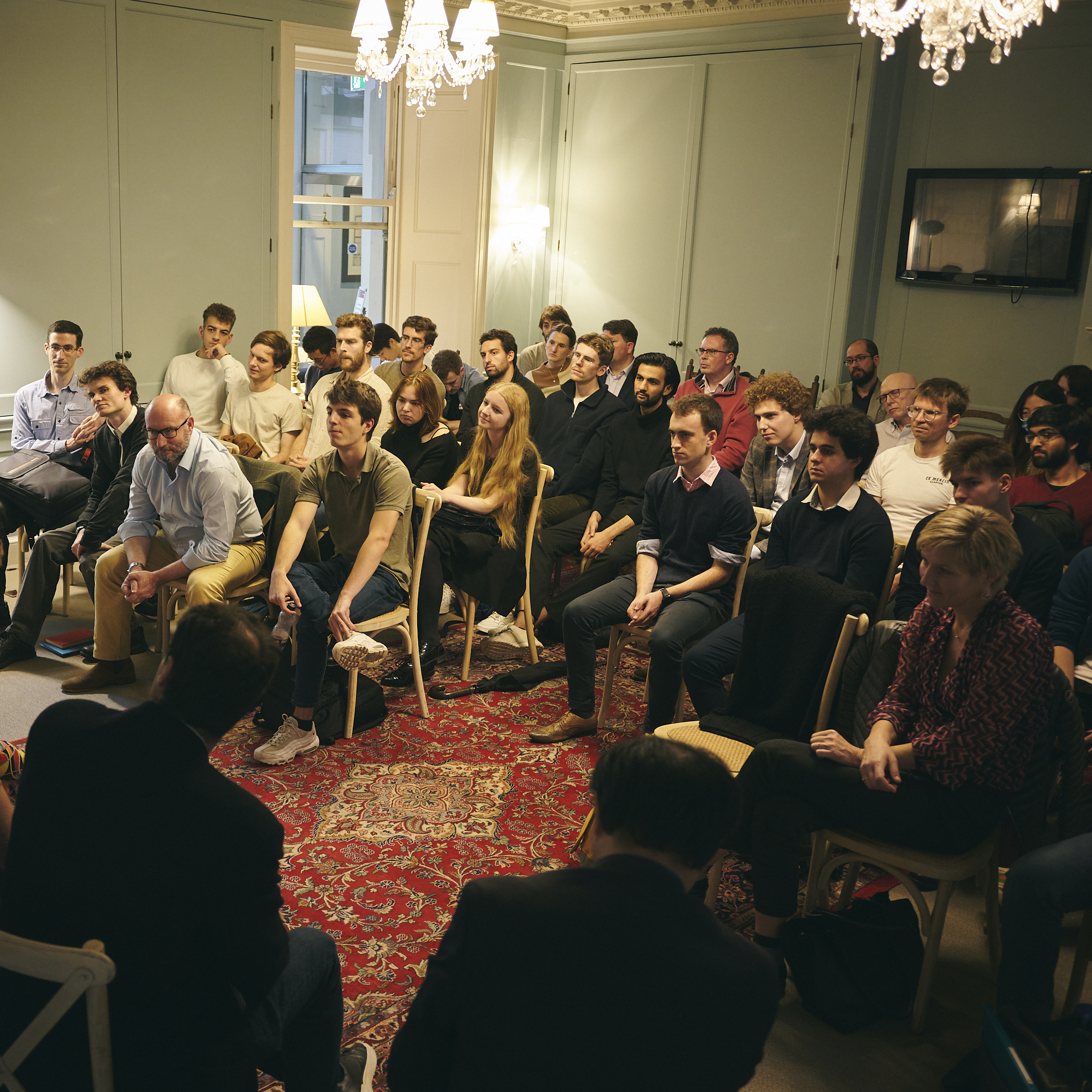
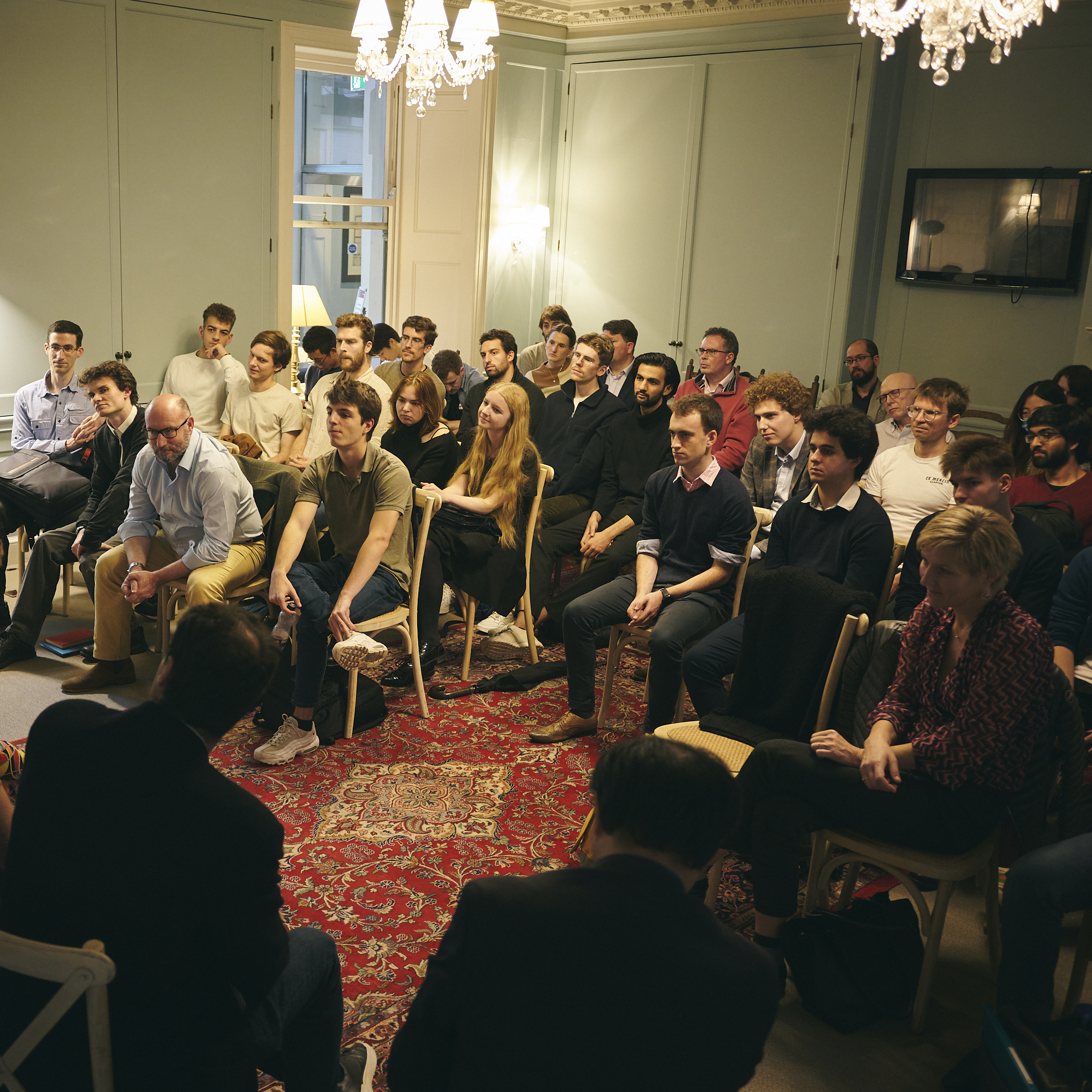
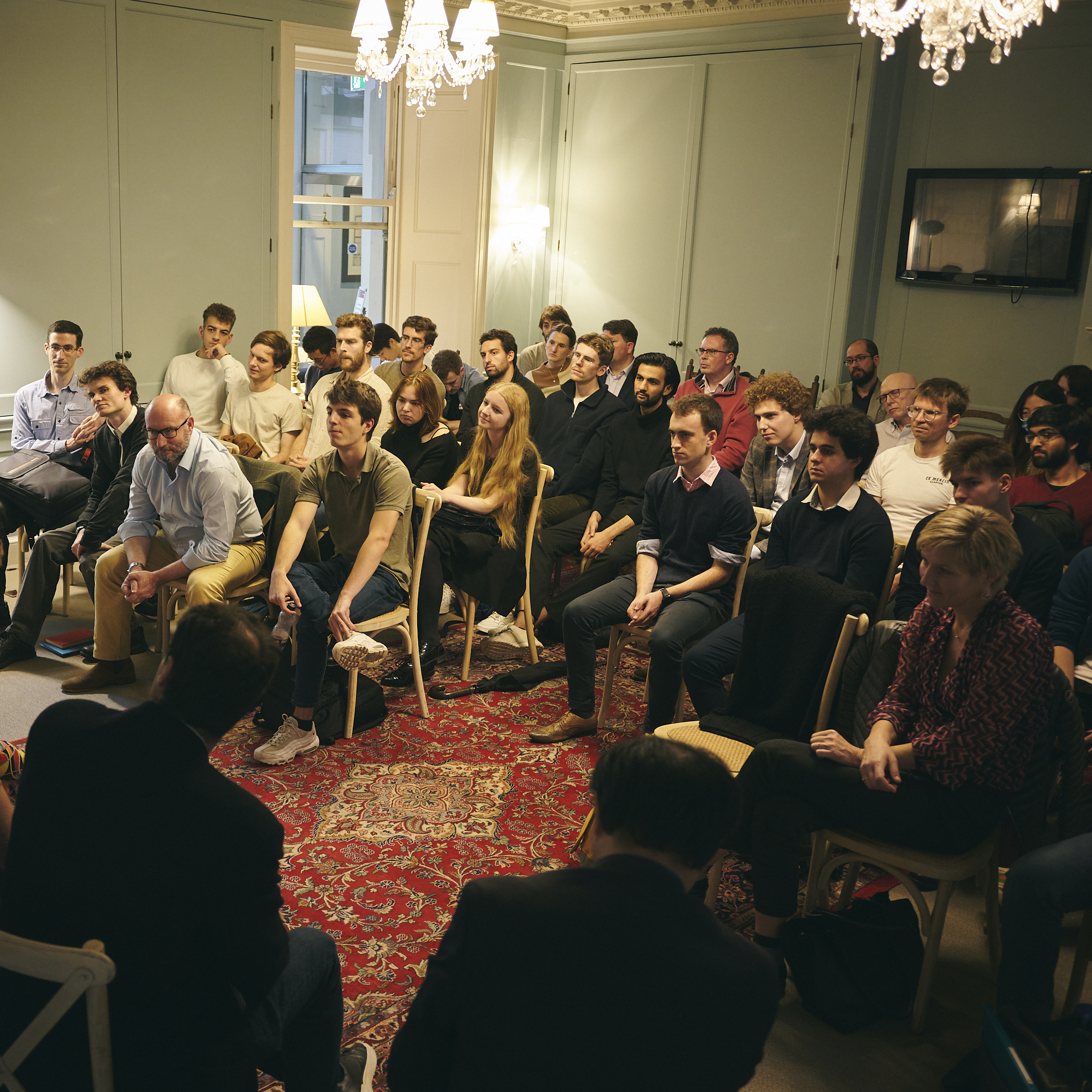
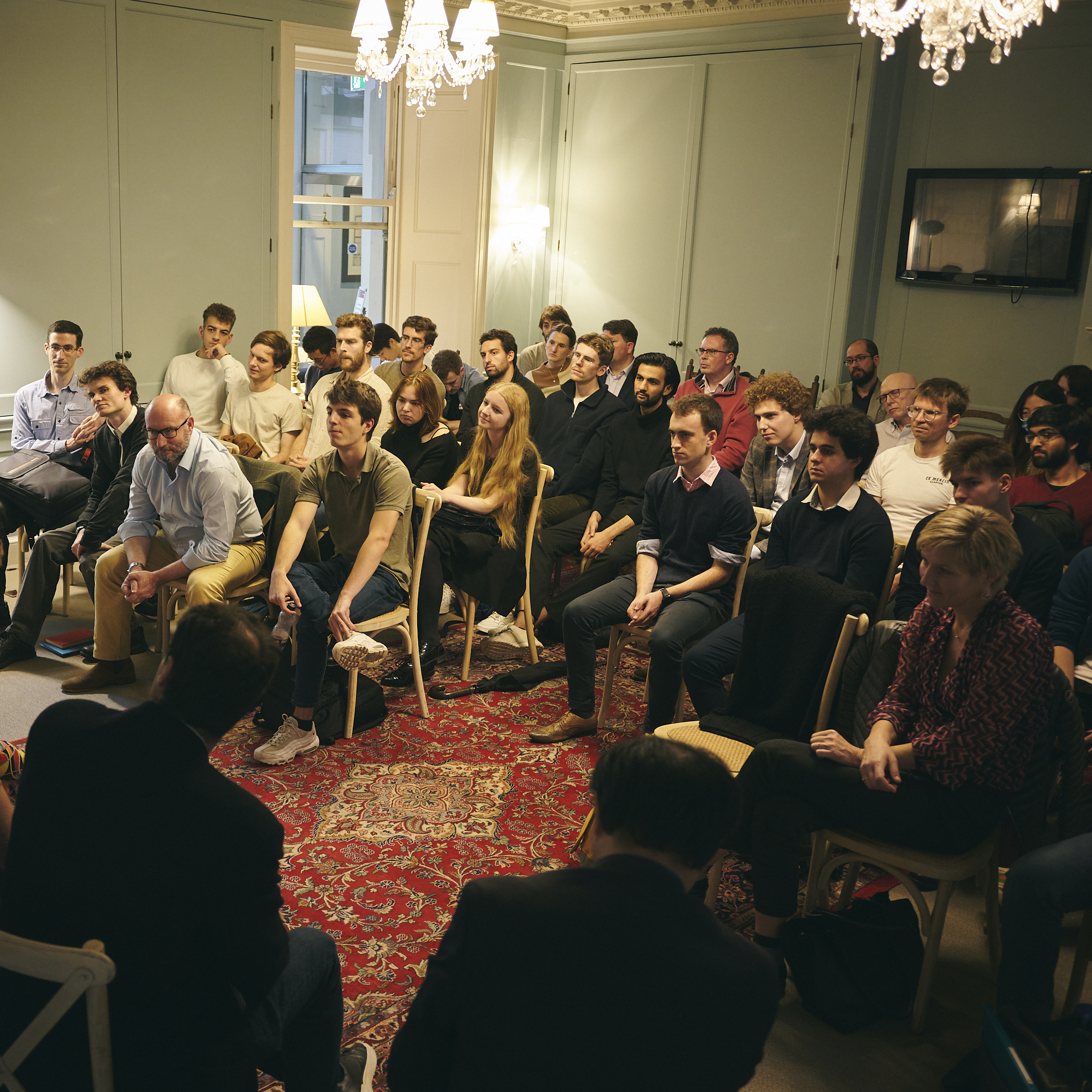
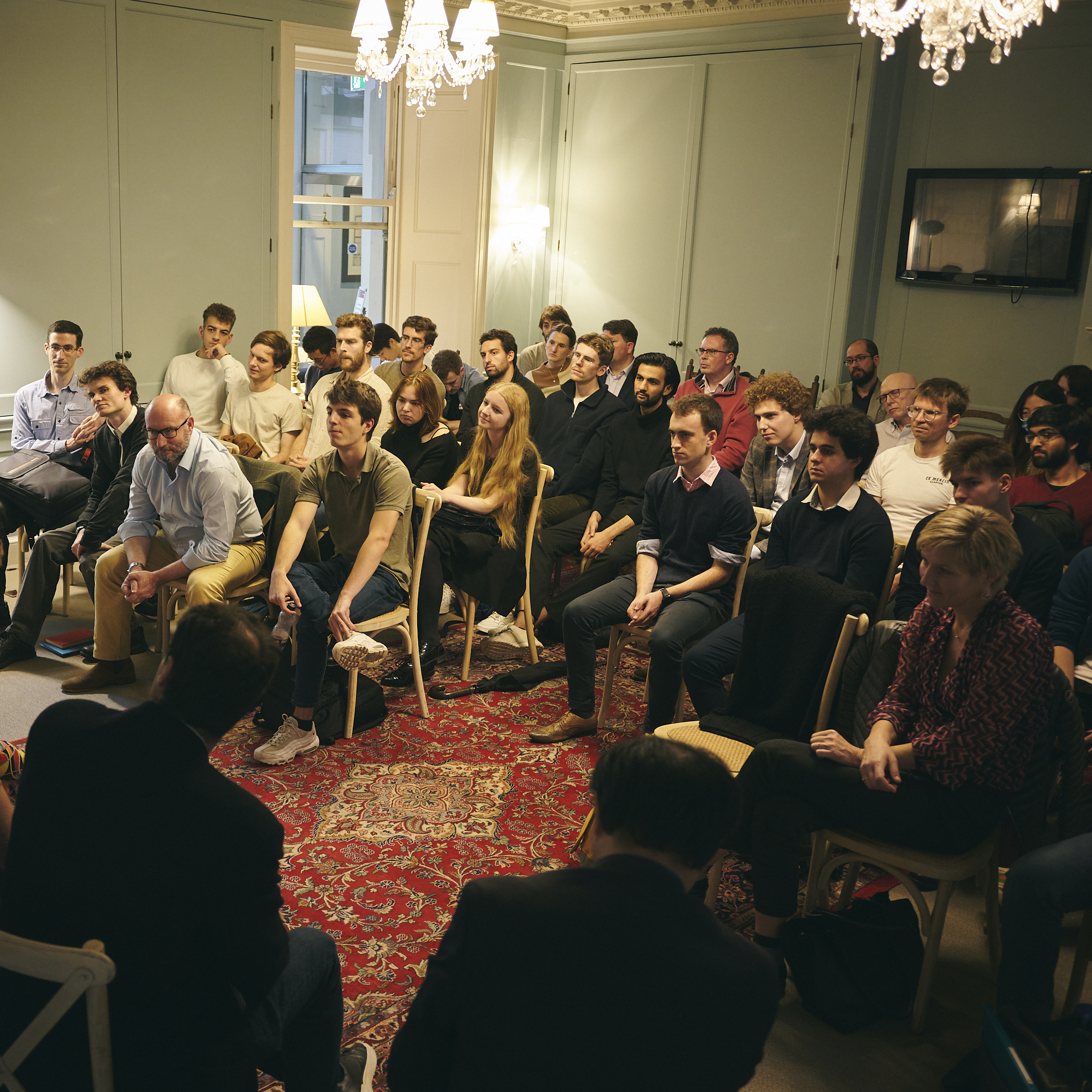
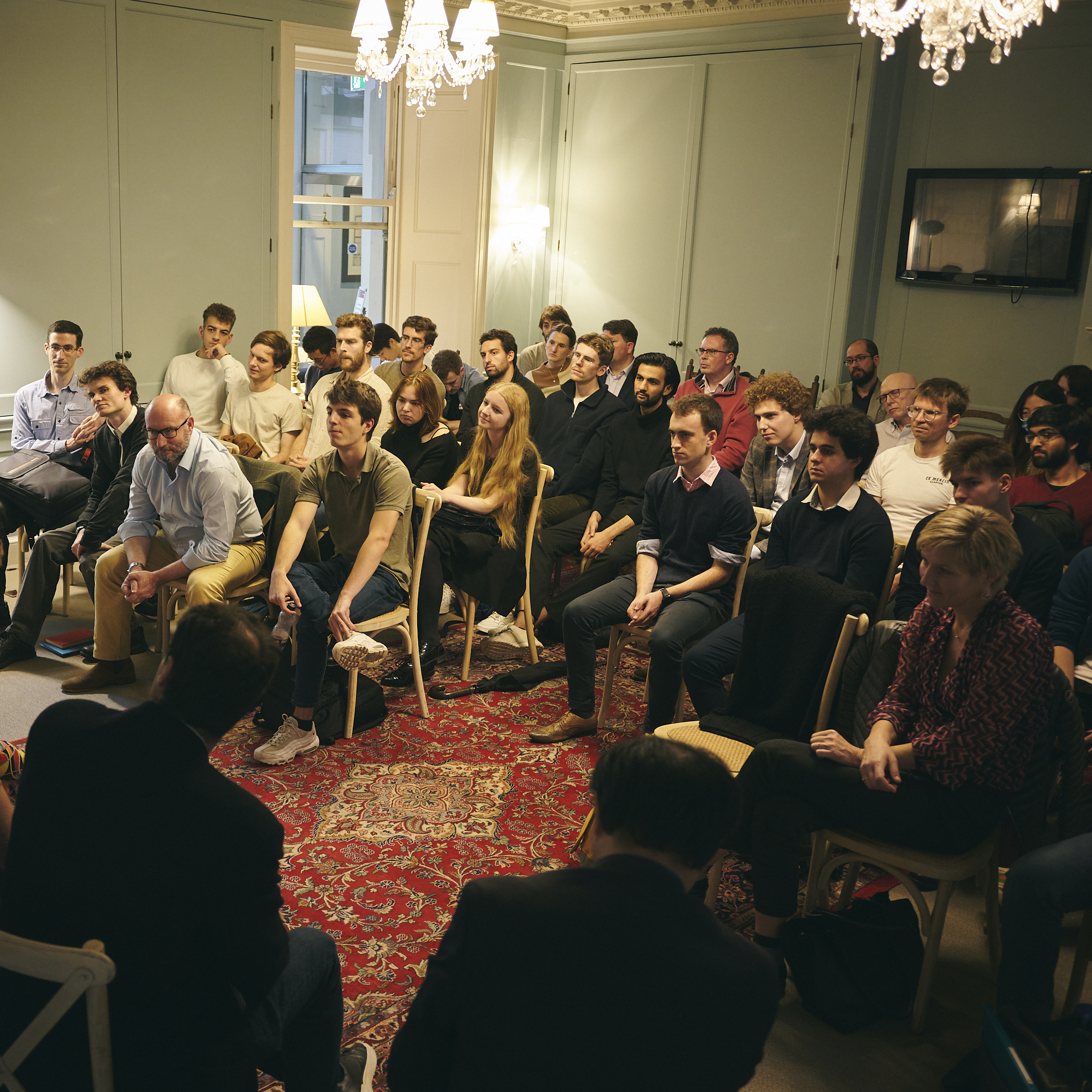
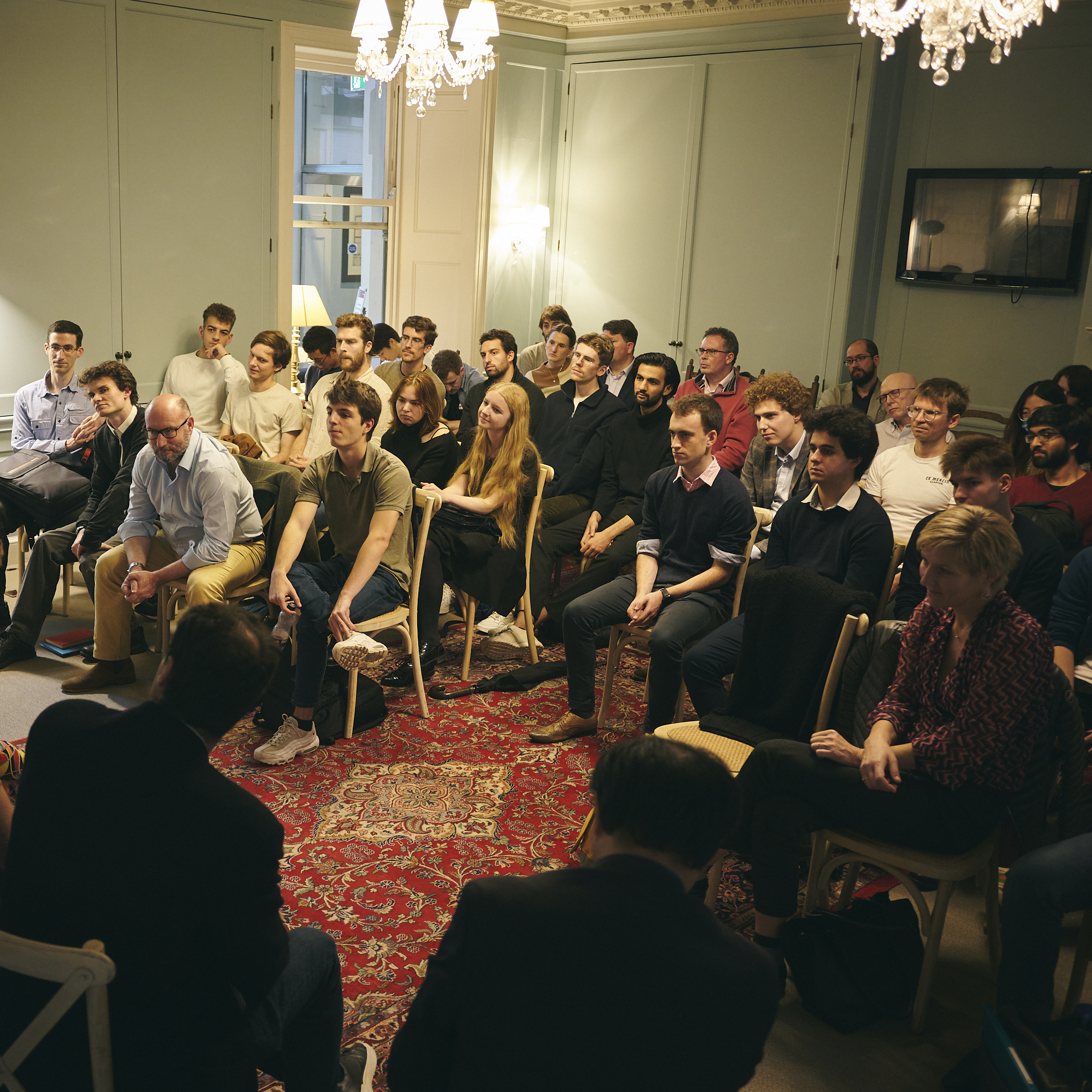
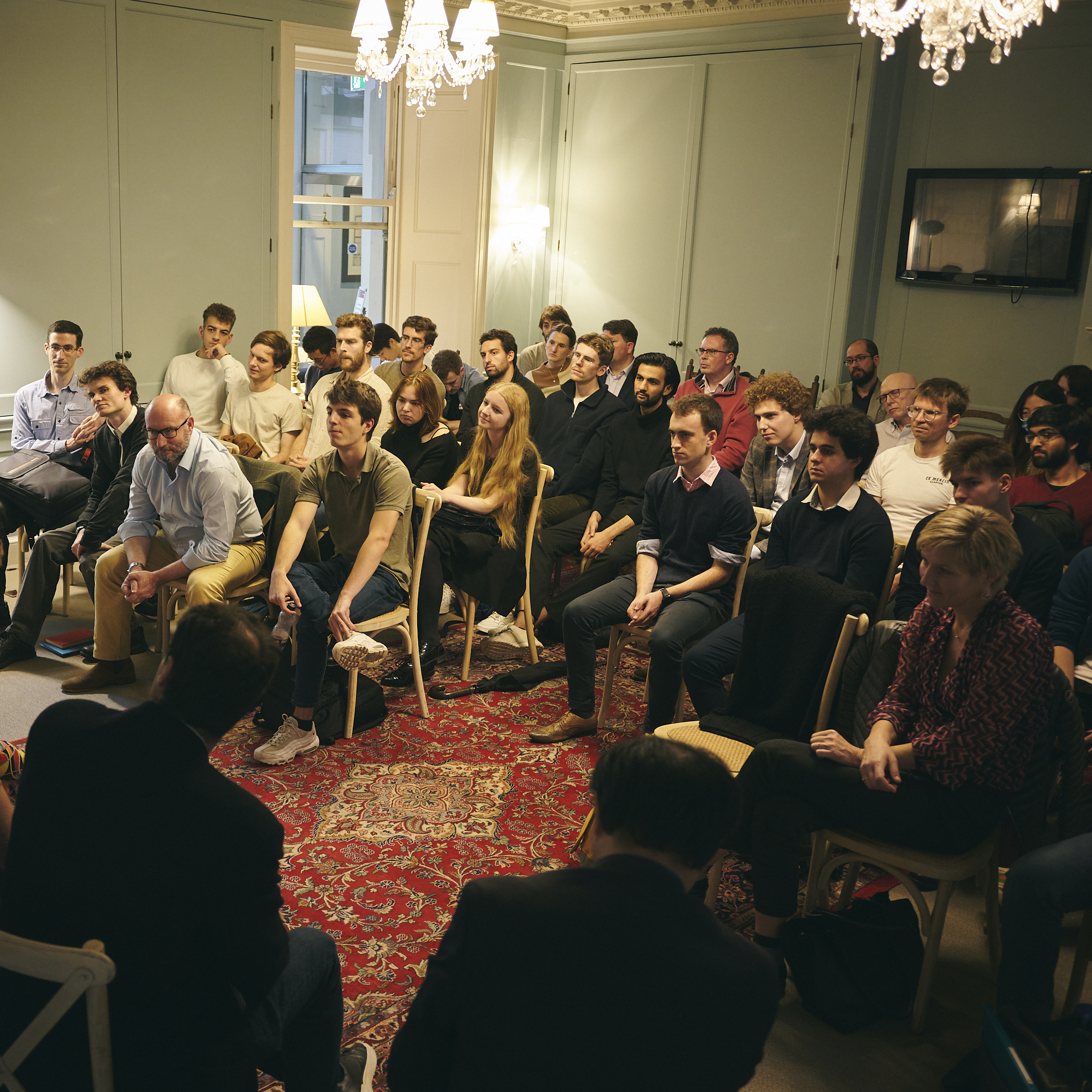
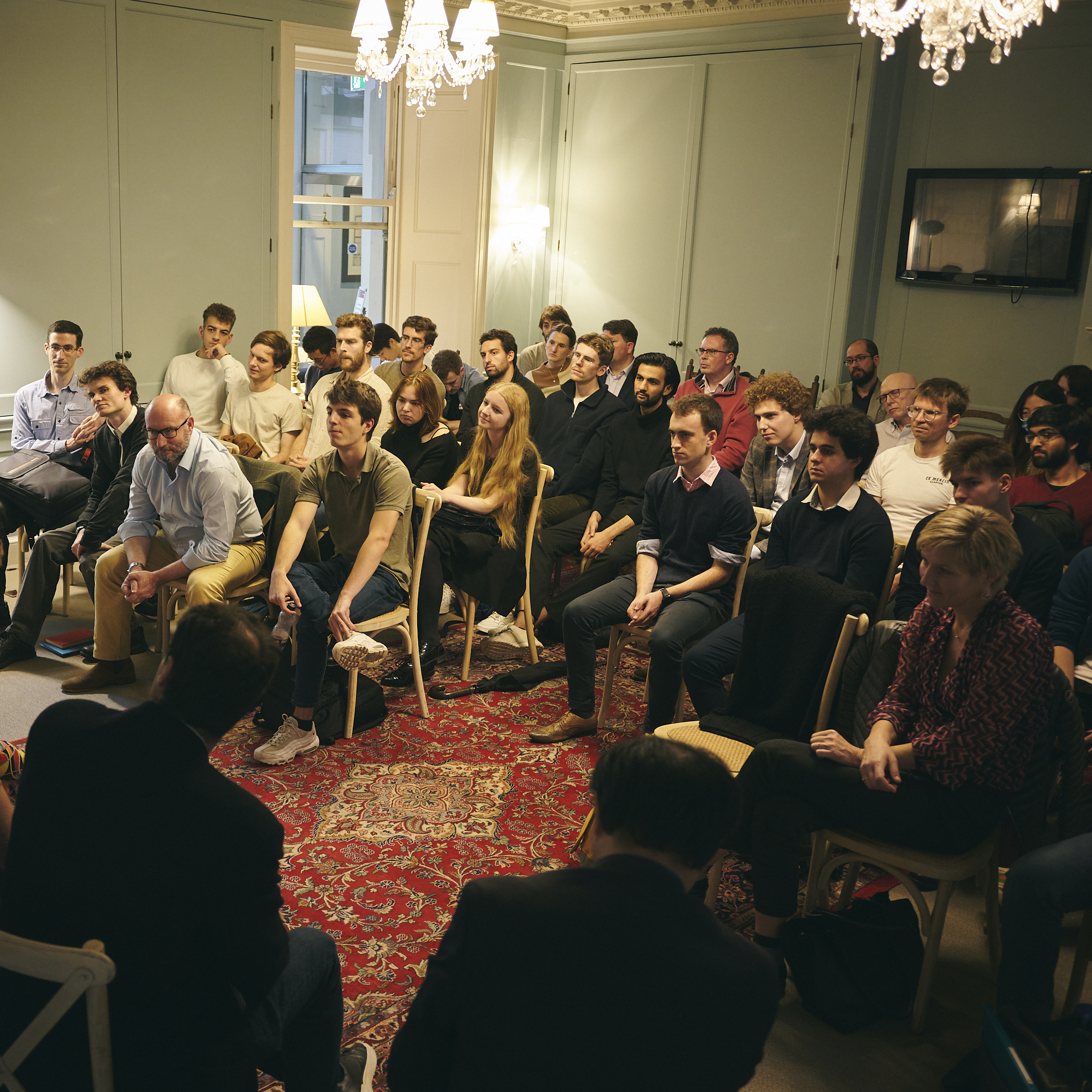
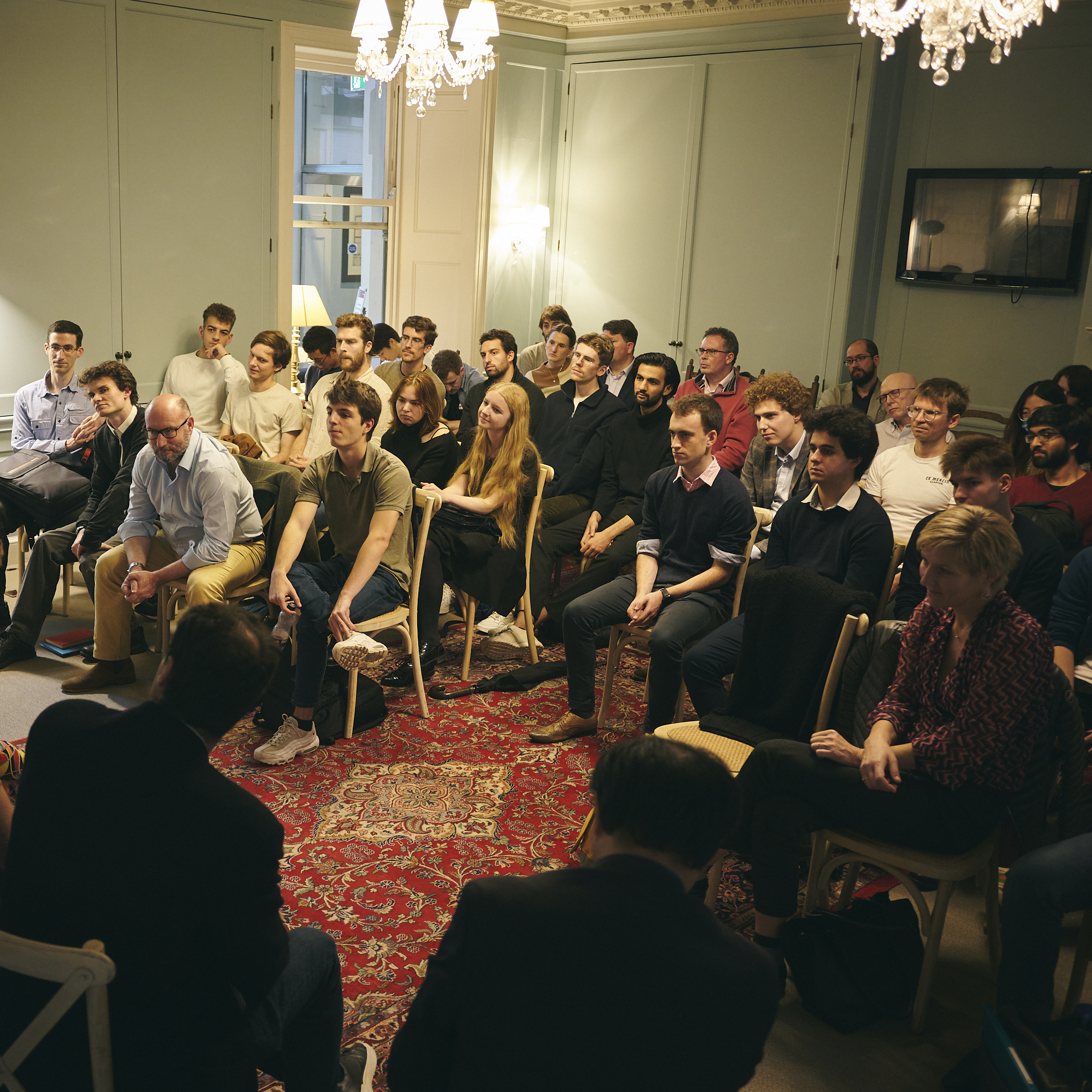
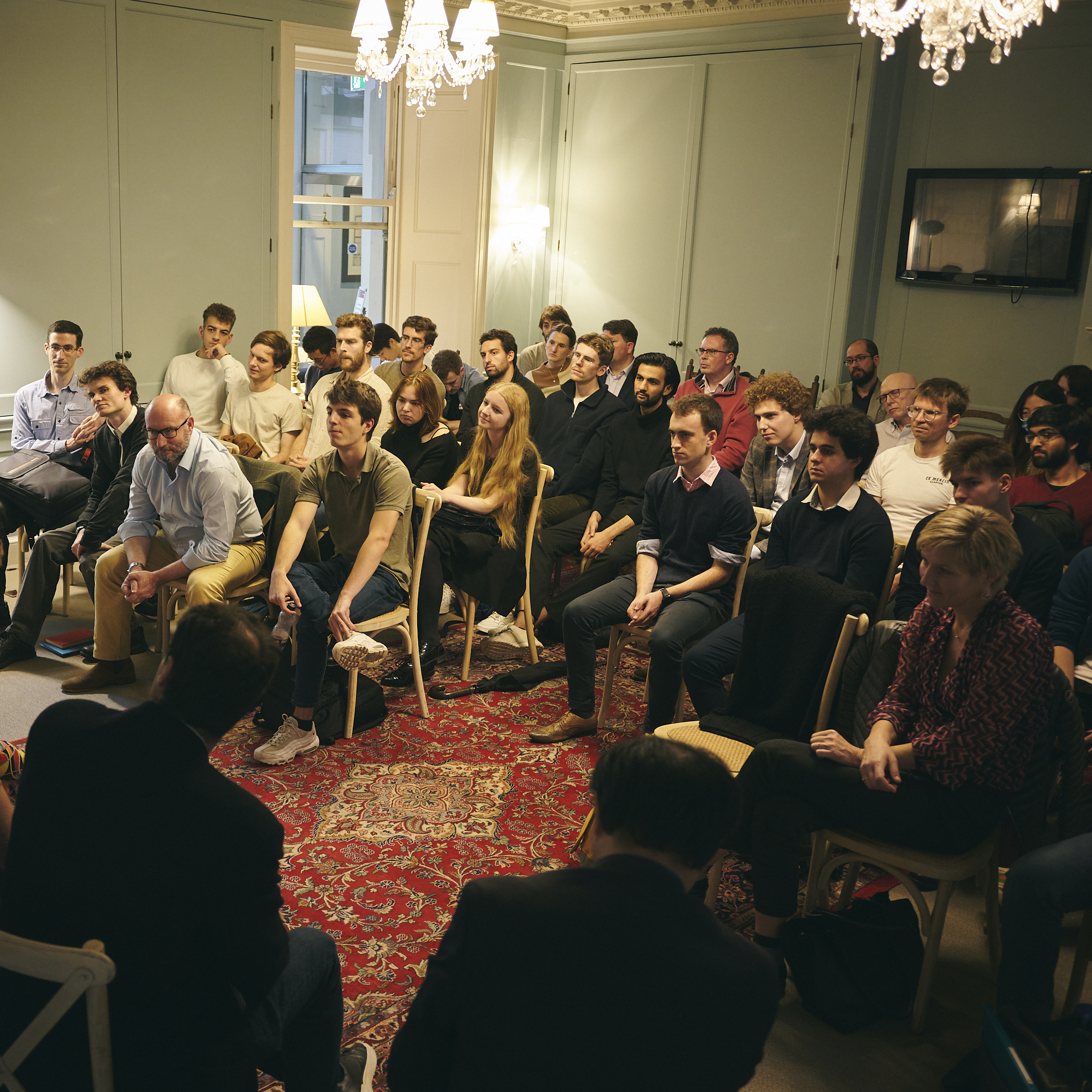
Speakers and panel members
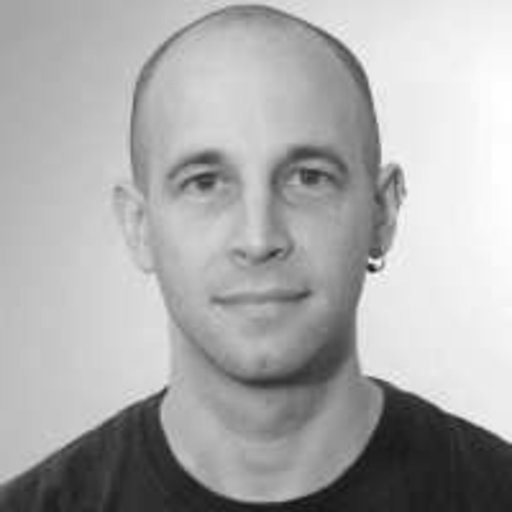
Kevin Buzzard is Professor of Pure Mathematics at ICL. He has taught at Harvard, Princeton and Berkeley. He holds the Whitehead and Berwick Prizes for his work in number theory. His research concerns the Langlands program and the role of AI in formal proof verification.
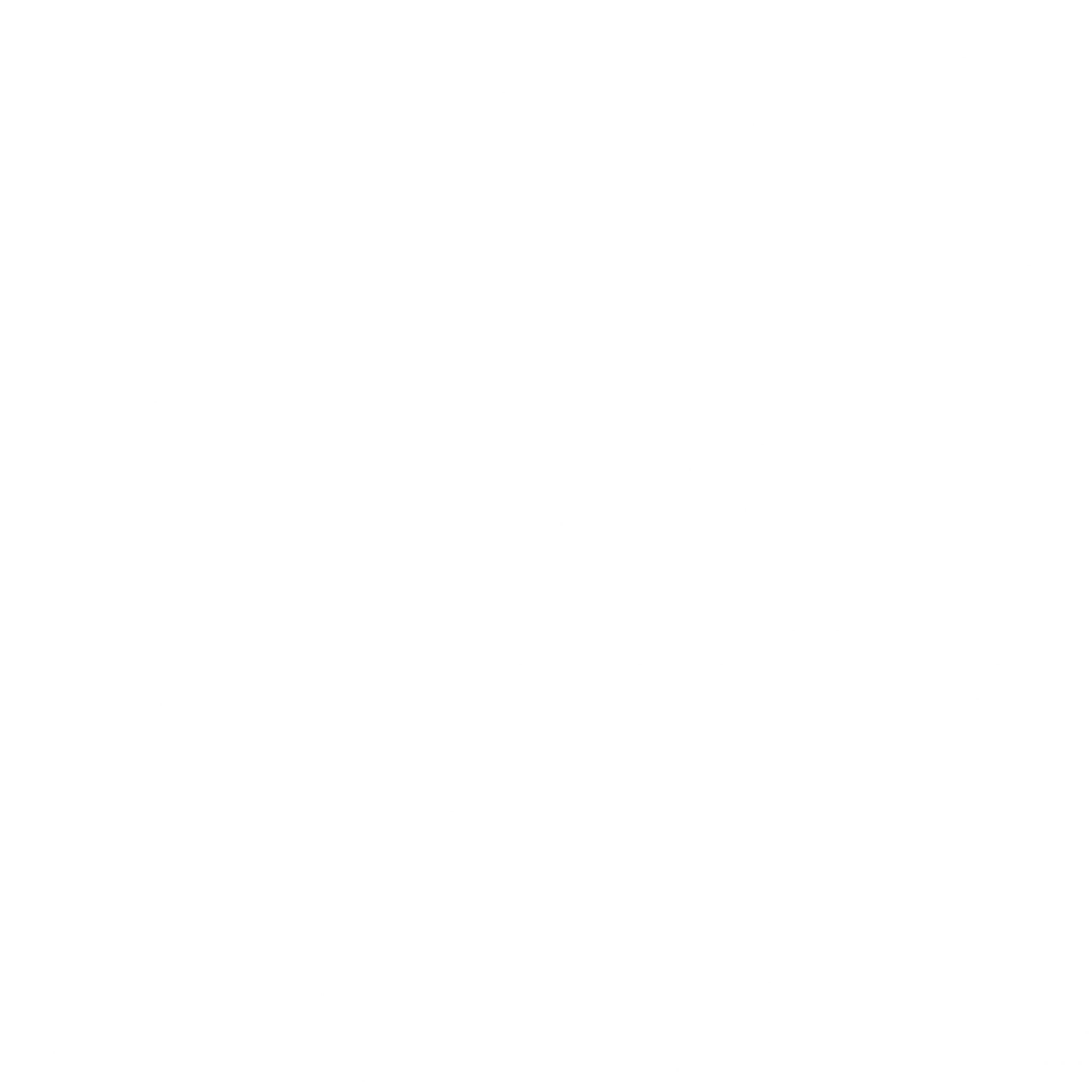
Teaching theorems to computers
I will discuss efforts made by mathematicians to teach proofs of complex theorems to computers. I will also explore why I believe this is an important and necessary thing to do, along with the benefits of such activities and potential future advancements.
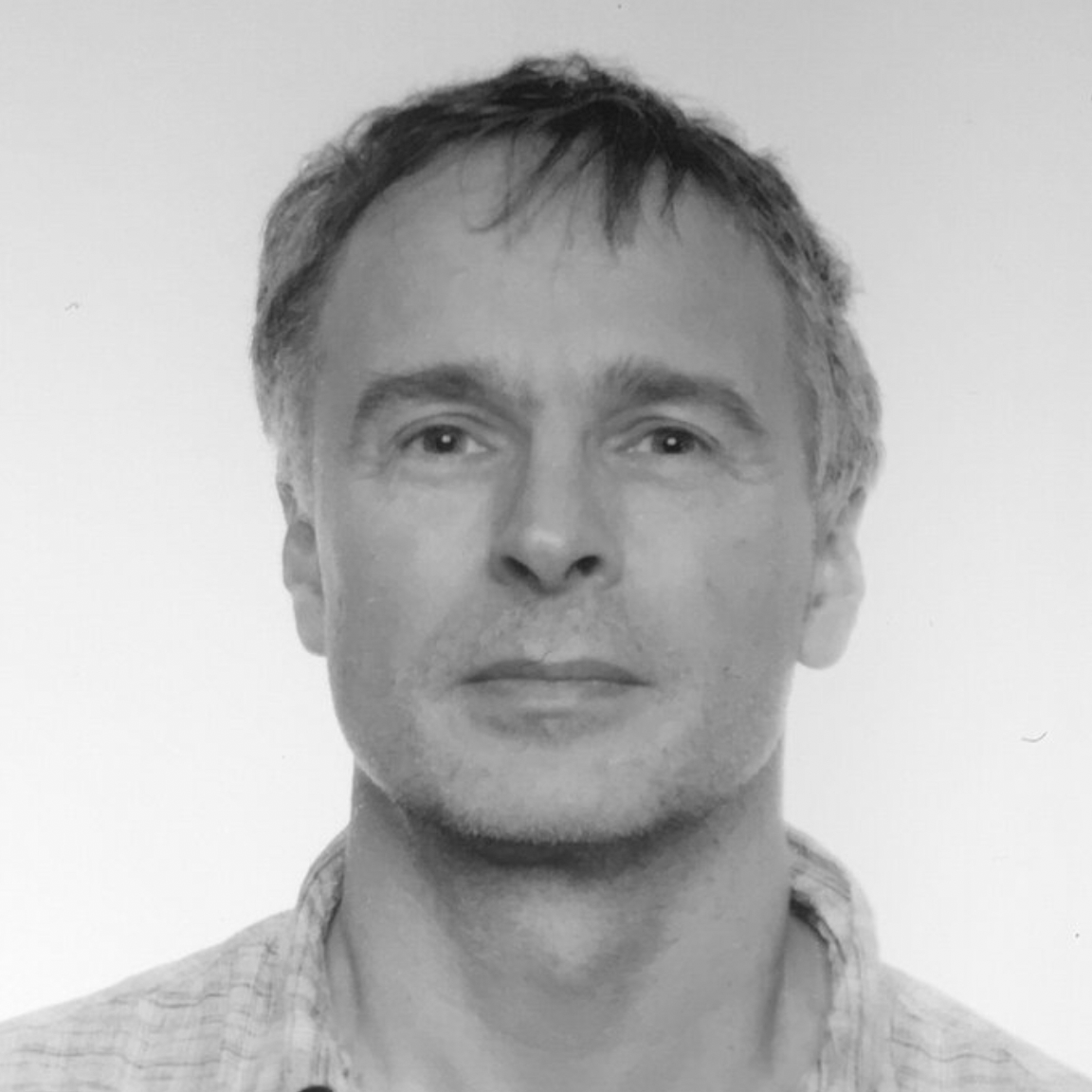
Peter Braam is a Professor at Oxford. He has taught at Utah, Carnegie Mellon and Cambridge. Peter created the Lustre parallel system and founded six start-ups, including Turbolinuz and Xyratex. He introduced SEI to the SKA telescope project and works on data-intensive computing.
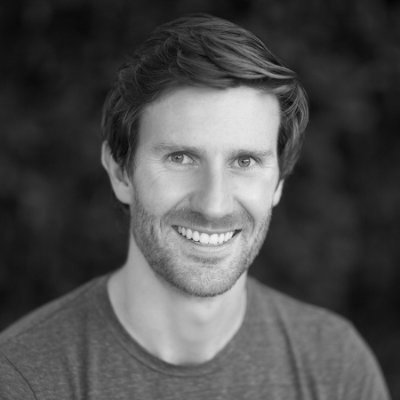
Dr Alex Davies is the founding lead of the AI for Maths initiative at DeepMind. He is a guest lecturer at Oxford and completed his PhD at Cambridge. He previously built machine learning systems at Google and currently works on the advancement of human intuition with AI.
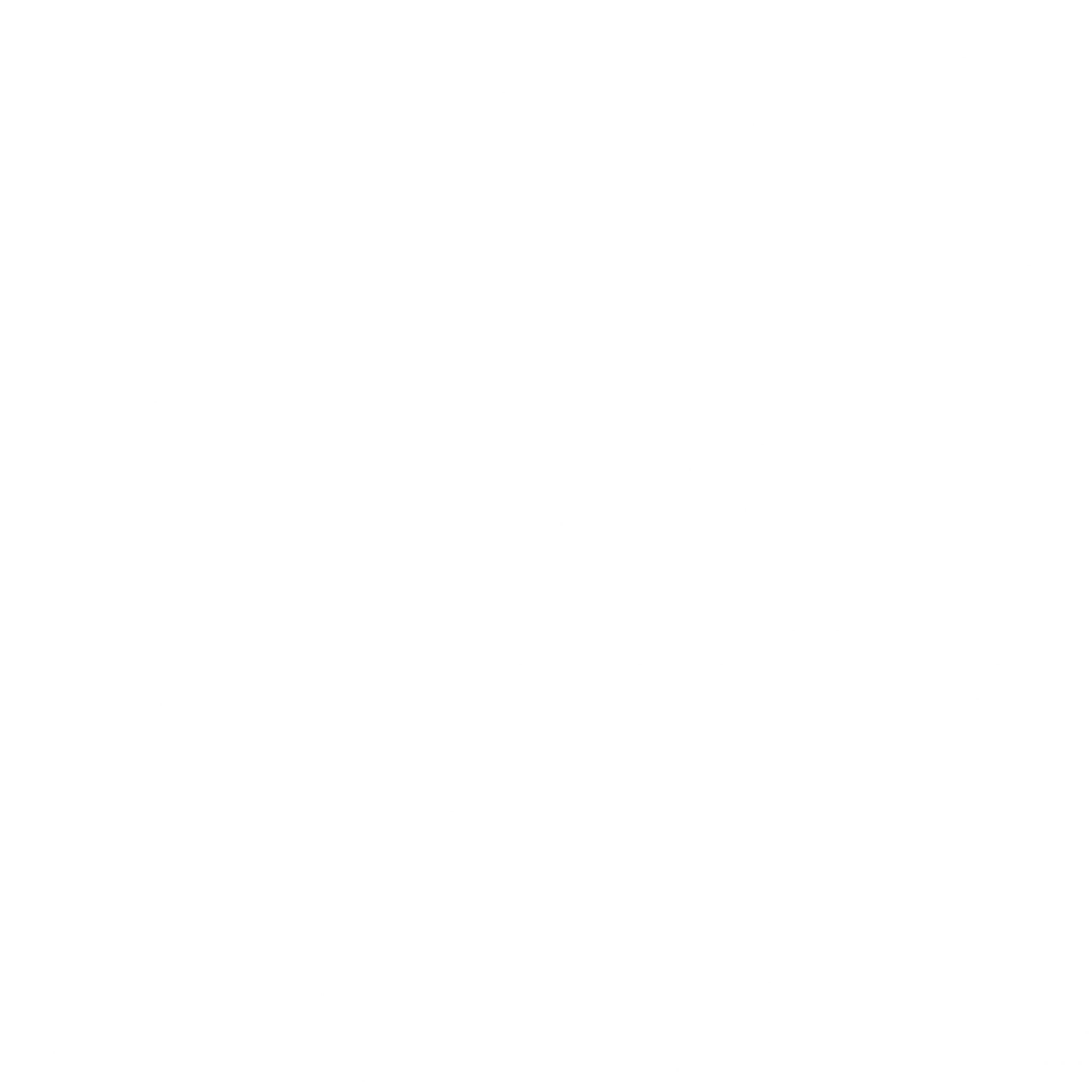
Machine learning with mathematicians
I use two examples to show how machine learning can be used to guide the development of beautiful new conjectures. The first is a connection between the hyperbolic and geometric structure of knots. The second is a resolution to a 50-year old problem in representation theory.
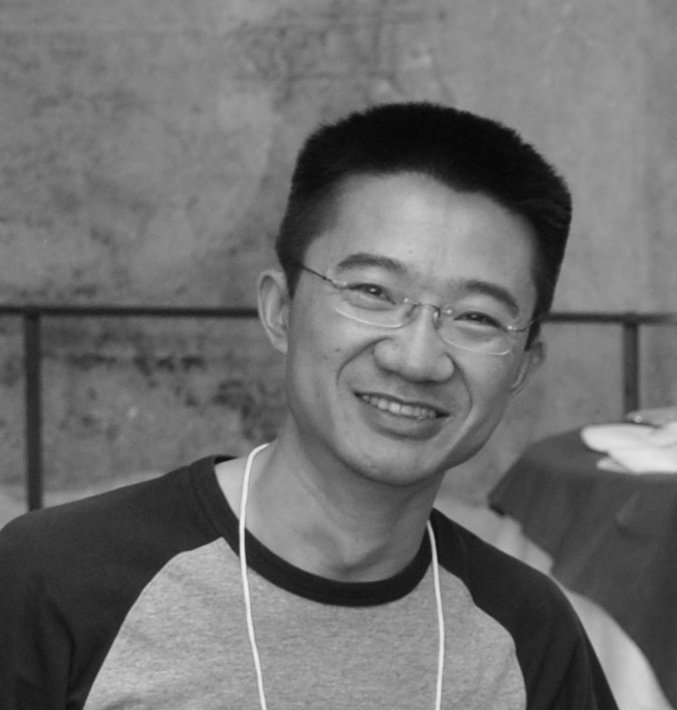
Dr Tiansi Dong is a senior research Fellow at the University of Bonn and a senior researcher at the Competence Center Machine Learning Rhine-Ruhr. His research concerns AI applications in language and vision, ML models, and neural geometric representation and reasoning.
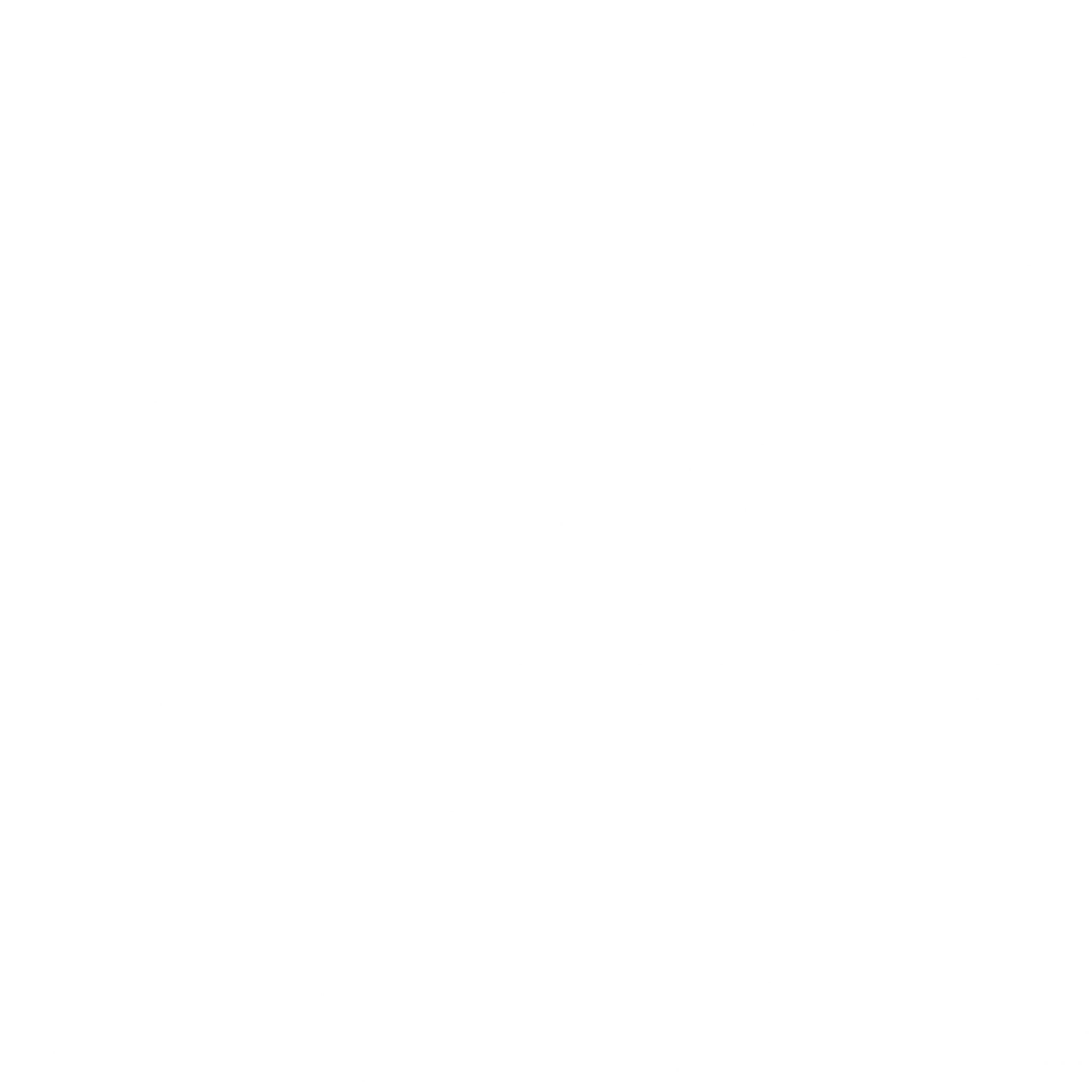
Syllogistic reasoning and beyond
We motivate and present a novel neural-network, called sphere neural network, which carries out deterministic, explainable, and rigorous syllogistic reasoning. Meaning in our model is embedded as a topological configuration of spheres, instead of relative locations among points.
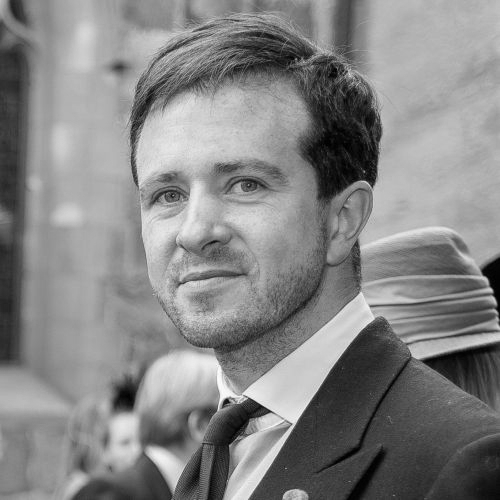
Dr Thomas Fink is the founding Director of the London Institute and Chargé de Recherche in the French CNRS. He studied physics at Caltech, Cambridge, and École Normale Supérieure. His work includes statistical physics, combinatorics, and the mathematics of evolvable systems.
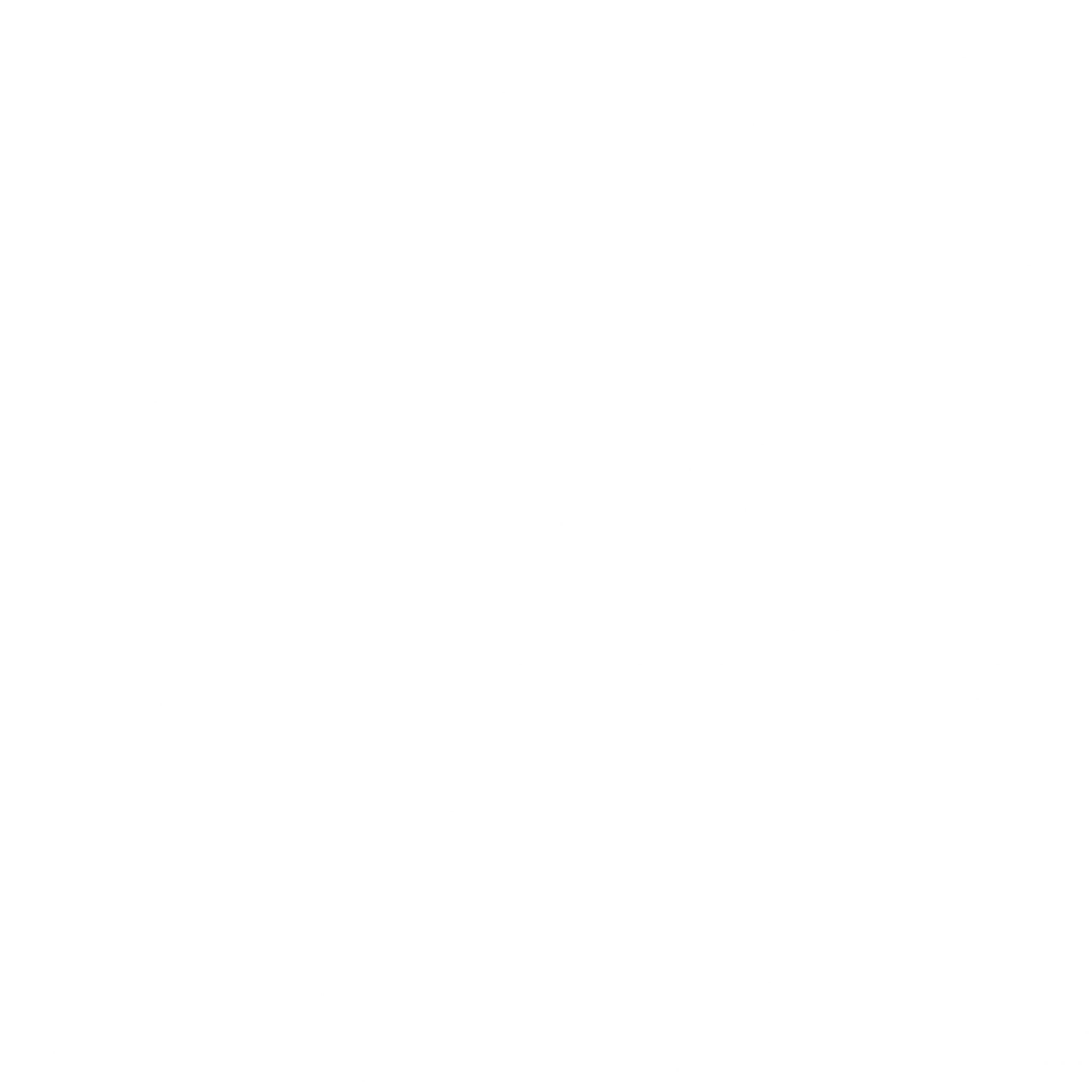
LIMS, AI and mathematics
When the London Institute wrote down the top 23 mathematical challenges to mark the launch of ARIA, automated conjectures was on the list. I’ll explain why, and share two mathematical constructs in number theory and graph theory, for which I hope AI can help form new conjectures.
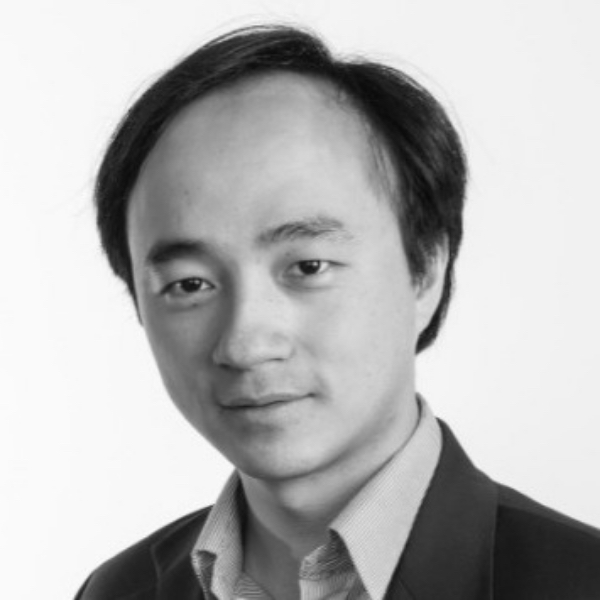
Prof. Yang-Hui He is a Fellow at the London Institute, Professor at City University, Chang-Jiang Chair at NanKai University and Lecturer at Merton College, Oxford. He studied at Princeton, Cambridge and MIT and researches string theory, algebraic geometry and machine learning.
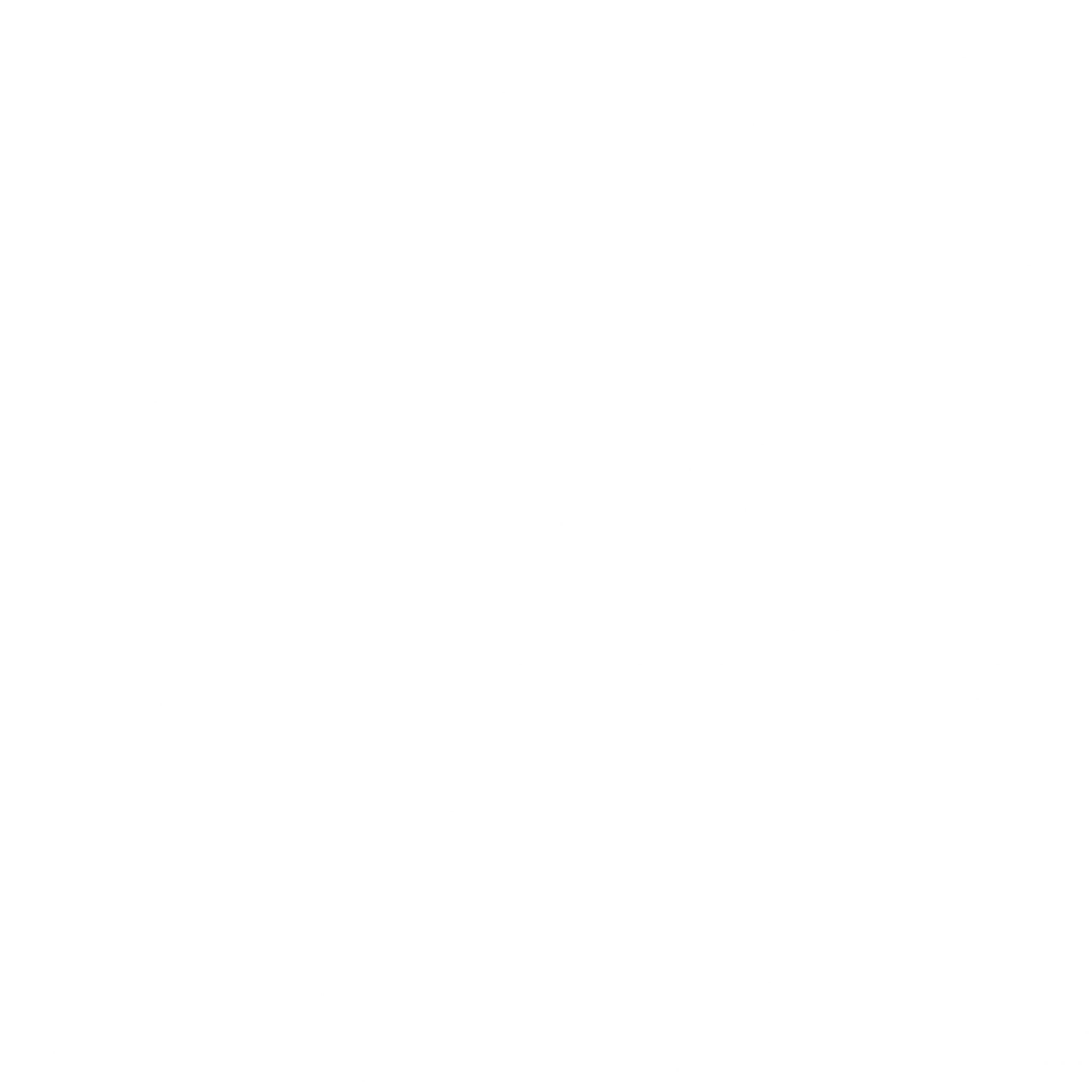
Machine-Learning Mathematical Structures
We present a number of experiments on machine-learning algorithms detecting patterns across mathematical disciplines from geometry to number theory, speculating on an inherent hierarchy. At the heart of the programme is the question how does AI help with mathematical discovery.
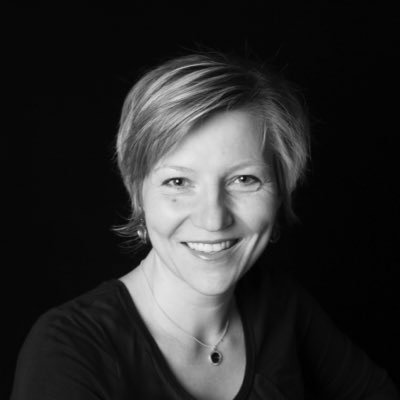
Prof. Mateja Jamnik is an Associate Fellow at the Leverhulme Centre and Professor of AI at Cambridge. Prof. Jamnik was awarded the Royal Society’s Athena Prize and served as an adviser to the House of Lords. Her research is in automated reasoning and human-computer interaction.
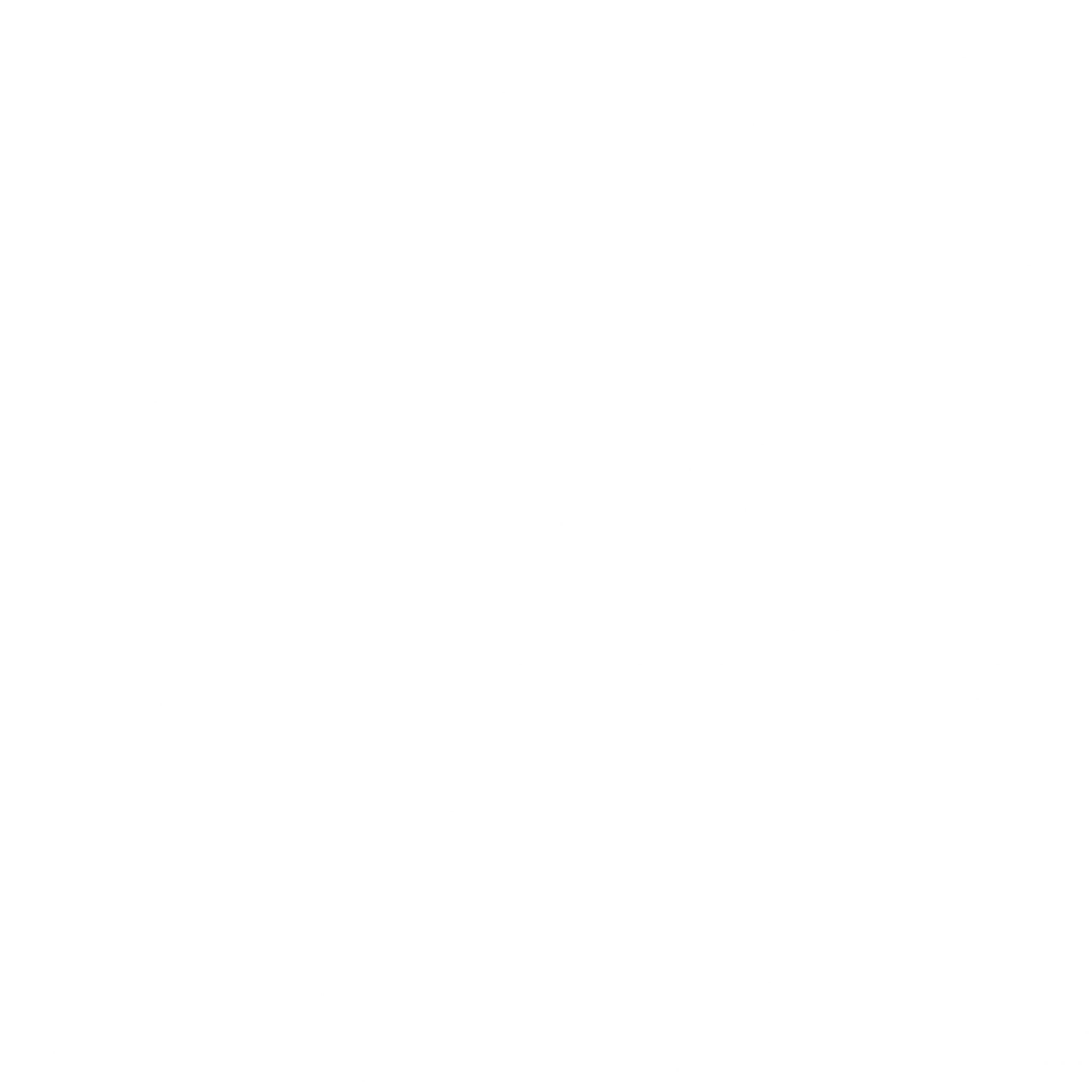
Towards a human-like reasoning system
We developed foundations for the analysis of representations used in reasoning. We built novel methods to computationally characterise and select representations appropriate for a given problem, and how a user reasons about it. This paves the way for personalising AI systems.
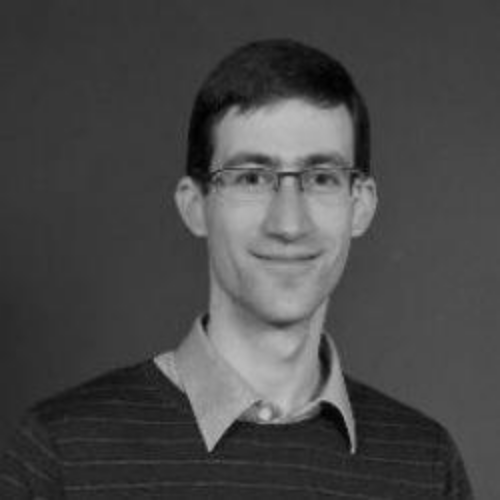
Prof. Andras Juhasz is a Fellow at Keble College, Oxford, and a Royal Society Research Fellow. He is the winner of the Pro Scientia Gold Medal and the Géza Grünwald prize. His work concerns advancing mathematics by guiding human intuition with AI and geometric topology.
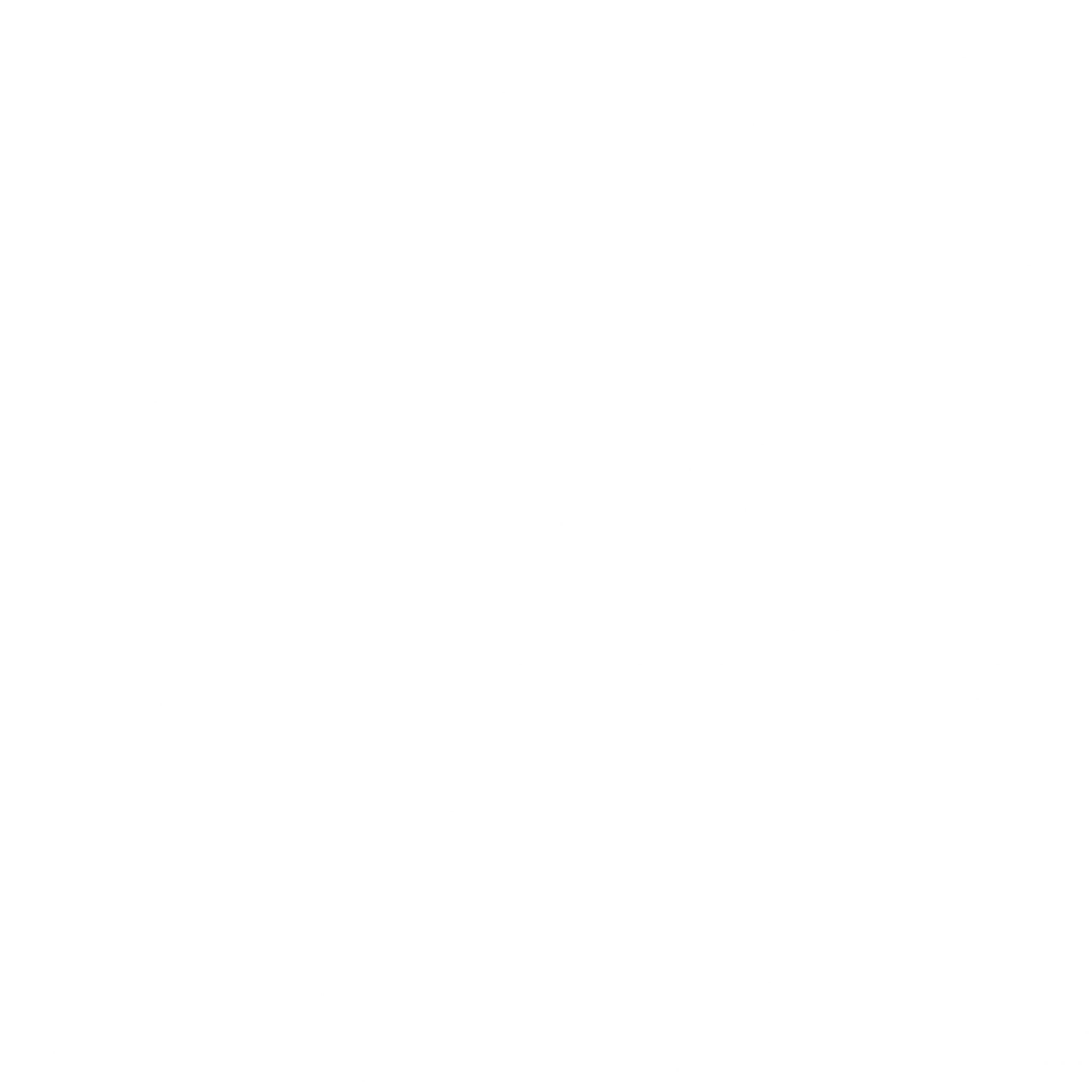
Knot theory and machine learning
The signature of a knot is a topological invariant in knot theory. A knot is hyperbolic if it is a hyperbolic link with one component. I will explain how we used machine learning methods to find an unexpected relationship between the signature and cusp shape of a hyperbolic knot.
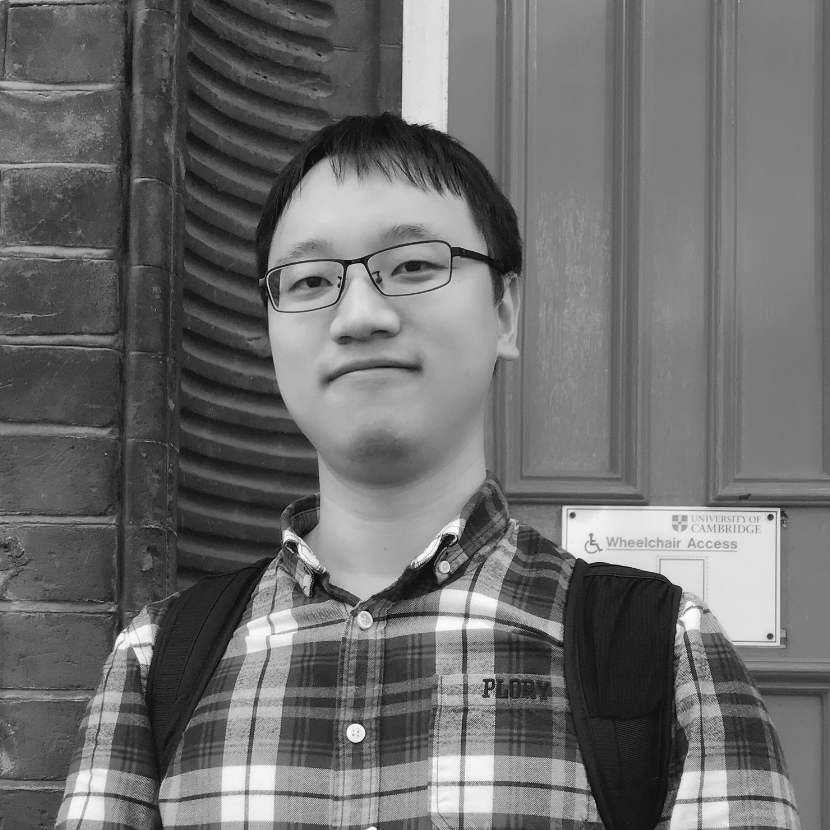
Dr Wenda Li is a Postdoctoral Research Associate in programming, semantics and logic at Cambridge. His research focuses on machine learning techniques, including verified symbolic computing, mechanised mathematics, and using language models to aid reasoning with theorem provers.
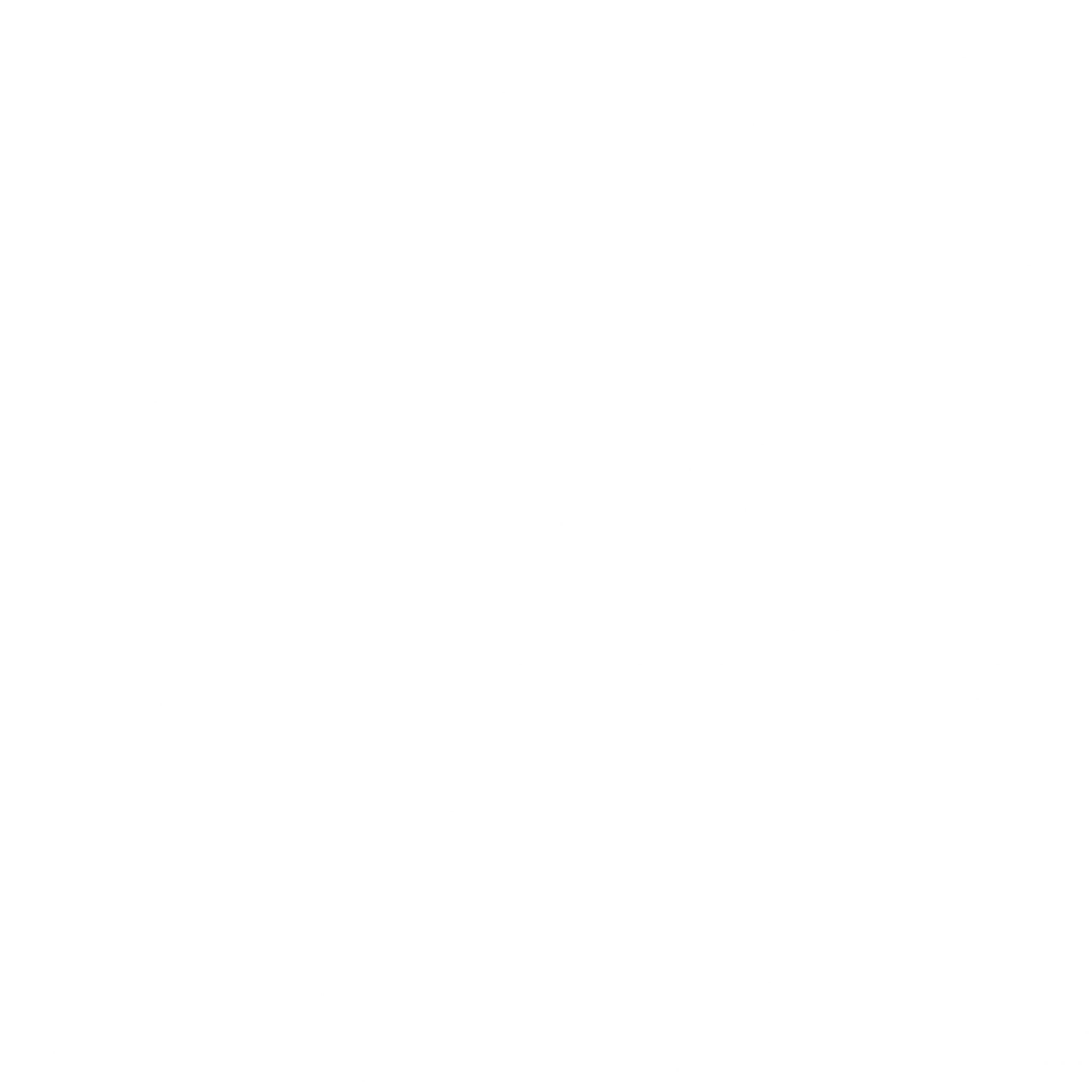
Draft, sketch and prove
To counter the difficulty of formalising mathematical proofs, we introduce a method that leverages informal proofs using large language models. The method maps informal proofs to formal sketches, and uses the sketches to guide an automated prover to navigate easier sub-problems.
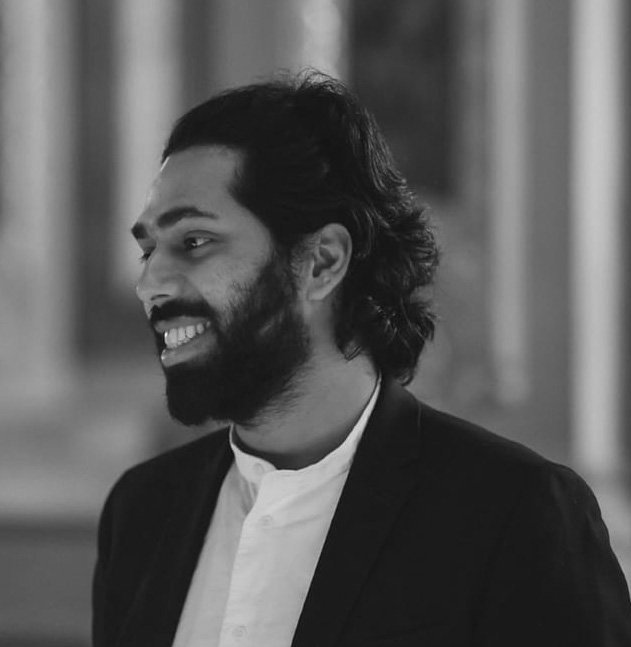
Dr Challenger Mishra is a Research Fellow at Cambridge and a Fellow of Queens’ College. His research includes machine learning, Calabi-Yau manifolds and string compactifications. He was previously a Rhodes Scholar at the Rudolf Peierls Centre for Theoretical Physics in Oxford.
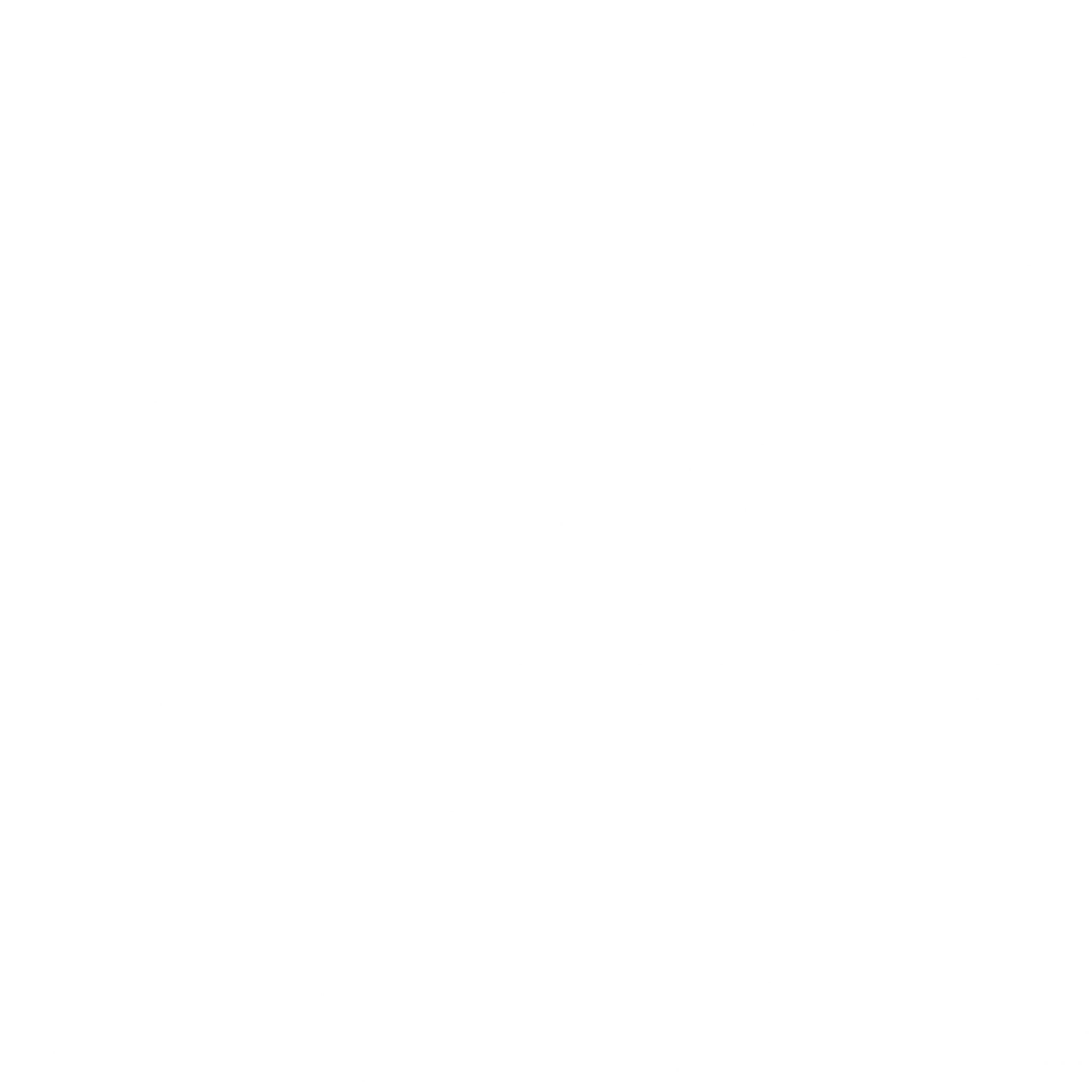
Deep Learning and pure mathematics
I will introduce different approaches to mathematical discovery using machine intelligence. Forging strong interdisciplinary collaborations between domain experts plays a pivotal role in accelerating the pace of discovery. I will use two examples to demonstrate connections between modern Deep Learning and pure mathematics.
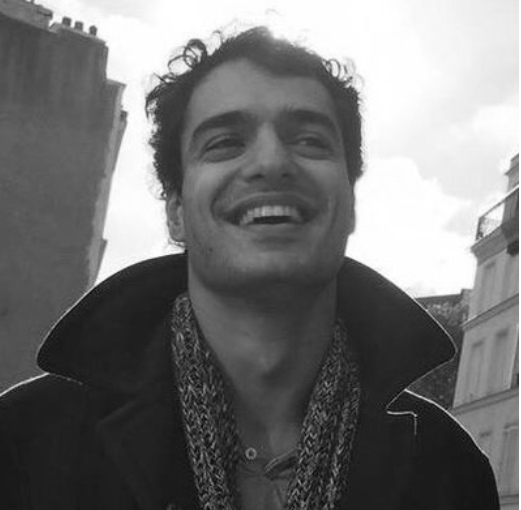
Dr Theo Zenou is a journalist and historian. He has written for The Washington Post, FT, The Times, Telegraph, Spectator, among others, and has interviewed Oliver Stone, Werner Herzog and Neal Stephenson. He did a PhD in history at Cambridge, focusing on the Cold War and nuclear weapons.
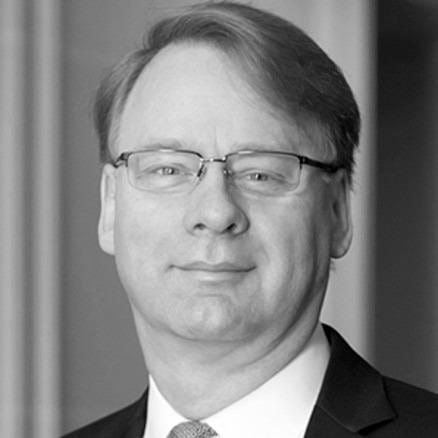
Martin Reeves is a Senior Partner and Managing Director at BCG and is the chairman of the BCG Henderson Institute. He studied at Cambridge, the Cranfield Institute, Tokyo and Osaka. Martin leads research on science and technology and business and society. He is also a published author.